Deep Imitation Learning for Bimanual Robotic Manipulation
NIPS 2020(2020)
摘要
We present a deep imitation learning framework for robotic bimanual manipulation in a continuous state-action space. Imitation learning has been effectively utilized in mimicking bimanual manipulation movements, but generalizing the movement to objects in different locations has not been explored. We hypothesize that to precisely generalize the learned behavior relative to an object's location requires modeling relational information in the environment. To achieve this, we designed a method that (i) uses a multi-model framework to decomposes complex dynamics into elemental movement primitives, and (ii) parameterizes each primitive using a recurrent graph neural network to capture interactions. Our model is a deep, hierarchical, modular architecture with a high-level planner that learns to compose primitives sequentially and a low-level controller which integrates primitive dynamics modules and inverse kinematics control. We demonstrate the effectiveness using several simulated bimanual robotic manipulation tasks. Compared to models based on previous imitation learning studies, our model generalizes better and achieves higher success rates in the simulated tasks.
更多查看译文
关键词
manipulation,learning,deep
AI 理解论文
溯源树
样例
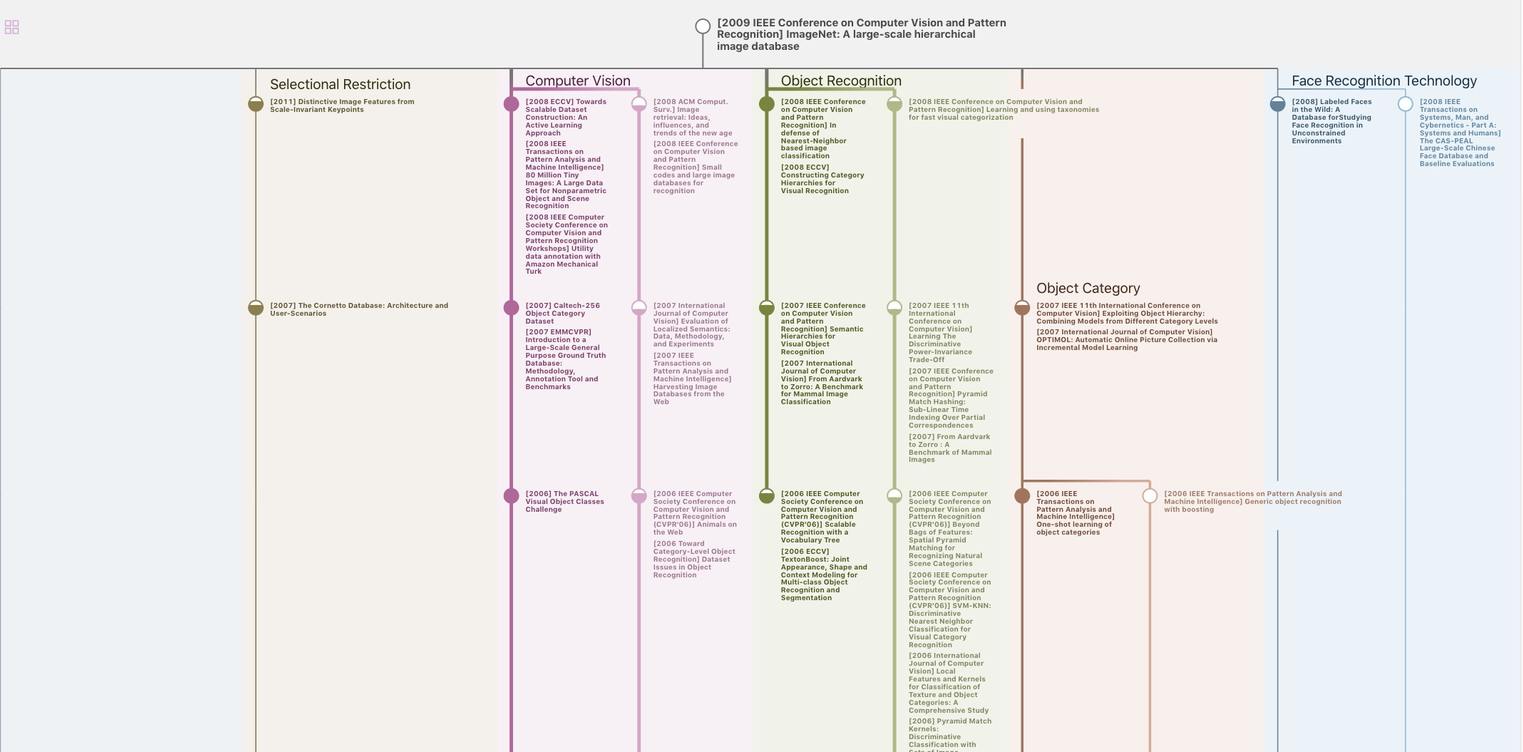
生成溯源树,研究论文发展脉络
Chat Paper
正在生成论文摘要