Filtering Noisy Dialogue Corpora by Connectivity and Content Relatedness
Conference on Empirical Methods in Natural Language Processing(2020)
摘要
Large-scale dialogue datasets have recently become available for training neural dialogue agents. However, these datasets have been reported to contain a non-negligible number of unacceptable utterance pairs. In this paper, we propose a method for scoring the quality of utterance pairs in terms of their connectivity and relatedness. The proposed scoring method is designed based on findings widely shared in the dialogue and linguistics research communities. We demonstrate that it has a relatively good correlation with the human judgment of dialogue quality. Furthermore, the method is applied to filter out potentially unacceptable utterance pairs from a large-scale noisy dialogue corpus to ensure its quality. We experimentally confirm that training data filtered by the proposed method improves the quality of neural dialogue agents in response generation.
更多查看译文
关键词
filtering noisy dialogue corpora,relatedness,connectivity
AI 理解论文
溯源树
样例
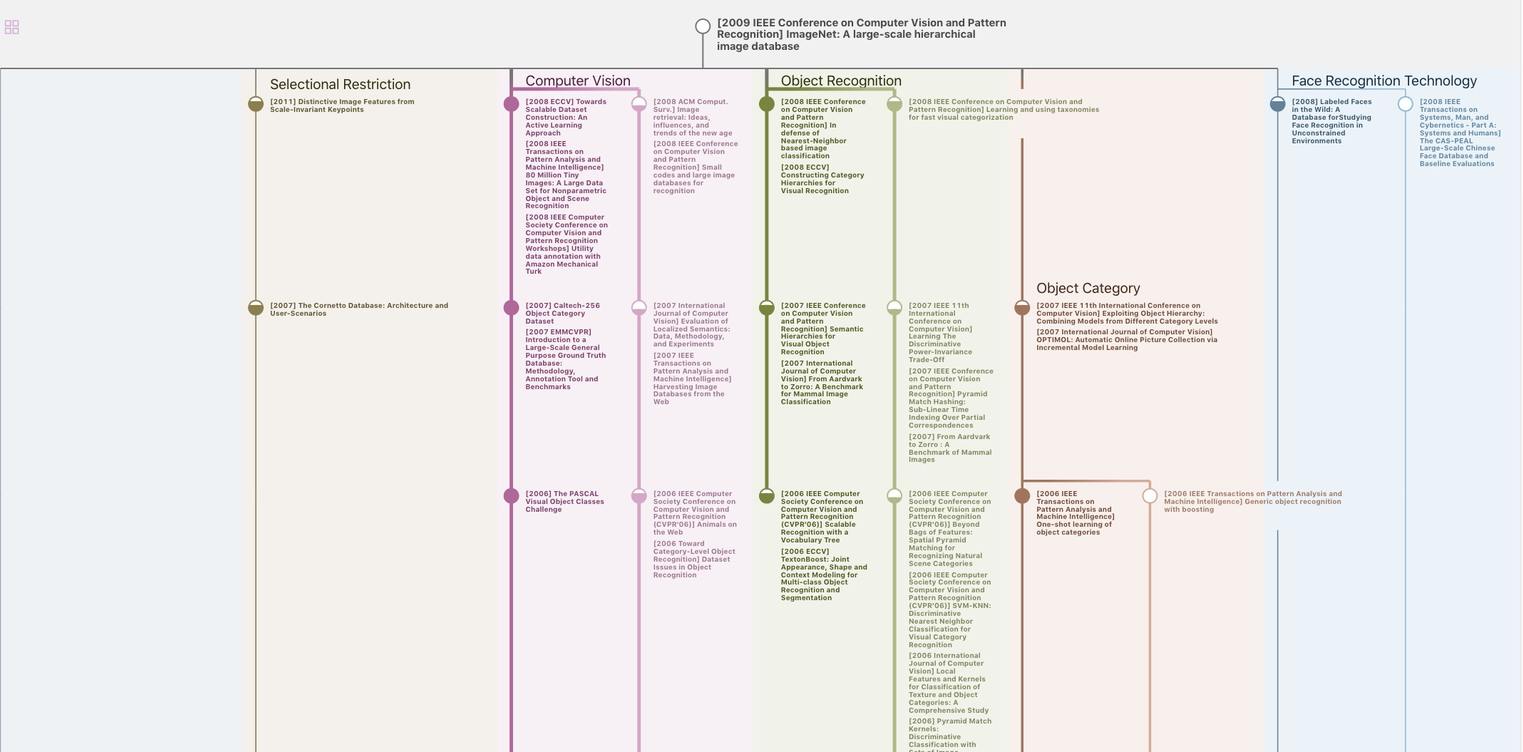
生成溯源树,研究论文发展脉络
Chat Paper
正在生成论文摘要