Relational Graph Learning for Grounded Video Description Generation
MM '20: The 28th ACM International Conference on Multimedia Seattle WA USA October, 2020(2020)
摘要
Grounded video description (GVD) encourages captioning models to attend to appropriate video regions (e.g., objects) dynamically and generate a description. Such a setting can help explain the decisions of captioning models and prevents the model from hallucinating object words in its description. However, such design mainly focuses on object word generation and thus may ignore fine-grained information and suffer from missing visual concepts. Moreover, relational words (e.g., 'jump left or right') are usual spatio-temporal inference results, i.e., these words cannot be grounded on certain spatial regions. To tackle the above limitations, we design a novel relational graph learning framework for GVD, in which a language-refined scene graph representation is designed to explore fine-grained visual concepts. Furthermore, the refined graph can be regarded as relational inductive knowledge to assist captioning models in selecting the relevant information it needs to generate correct words. We validate the effectiveness of our model through automatic metrics and human evaluation, and the results indicate that our approach can generate more fine-grained and accurate description, and it solves the problem of object hallucination to some extent.
更多查看译文
关键词
grounded video description, language refined scene graph, object hallucination, fine-grained information
AI 理解论文
溯源树
样例
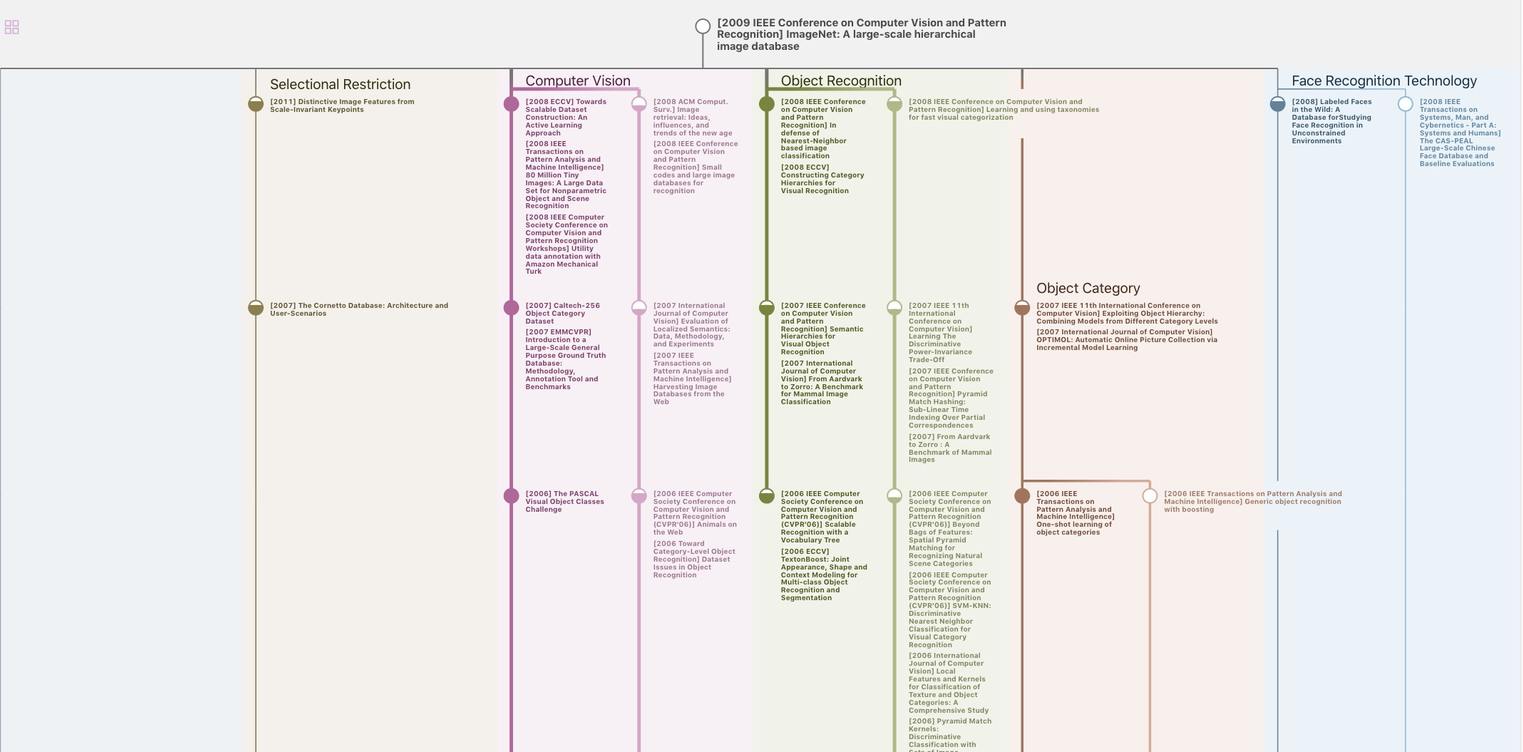
生成溯源树,研究论文发展脉络
Chat Paper
正在生成论文摘要