Astronomical Image Restoration and Point Spread Function Estimation with Deep Neural Networks
ADVANCES IN OPTICAL ASTRONOMICAL INSTRUMENTATION 2019(2020)
摘要
From ground-based extremely large telescopes to small telescope arrays used for time domain astronomy, point spread function plays an important role both for scientific data post-processing and instrument performance estimation. In this paper, we propose a new method which can restore astronomical images and obtain the point spread function of the whole optical system at the same time. Our method uses simulated high resolution astronomical images and real observed blurred images to train a deep neural network (Cycle-GAN). The Cycle-GAN contains a pair of generative adversarial neural networks and each generative adversarial neural network contains a generator and a discriminator. After training, one generator (PSF-Gen) can learn the point spread function and the other generator (Dec-Gen) can learn the deconvolution kernel. We test our method with real observation data from solar telescope and small aperture telescopes. We find that the Dec-Gen can give promising restoration results for solar images and can reduce the PSF spatial variation for images obtained by smaller telescopes. Besides, we also find that the PSF-Gen can provide a non-parametric PSF model for short exposure images, which would then be used as prior model for PSF reconstruction algorithms in adaptive optics systems.
更多查看译文
关键词
Point spread function reconstruction,Deep neural network,Cyclegan,Image restoration
AI 理解论文
溯源树
样例
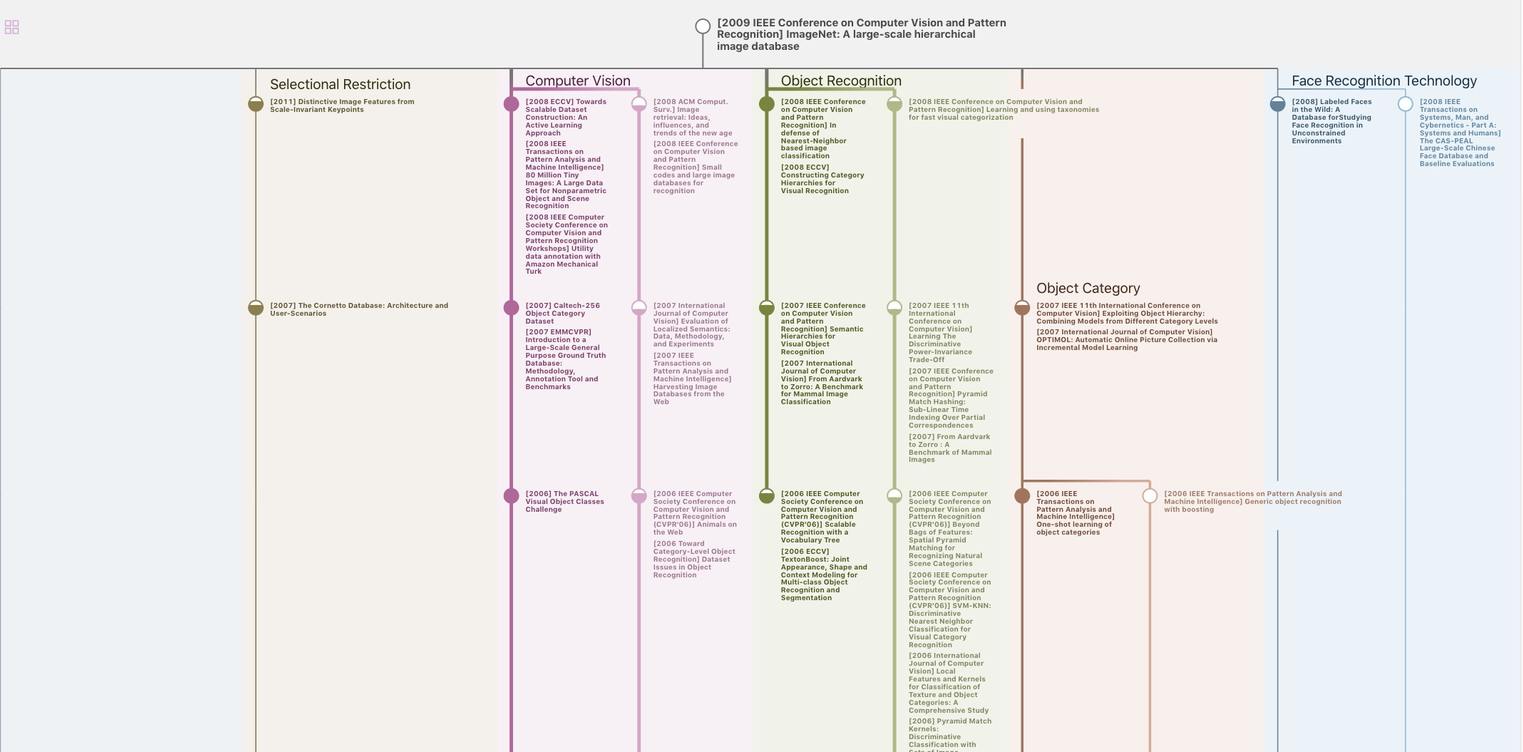
生成溯源树,研究论文发展脉络
Chat Paper
正在生成论文摘要