An Empirical Study of Pre-trained Transformers for Arabic Information Extraction
Conference on Empirical Methods in Natural Language Processing(2020)
摘要
Multilingual pre-trained Transformers, such as mBERT (Devlin et al., 2019) and XLM-RoBERTa (Conneau et al., 2020a), have been shown to enable effective cross-lingual zero-shot transfer. However, their performance on Arabic information extraction (IE) tasks is not very well studied. In this paper, we pre-train a customized bilingual BERT, dubbed GigaBERT, that is designed specifically for Arabic NLP and English-to-Arabic zero-shot transfer learning. We study GigaBERT’s effectiveness on zero-short transfer across four IE tasks: named entity recognition, part-of-speech tagging, argument role labeling, and relation extraction. Our best model significantly outperforms mBERT, XLM-RoBERTa, and AraBERT (Antoun et al., 2020) in both the supervised and zero-shot transfer settings. We have made our pre-trained models publicly available at: https://github.com/lanwuwei/GigaBERT.
更多查看译文
关键词
arabic information
AI 理解论文
溯源树
样例
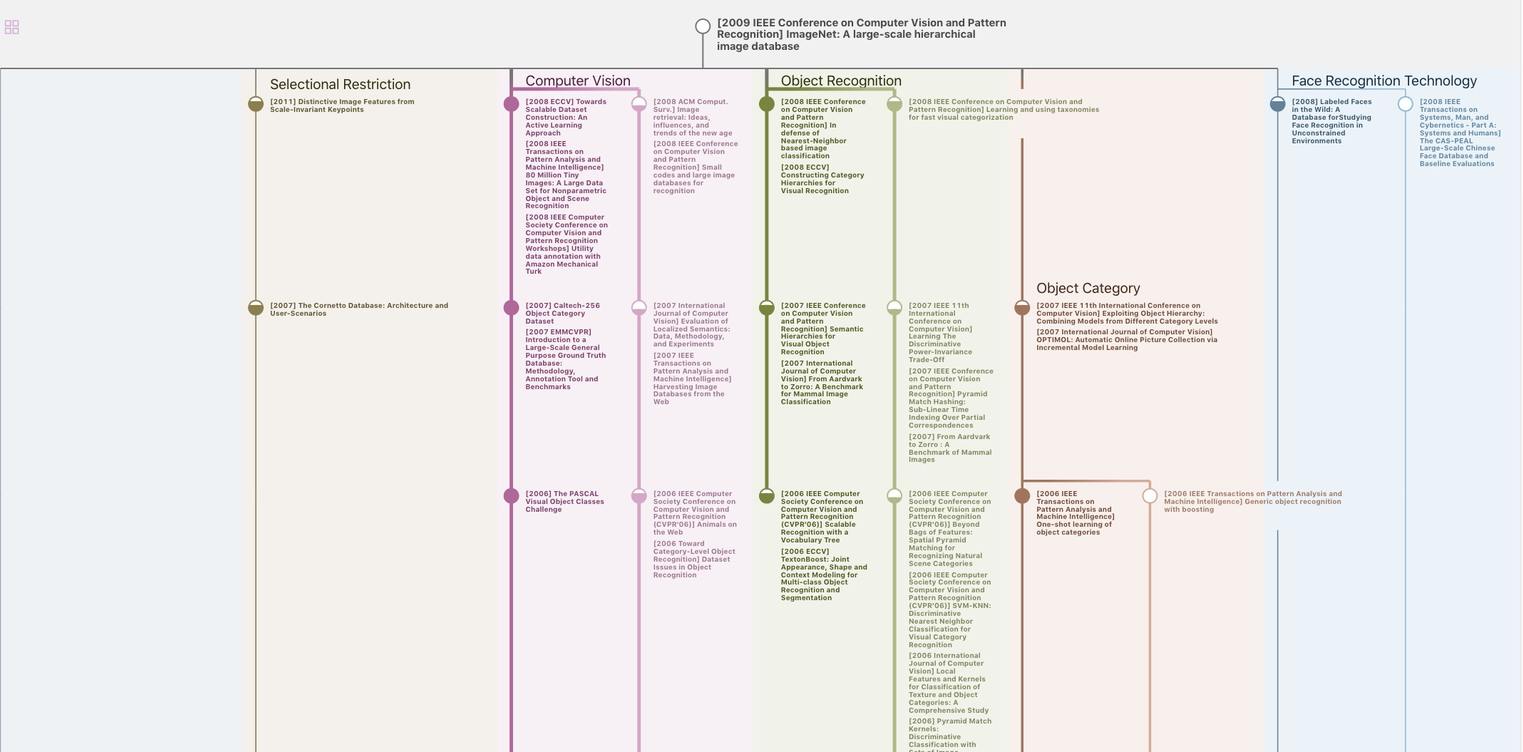
生成溯源树,研究论文发展脉络
Chat Paper
正在生成论文摘要