Approximating smooth functions by deep neural networks with sigmoid activation function
Journal of Multivariate Analysis(2021)
摘要
We study the power of deep neural networks (DNNs) with sigmoid activation function. Recently, it was shown that DNNs approximate any d-dimensional, smooth function on a compact set with a rate of order W−p∕d, where W is the number of nonzero weights in the network and p is the smoothness of the function. Unfortunately, these rates only hold for a special class of sparsely connected DNNs. We ask ourselves if we can show the same approximation rate for a simpler and more general class, i.e., DNNs which are only defined by its width and depth. In this article we show that DNNs with fixed depth and a width of order Md achieve an approximation rate of M−2p. As a conclusion we quantitatively characterize the approximation power of DNNs in terms of the overall weights W0 in the network and show an approximation rate of W0−p∕d. This more general result finally helps us to understand which network topology guarantees a special target accuracy.
更多查看译文
关键词
primary,secondary
AI 理解论文
溯源树
样例
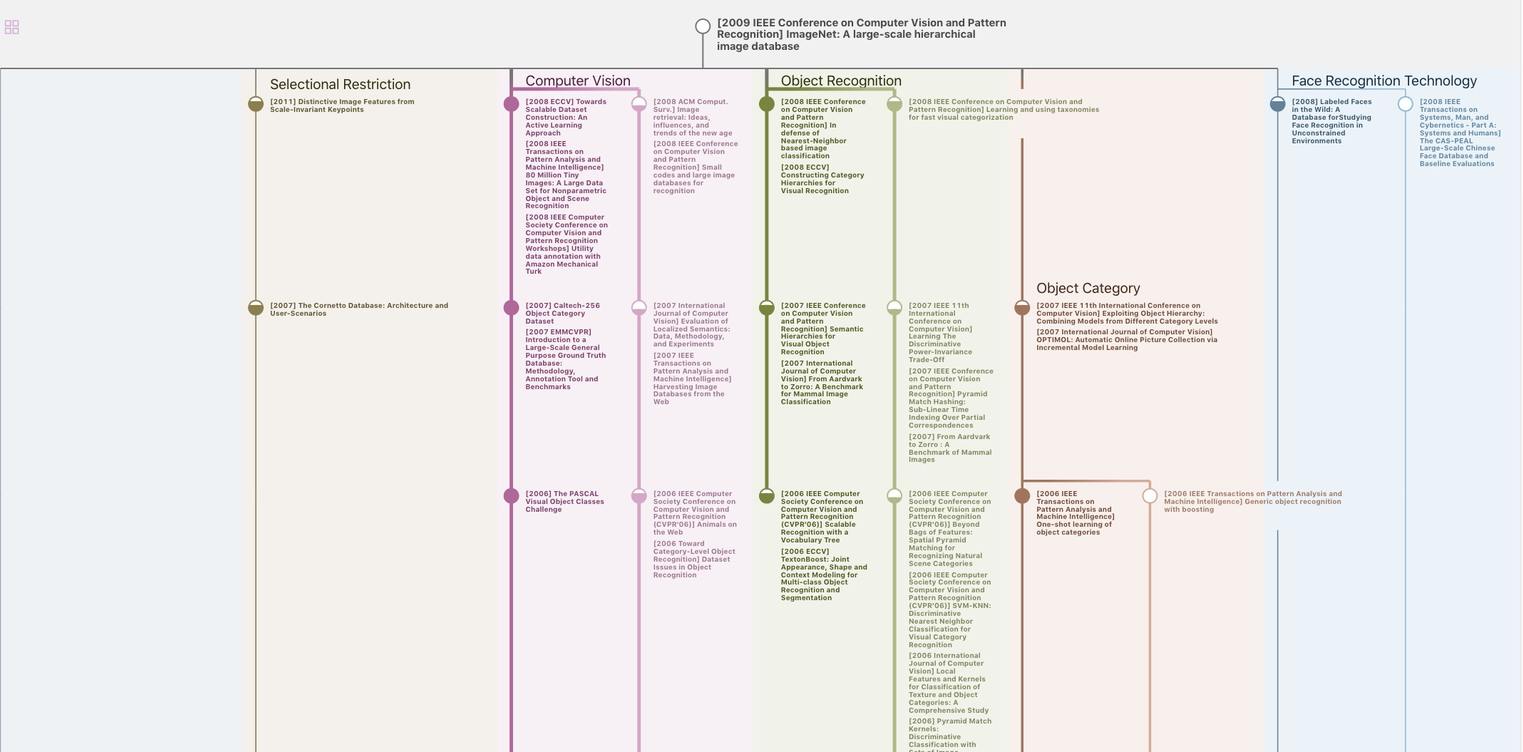
生成溯源树,研究论文发展脉络
Chat Paper
正在生成论文摘要