Fuzzy-Rough Cognitive Networks: Theoretical Analysis and Simpler Models
IEEE Transactions on Cybernetics(2022)
摘要
Fuzzy-rough cognitive networks (FRCNs) are recurrent neural networks (RNNs) intended for structured classification purposes in which the problem is described by an explicit set of features. The advantage of this granular neural system relies on its transparency and simplicity while being competitive to state-of-the-art classifiers. Despite their relative empirical success in terms of prediction rates, there are limited studies on FRCNs’ dynamic properties and how their building blocks contribute to the algorithm’s performance. In this article, we theoretically study these issues and conclude that boundary and negative neurons always converge to a
unique
fixed-point attractor. Moreover, we demonstrate that negative neurons have no impact on the algorithm’s performance and that the ranking of positive neurons is invariant. Moved by our theoretical findings, we propose two simpler fuzzy-rough classifiers that overcome the detected issues and maintain the competitive prediction rates of this classifier. Toward the end, we present a case study concerned with image classification, in which a convolutional neural network is coupled with one of the simpler models derived from the theoretical analysis of the FRCN model. The numerical simulations suggest that once the features have been extracted, our granular neural system performs as well as other RNNs.
更多查看译文
关键词
Cognition,Fuzzy Logic,Models, Theoretical,Neural Networks, Computer,Neurons
AI 理解论文
溯源树
样例
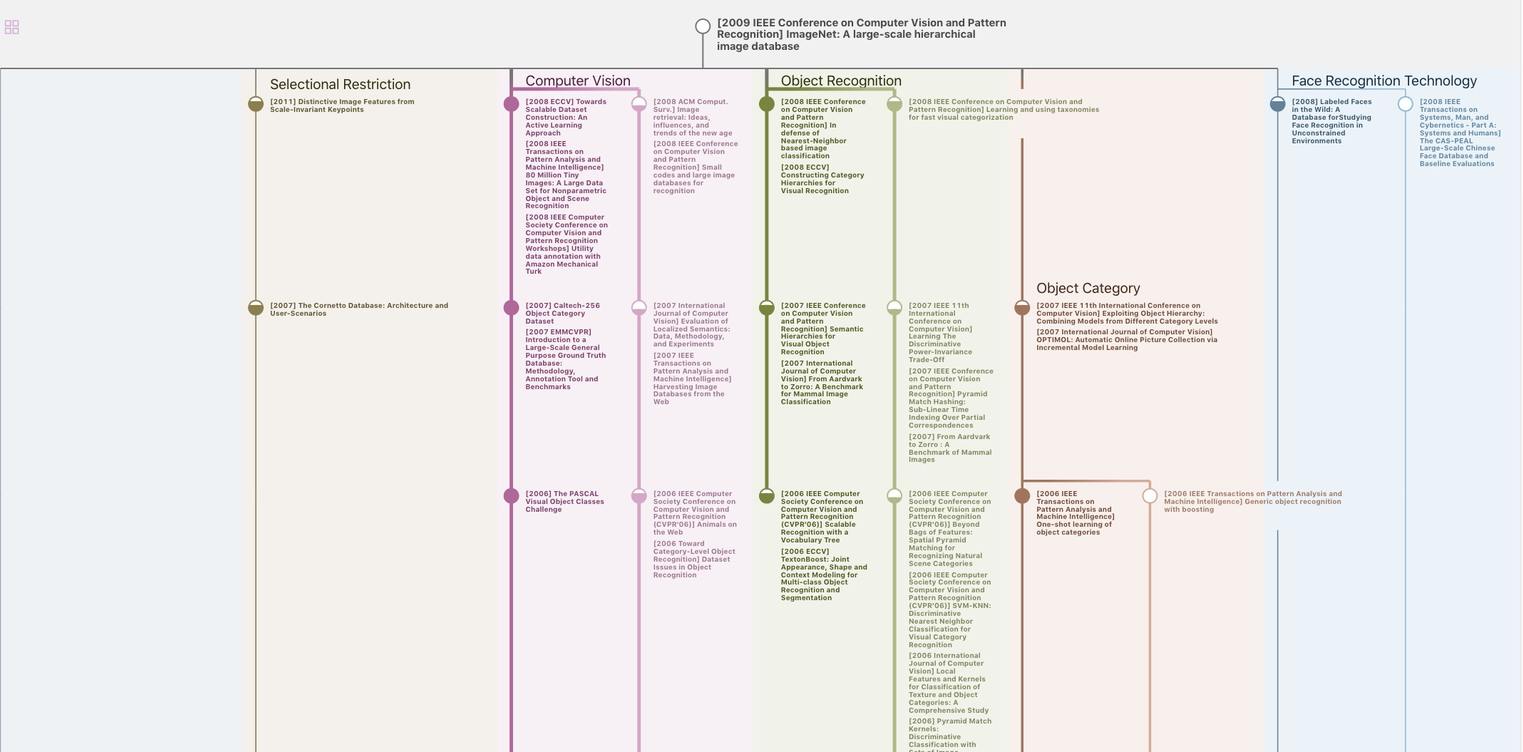
生成溯源树,研究论文发展脉络
Chat Paper
正在生成论文摘要