Uncertainty modelling in deep learning for safer neuroimage enhancement: Demonstration in diffusion MRI
NeuroImage(2021)
摘要
•Proposes methods for modelling different types of uncertainty that arise in deep learning (DL) applications for image enhancement problems.•Demonstrates in dMRI super-resolution tasks that modelling uncertainty enhances the safety of DL-based enhancement system by bringing two categories of practical benefits:(1) “performance improvement”: e.g., the generalisation to out-of-distribution data, robustness to noise and outliers (Section 4.3)(2) “reliability assessment of prediction”: e.g., certification of performance based on uncertainty-thresholding (Section 4.4.1); detection of unfamiliar structures and understanding the sources of uncertainty (Section 4.4.2).•Provide a comprehensive set of experiments in a diverse set of datasets, which vary in demographics, scanner types, acquisition protocols or pathology.•The methods are in theory applicable to many other imaging modalities and data enhancement applications.•Codes will be available on Github.
更多查看译文
关键词
Uncertainty quantification,Deep learning,Safety,Robustness,Interpretability,Super-resolution,Image enhancement,Image synthesis,Neuroimaging,Diffusion MRI,Tractography
AI 理解论文
溯源树
样例
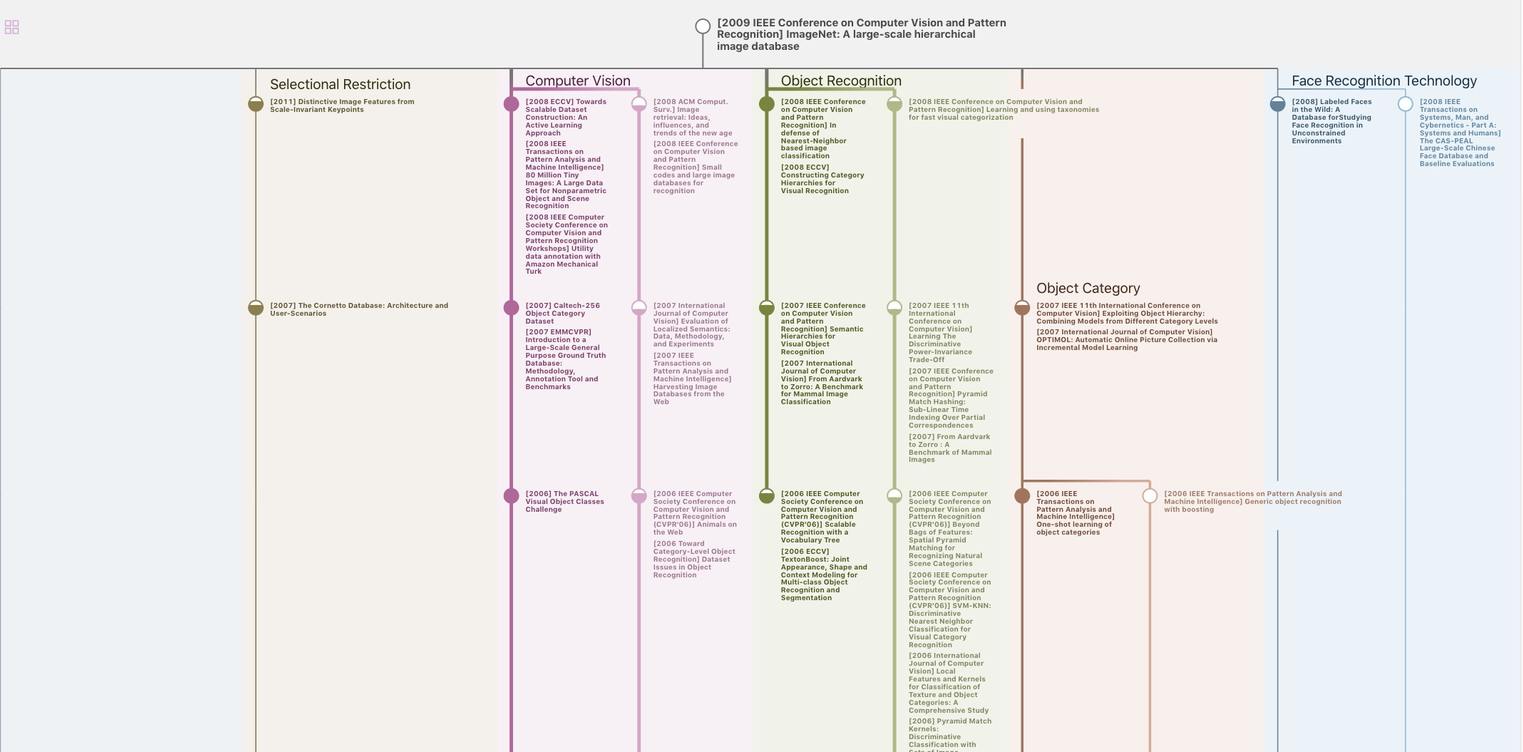
生成溯源树,研究论文发展脉络
Chat Paper
正在生成论文摘要