When Bitstream Prior Meets Deep Prior: Compressed Video Super-resolution with Learning from Decoding
MM '20: The 28th ACM International Conference on Multimedia Seattle WA USA October, 2020(2020)
摘要
The standard paradigm of video super-resolution (SR) is to generate the spatial-temporal coherent high-resolution (HR) sequence from the corresponding low-resolution (LR) version which has already been decoded from the bitstream. However, a highly practical while relatively under-studied way is enabling the built-in SR functionality in the decoder, in the sense that almost all videos are compactly represented. In this paper, we systematically investigate the SR of compressed LR videos by leveraging the interactivity between decoding prior and deep prior. By fully exploiting the compact video stream information, the proposed bitstream prior embedded SR framework achieves compressed video SR and quality enhancement simultaneously in a single feed-forward process. More specifically, we propose a motion vector guided multi-scale local attention module that explicitly exploits the temporal dependency and suppresses coding artifacts with substantially economized computational complexity. Moreover, a scale-wise deep residual-in-residual network is learned to reconstruct the SR frames from the multi-scale fused features. To facilitate the research of compressed video SR, we also build a large-scale dataset with compressed videos of diverse content, including ready-made diversified kinds of side information extracted from the bitstream. Both quantitative and qualitative evaluations show that our model achieves superior performance for compressed video SR, and offers competitive performance compared to the sequential combinations of the state-of-the-art methods for compressed video artifacts removal and SR.
更多查看译文
关键词
Compressed video, video super-resolution, deep learning, coding bitstream prior
AI 理解论文
溯源树
样例
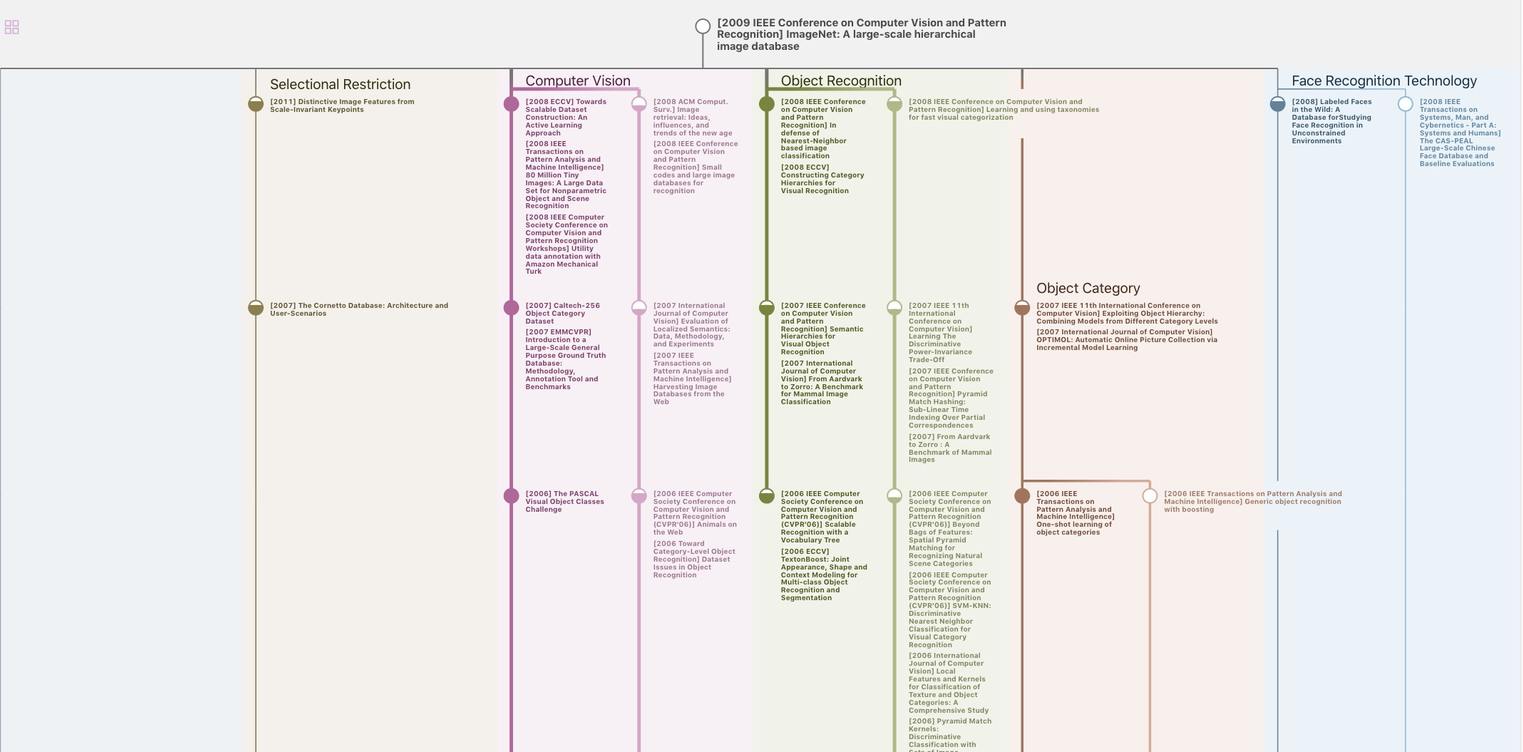
生成溯源树,研究论文发展脉络
Chat Paper
正在生成论文摘要