Regret Bounds without Lipschitz Continuity: Online Learning with Relative-Lipschitz Losses
NIPS 2020(2020)
摘要
In online convex optimization (OCO), Lipschitz continuity of the functions is commonly assumed in order to obtain sublinear regret. Moreover, many algorithms have only logarithmic regret when these functions are also strongly convex. Recently, researchers from convex optimization proposed the notions of "relative Lipschitz continuity" and "relative strong convexity". Both of the notions are generalizations of their classical counterparts. It has been shown that subgradient methods in the relative setting have performance analogous to their performance in the classical setting. In this work, we consider OCO for relative Lipschitz and relative strongly convex functions. We extend the known regret bounds for classical OCO algorithms to the relative setting. Specifically, we show regret bounds for the follow the regularized leader algorithms and a variant of online mirror descent. Due to the generality of these methods, these results yield regret bounds for a wide variety of OCO algorithms. Furthermore, we further extend the results to algorithms with extra regularization such as regularized dual averaging.
更多查看译文
关键词
online learning,lipschitz continuity,relative-lipschitz
AI 理解论文
溯源树
样例
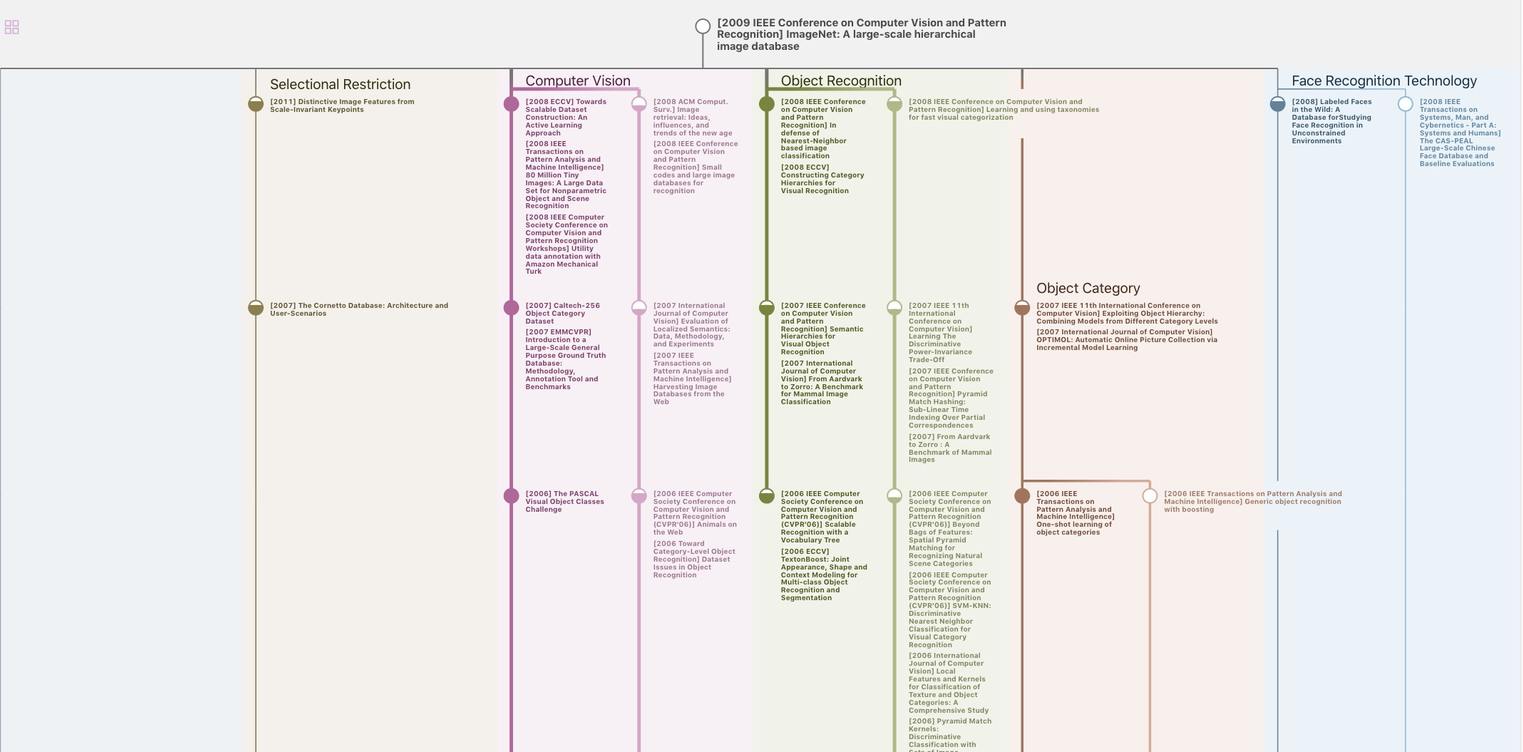
生成溯源树,研究论文发展脉络
Chat Paper
正在生成论文摘要