Neural Gaussian Mirror for Controlled Feature Selection in Neural Networks
arxiv(2020)
摘要
Deep neural networks (DNNs) have become increasingly popular and achieved outstanding performance in predictive tasks. However, the DNN framework itself cannot inform the user which features are more or less relevant for making the prediction, which limits its applicability in many scientific fields. We introduce neural Gaussian mirrors (NGMs), in which mirrored features are created, via a structured perturbation based on a kernel-based conditional dependence measure, to help evaluate feature importance. We design two modifications of the DNN architecture for incorporating mirrored features and providing mirror statistics to measure feature importance. As shown in simulated and real data examples, the proposed method controls the feature selection error rate at a predefined level and maintains a high selection power even with the presence of highly correlated features.
更多查看译文
关键词
Deep neural networks,false discovery rate control,kernel methods,interpretability
AI 理解论文
溯源树
样例
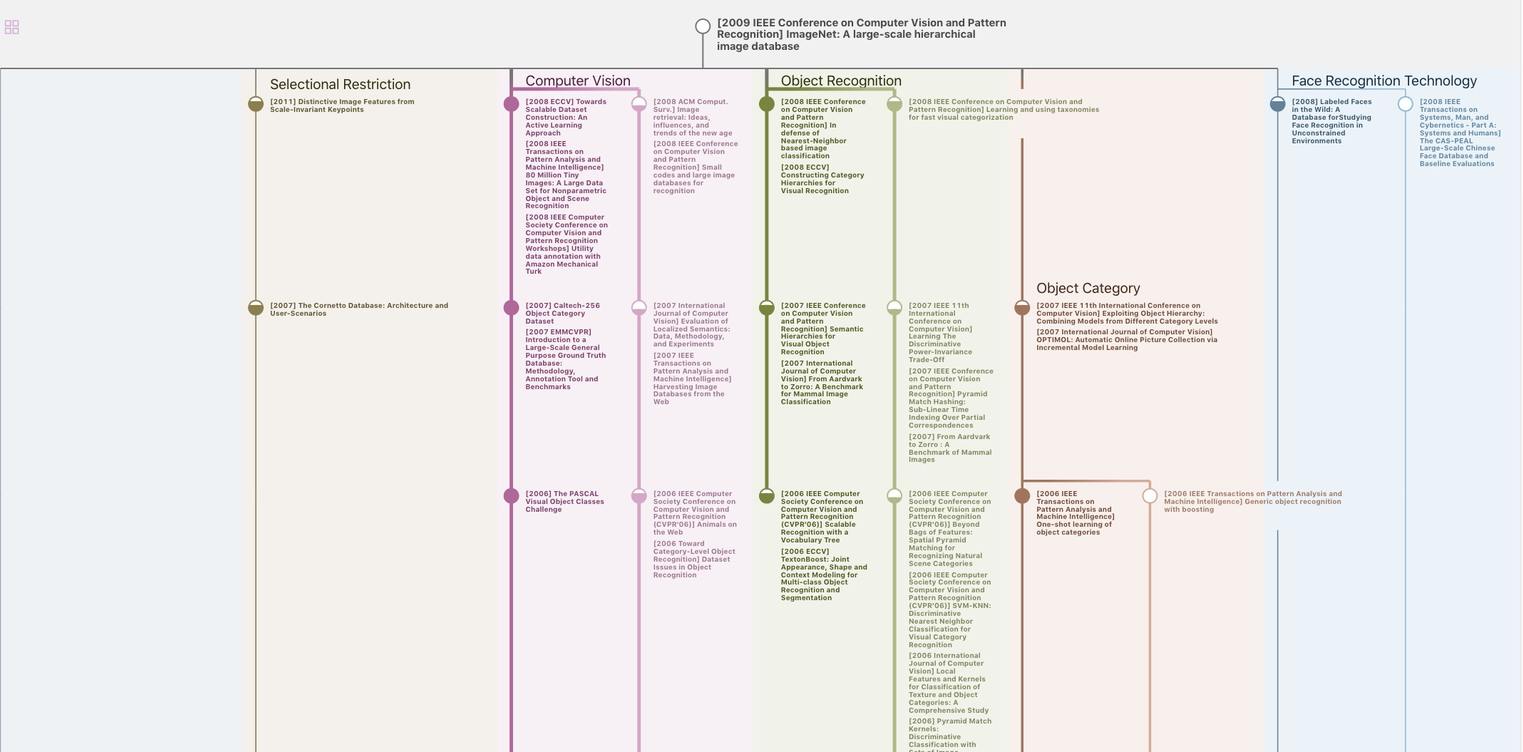
生成溯源树,研究论文发展脉络
Chat Paper
正在生成论文摘要