Event Detection Based on Nonnegative Matrix Factorization: Ceasefire Violation, Environmental, and Malware Events
ADVANCES IN HUMAN FACTORS IN CYBERSECURITY(2018)
摘要
Event detection is a very important problem across many domains and is a broadly applicable encompassing many disciplines within engineering systems. In this paper, we focus on improving the user's ability to quickly identify threat events such as malware, military policy violations, and natural environmental disasters. The information to perform these detections is extracted from text data sets in the latter two cases. Malware threats are important as they compromise computer system integrity and potentially allow the collection of sensitive information. Military policy violations such as ceasefire policies are important to monitor as they disrupt the daily lives of many people within countries that are torn apart by social violence or civil war. The threat of environmental disasters takes many forms and is an ever-present danger worldwide, and indiscriminate regarding who is harmed or killed. In this paper, we address all three of these threat event types using the same underlying technology for mining the information that leads to detecting such events. We approach malware event detection as a binary classification problem, i.e., one class for the threat mode and another for non-threat mode. We extend our novel classifier utilizing constrained low rank approximation as the core algorithm innovation and apply our Non-negative Generalized Moody-Darken Architecture (NGMDA) hybrid method using various combinations of input and output layer algorithms. The new algorithm uses a nonconvex optimization problem via the nonnegative matrix factorization (NMF) for the hidden layer of a single layer perceptron and a nonnegative constrained adaptive filter for the output layer estimator. We first show the utility of the core NMF technology for both ceasefire violation and environmental disaster event detection. Next NGMDA is applied to the problem of malware threat events, again based on the NMF as the core computational tool. Also, we demonstrate that an algorithm should be appropriately selected for the data generation process. All this has critical implications for design of solutions for important threat/event detection scenarios. Lastly, we present experimental results on foreign language text for ceasefire violation and environmental disaster events. Experimental results on a KDD competition data set for malware classification are presented using our new NGMDA classifier.
更多查看译文
关键词
Malware detection,Event detection,Perceptron,Clustering,Nonnegative matrix factorization,Adaptive filtering,Hybrid classifier,Topic modeling,Classification
AI 理解论文
溯源树
样例
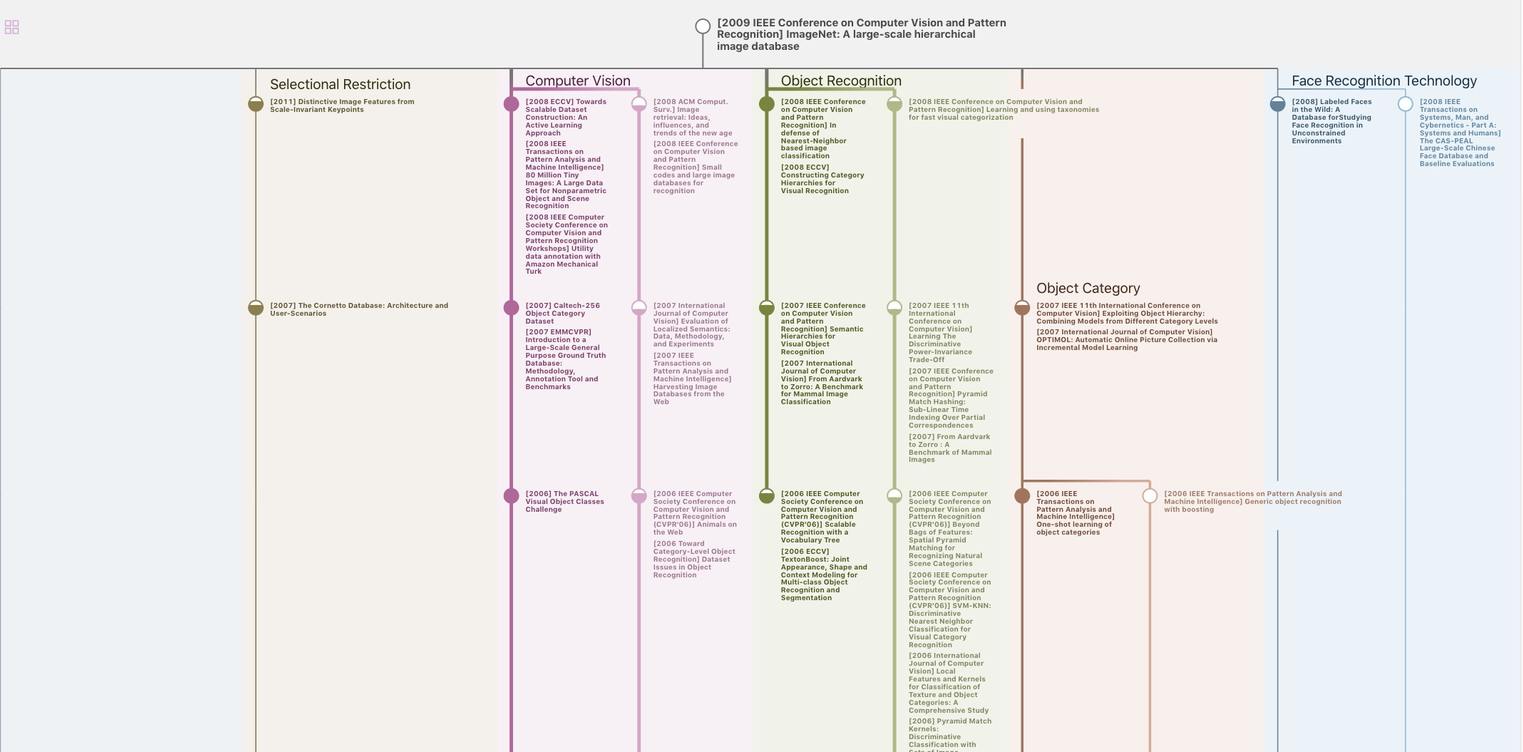
生成溯源树,研究论文发展脉络
Chat Paper
正在生成论文摘要