DAGA: Data Augmentation with a Generation Approach for Low resource Tagging Tasks
EMNLP 2020(2020)
摘要
Data augmentation techniques have been widely used to improve machine learning performance as they facilitate generalization. In this work, we propose a novel augmentation method to generate high quality synthetic data for low-resource tagging tasks with language models trained on the linearized labeled sentences. Our method is applicable to both supervised and semi-supervised settings. For the supervised settings, we conduct extensive experiments on named entity recognition (NER), part of speech (POS) tagging and end-to-end target based sentiment analysis (E2E-TBSA) tasks. For the semi-supervised settings, we evaluate our method on the NER task under the conditions of given unlabeled data only and unlabeled data plus a knowledge base. The results show that our method can consistently outperform the baselines, particularly when the given gold training data are less.
更多查看译文
关键词
tagging tasks,data augmentation,generation approach,low-resource
AI 理解论文
溯源树
样例
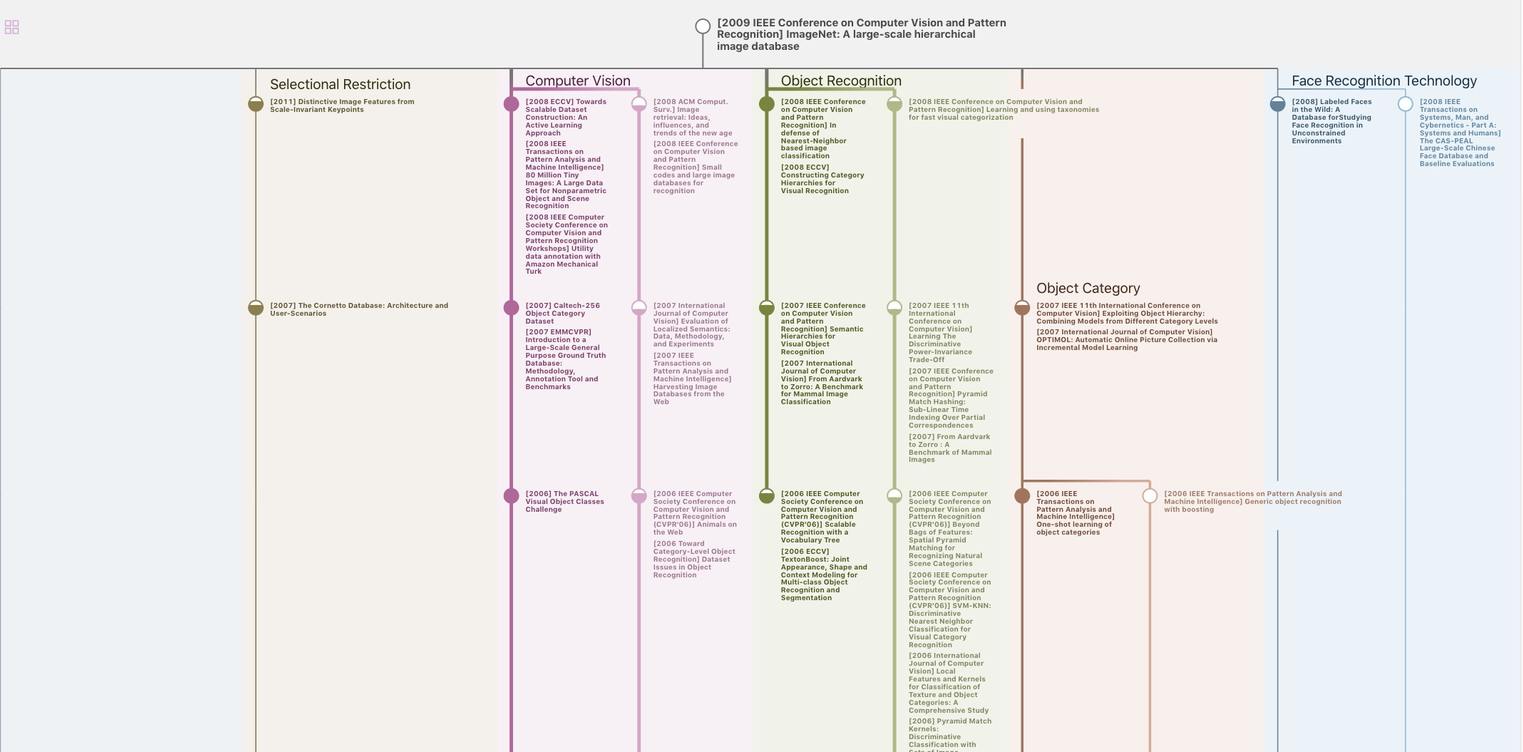
生成溯源树,研究论文发展脉络
Chat Paper
正在生成论文摘要