ETC: Encoding Long and Structured Inputs in Transformers
Conference on Empirical Methods in Natural Language Processing(2020)
摘要
Transformer models have advanced the state of the art in many Natural Language Processing (NLP) tasks. In this paper, we present a new Transformer architecture, “Extended Transformer Construction” (ETC), that addresses two key challenges of standard Transformer architectures, namely scaling input length and encoding structured inputs. To scale attention to longer inputs, we introduce a novel global-local attention mechanism between global tokens and regular input tokens. We also show that combining global-local attention with relative position encodings and a “Contrastive Predictive Coding” (CPC) pre-training objective allows ETC to encode structured inputs. We achieve state-of-the-art results on four natural language datasets requiring long and/or structured inputs.
更多查看译文
关键词
structured inputs,encoding
AI 理解论文
溯源树
样例
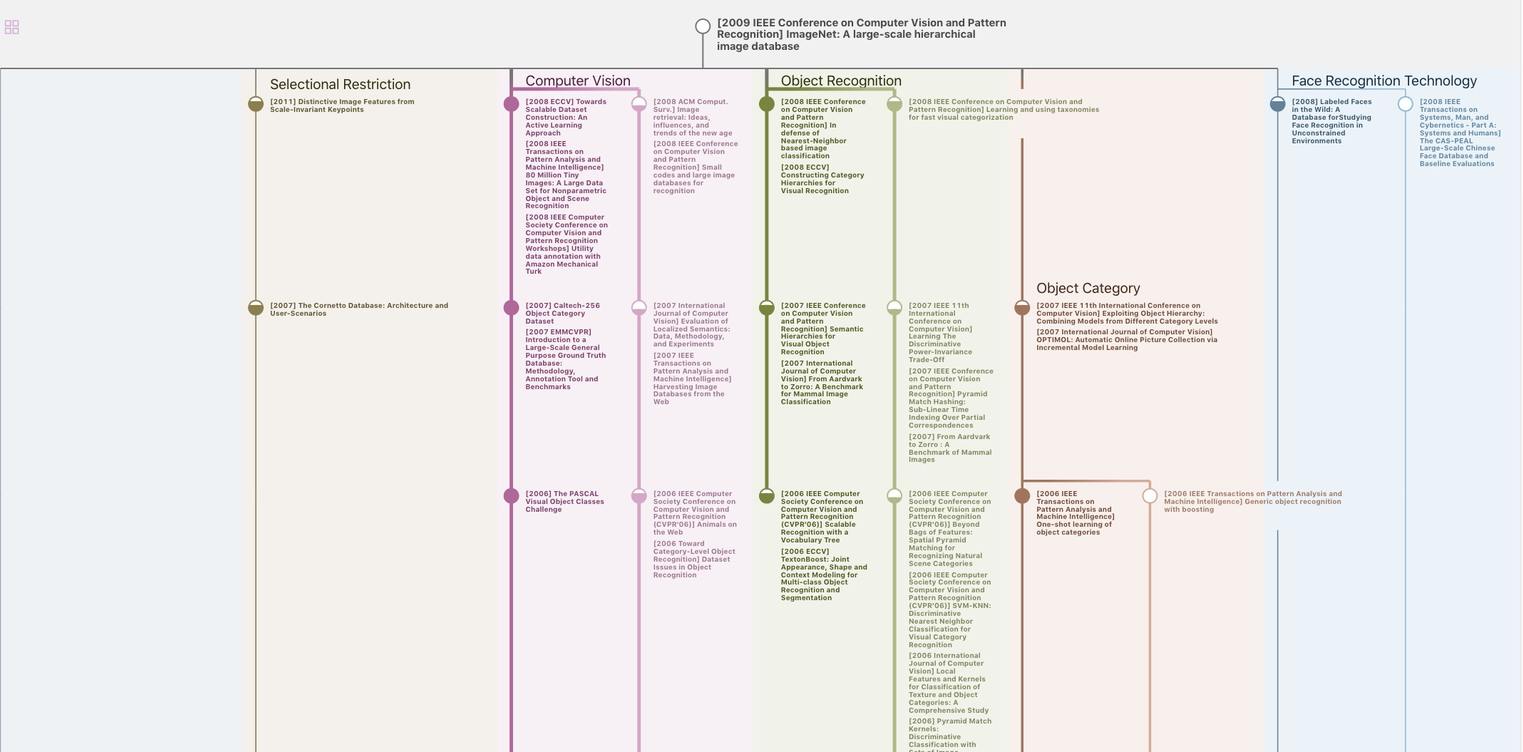
生成溯源树,研究论文发展脉络
Chat Paper
正在生成论文摘要