Experimental design for MRI by greedy policy search
NIPS 2020(2020)
摘要
In today's clinical practice, magnetic resonance imaging (MRI) is routinely accelerated through subsampling of the associated Fourier domain. Currently, the construction of these subsampling strategies - known as experimental design - relies primarily on heuristics. We propose to learn experimental design strategies for accelerated MRI with policy gradient methods. Unexpectedly, our experiments show that a simple greedy approximation of the objective leads to solutions nearly on-par with the more general non-greedy approach. We offer a partial explanation for this phenomenon rooted in greater variance in the non-greedy objective's gradient estimates, and experimentally verify that this variance hampers non-greedy models in adapting their policies to individual MR images. We empirically show that this adaptivity is key to improving subsampling designs.
更多查看译文
关键词
mri,greedy policy search,experimental design
AI 理解论文
溯源树
样例
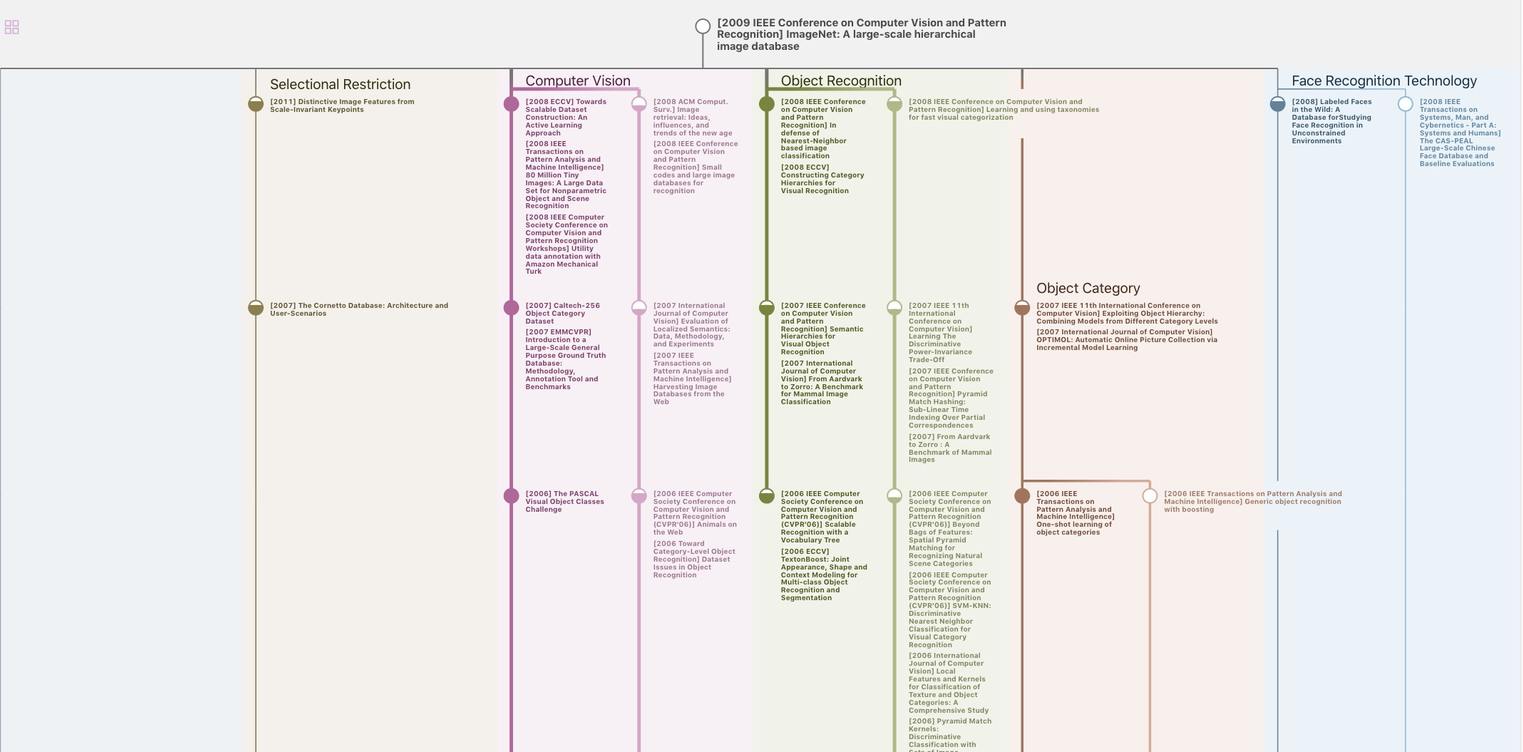
生成溯源树,研究论文发展脉络
Chat Paper
正在生成论文摘要