Content Analysis of Textbooks via Natural Language Processing: Findings on Gender, Race, and Ethnicity in Texas US History Textbooks
AERA OPEN(2020)
摘要
Cutting-edge data science techniques can shed new light on fundamental questions in educational research. We apply techniques from natural language processing (lexicons, word embeddings, topic models) to 15 U.S. history textbooks widely used in Texas between 2015 and 2017, studying their depiction of historically marginalized groups. We find that Latinx people are rarely discussed, and the most common famous figures are nearly all White men. Lexicon-based approaches show that Black people are described as performing actions associated with low agency and power. Word embeddings reveal that women tend to be discussed in the contexts of work and the home. Topic modeling highlights the higher prominence of political topics compared with social ones. We also find that more conservative counties tend to purchase textbooks with less representation of women and Black people. Building on a rich tradition of textbook analysis, we release our computational toolkit to support new research directions.
更多查看译文
关键词
artificial intelligence,case studies,content analysis,curriculum,data science,gender studies,history,natural language processing,race,textbooks,textual analysis
AI 理解论文
溯源树
样例
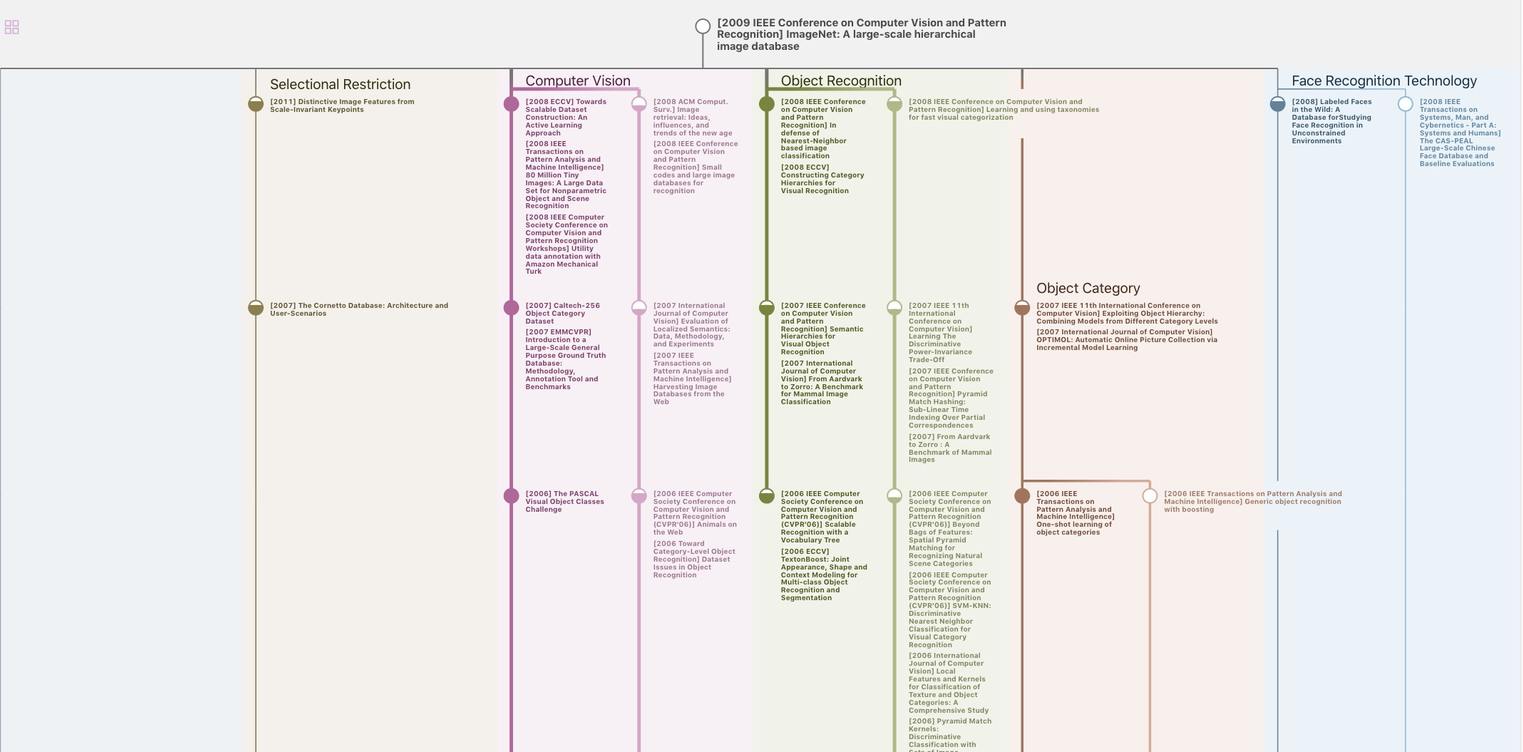
生成溯源树,研究论文发展脉络
Chat Paper
正在生成论文摘要