Learning Selective Mutual Attention and Contrast for RGB-D Saliency Detection
IEEE Transactions on Pattern Analysis and Machine Intelligence(2022)
摘要
How to effectively fuse cross-modal information is a key problem for RGB-D salient object detection. Early fusion and result fusion schemes fuse RGB and depth information at the input and output stages, respectively, and hence incur distribution gaps or information loss. Many models instead employ a feature fusion strategy, but they are limited by their use of low-order point-to-point fusion methods. In this paper, we propose a novel mutual attention model by fusing attention and context from different modalities. We use the non-local attention of one modality to propagate long-range contextual dependencies for the other, thus leveraging complementary attention cues to achieve high-order and trilinear cross-modal interaction. We also propose to induce contrast inference from the mutual attention and obtain a unified model. Considering that low-quality depth data may be detrimental to model performance, we further propose a selective attention to reweight the added depth cues. We embed the proposed modules in a two-stream CNN for RGB-D SOD. Experimental results demonstrate the effectiveness of our proposed model. Moreover, we also construct a new and challenging large-scale RGB-D SOD dataset of high-quality, which can promote both the training and evaluation of deep models.
更多查看译文
关键词
Image Processing, Computer-Assisted,Algorithms,Learning
AI 理解论文
溯源树
样例
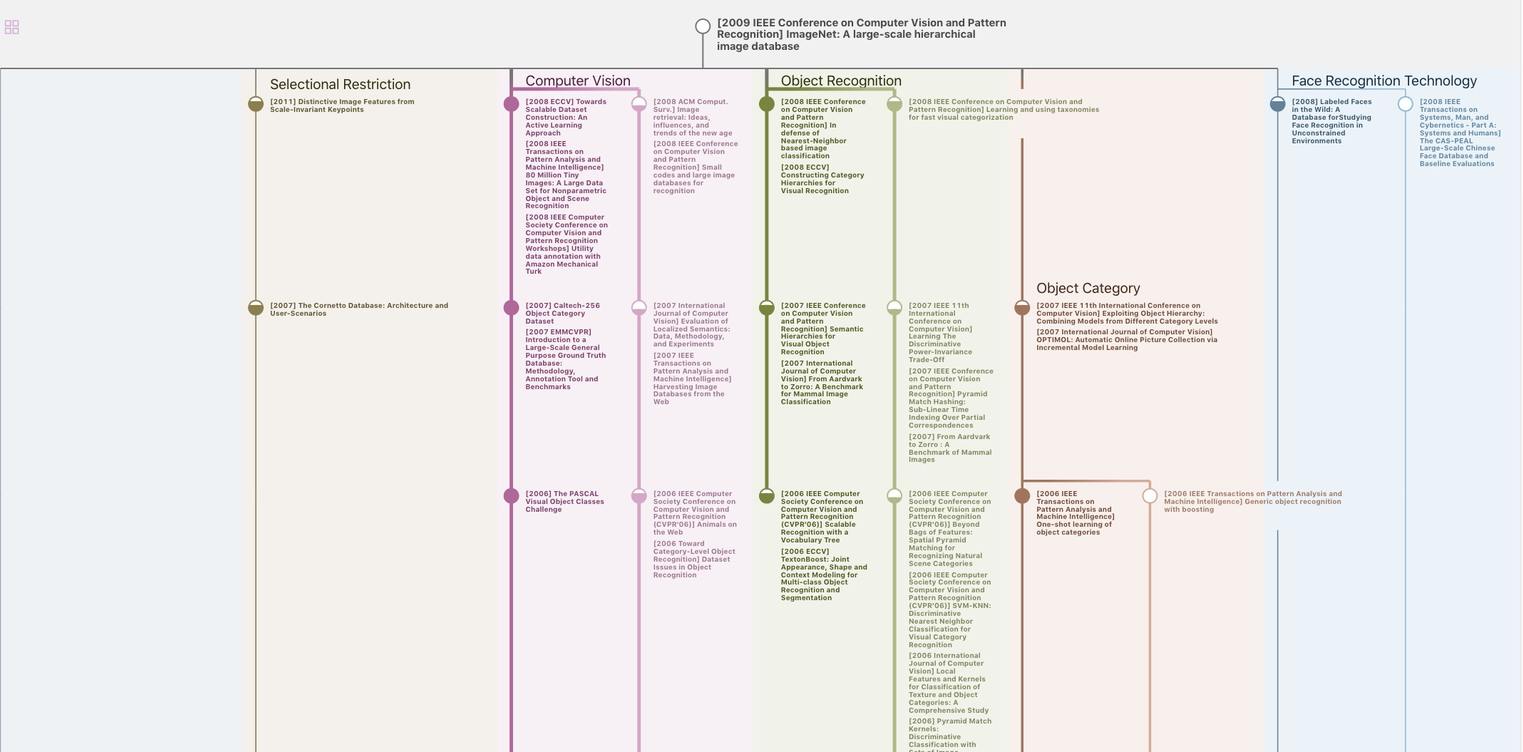
生成溯源树,研究论文发展脉络
Chat Paper
正在生成论文摘要