Bayesian Modeling of Associations in Bivariate Piecewise Linear Mixed-Effects Models
PSYCHOLOGICAL METHODS(2022)
摘要
Longitudinal processes rarely occur in isolation; often the growth curves of 2 or more variables are interdependent. Moreover, growth curves rarely exhibit a constant pattern of change. Many educational and psychological phenomena are comprised of different developmental phases (segments). Bivariate piecewise linear mixed-effects models (BPLMEM) are a useful and flexible statistical framework that allow simultaneous modeling of 2 processes that portray segmented change and investigates their associations over time. The purpose of the present study was to develop a BPLMEM using a Bayesian inference approach allowing the estimation of the association between the error variances and providing a more robust modeling choice for the joint random-effects of the 2 processes. This study aims to improve upon the limitations of the prior literature on bivariate piecewise mixed-effects models, such as only allowing the modeling of uncorrelated residual errors across the 2 longitudinal processes and restricting modeling choices for the random effects. The performance of the BPLMEM was investigated via a Monte Carlo simulation study. Furthermore, the utility of BPLMEM was illustrated by using a national educational dataset, Early Childhood Longitudinal Study-Kindergarten Cohort (ECLS-K), where we examined the joint development of mathematics and reading achievement scores and the association between their trajectories over 7 measurement occasions. The findings obtained shed new light on the relationship between these 2 prominent educational domains over time. Translational Abstract Longitudinal processes rarely occur in isolation; often the growth curves of two or more variables are interdependent. For instance, mathematics and reading achievement might not evolve independently over time but rather their trajectories may be associated along an educational time span. Moreover, longitudinal trajectories rarely exhibit a constant pattern of change. Many educational and psychological phenomena are comprised of different developmental phases (segments). Piecewise models describe nonlinear trajectories that have distinct rates of growth corresponding to different segments of development over time. The purpose of the present study was to develop a bivariate piecewise linear mixed-effects model (BPLMEM) under a Bayesian framework to allow simultaneous modeling of two processes that depict segmented change and investigate their associations over time. That is, two longitudinal variables of interest that portray differentiated segments of growth are modeled simultaneously with the objective of examining the relation between the growth curves as they unfold over the time span under study. The proposed Bayesian BPLMEM aims to improve upon the limitations of previous literature. Model performance was investigated via a Monte Carlo simulation study. Furthermore, the utility of the Bayesian BPLMEM was illustrated by using a national educational dataset, Early Childhood Longitudinal Study-Kindergarten Cohort (ECLS-K), where we examined the joint development of mathematics and reading achievement scores and the association between their trajectories over seven measurement occasions. The findings obtained shed new light on the relationship between these two prominent educational domains over time.
更多查看译文
关键词
bivariate models, Bayesian modeling, bivariate nonlinear mixed-effects models, piecewise models, segmented processes
AI 理解论文
溯源树
样例
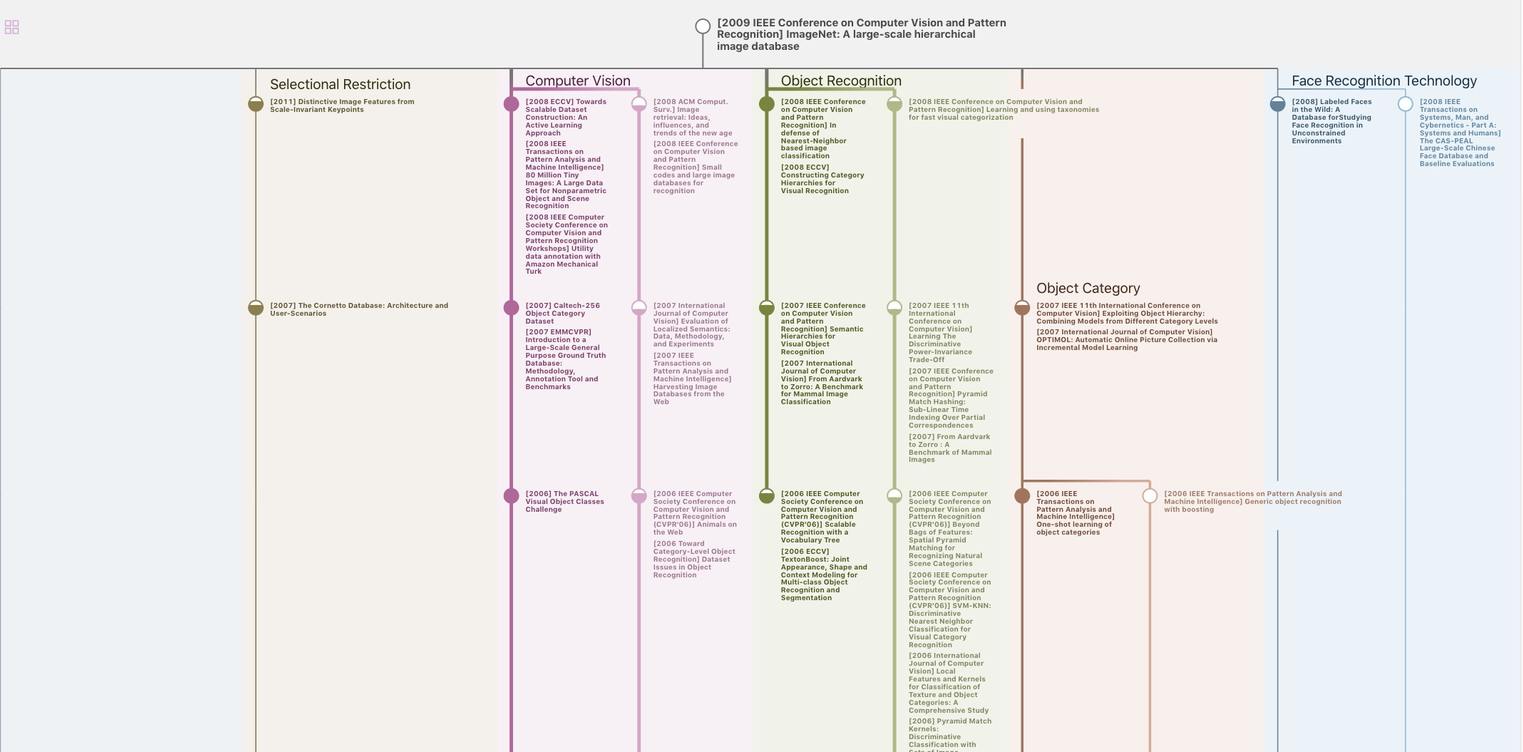
生成溯源树,研究论文发展脉络
Chat Paper
正在生成论文摘要