Interpolation Technique to Speed Up Gradients Propagation in Neural ODEs
NIPS 2020(2020)
摘要
We propose a simple interpolation-based method for the efficient approximation of gradients in neural ODE models. We compare it with reverse dynamic method (known in literature as “adjoint method”) to train neural ODEs on classification, density estimation and inference approximation tasks. We also propose a theoretical justification of our approach using logarithmic norm formalism. As a result, our method allows faster model training than the reverse dynamic method what was confirmed and validated by extensive numerical experiments for several standard benchmarks.
更多查看译文
关键词
neural odes,interpolation,gradients propagation
AI 理解论文
溯源树
样例
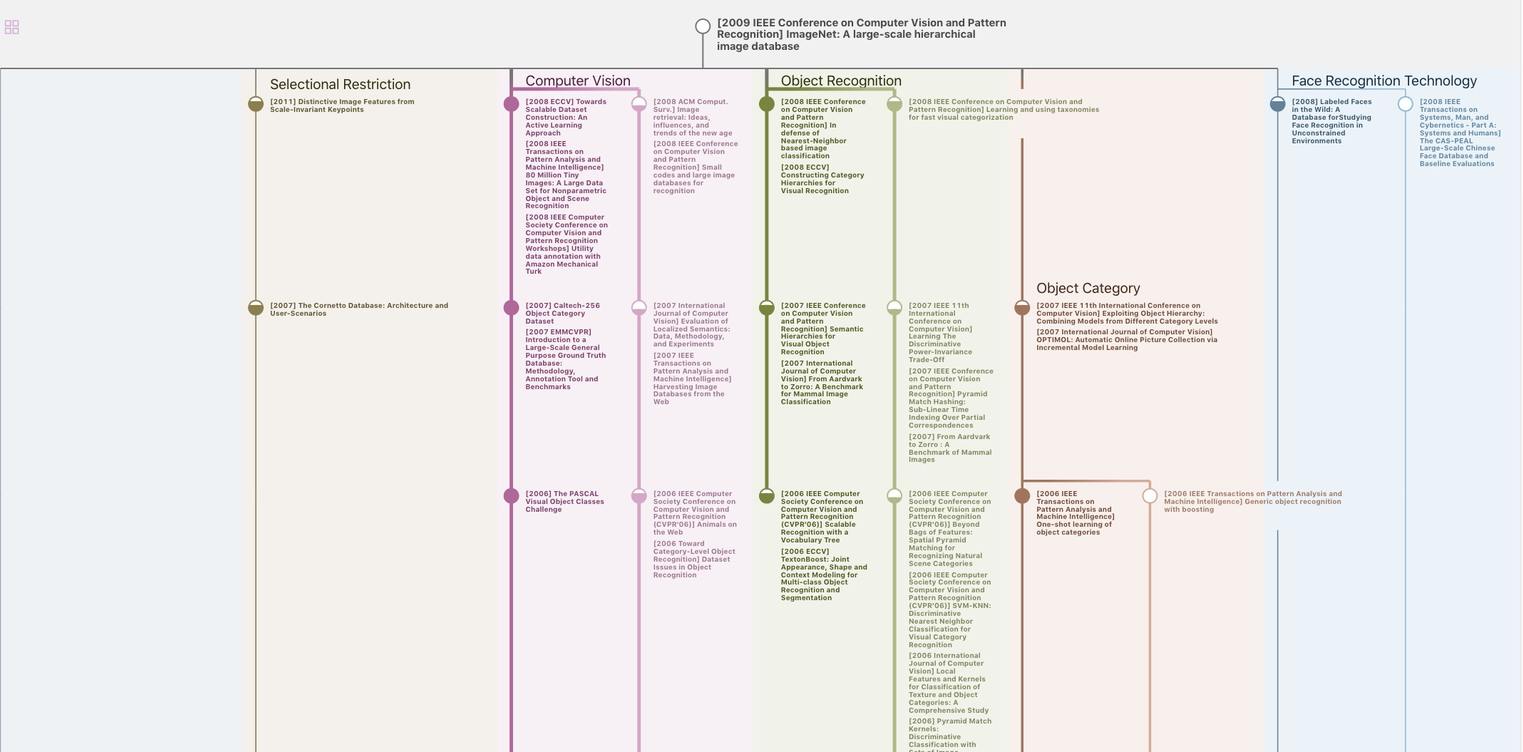
生成溯源树,研究论文发展脉络
Chat Paper
正在生成论文摘要