Continual Learning in Low-rank Orthogonal Subspaces
NIPS 2020(2020)
摘要
In continual learning (CL), a learner is faced with a sequence of tasks, arriving one after the other, and the goal is to remember all the tasks once the continual learning experience is finished. The prior art in CL uses episodic memory, parameter regularization or extensible network structures to reduce interference among tasks, but in the end, all the approaches learn different tasks in a joint vector space. We believe this invariably leads to interference among different tasks. We propose to learn tasks in different (low-rank) vector subspaces that are kept orthogonal to each other in order to minimize interference. Further, to keep the gradients of different tasks coming from these subspaces orthogonal to each other, we learn isometric mappings by posing network training as an optimization problem over the Stiefel manifold. To the best of our understanding, we report, for the first time, strong results over experience-replay baseline with and without memory on standard classification benchmarks in continual learning. The code is made publicly available.
更多查看译文
关键词
continual learning,low-rank
AI 理解论文
溯源树
样例
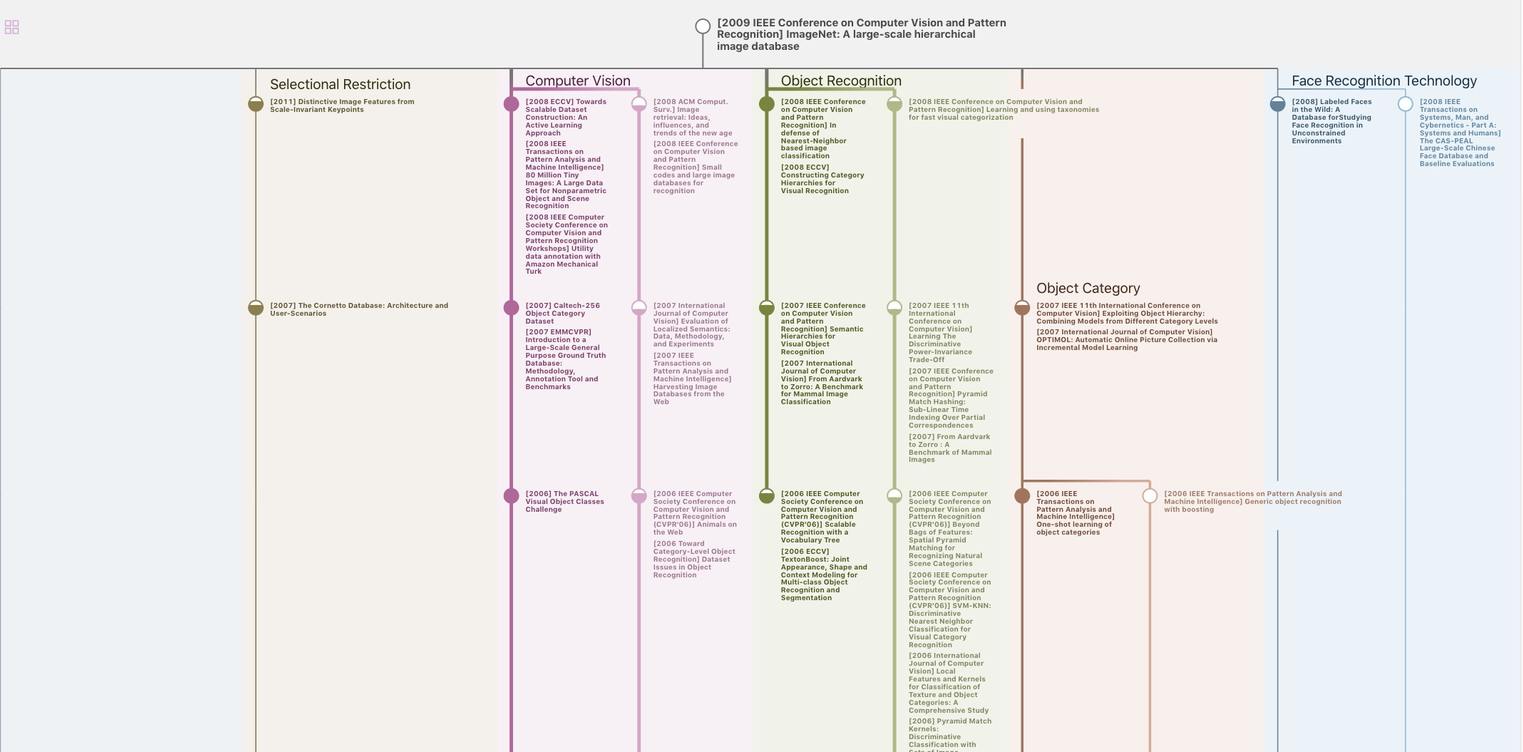
生成溯源树,研究论文发展脉络
Chat Paper
正在生成论文摘要