Efficient Projection-free Algorithms for Saddle Point Problems
NIPS 2020(2020)
摘要
The Frank-Wolfe algorithm is a classic method for constrained optimization problems. It has recently been popular in many machine learning applications because its projection-free property leads to more efficient iterations. In this paper, we study projection-free algorithms for convex-strongly-concave saddle point problems with complicated constraints. Our method combines Conditional Gradient Sliding with Mirror-Prox and shows that it only requires $\tilde{O}(1/\sqrt{\epsilon})$ gradient evaluations and $\tilde{O}(1/\epsilon^2)$ linear optimizations in the batch setting. We also extend our method to the stochastic setting and propose first stochastic projection-free algorithms for saddle point problems. Experimental results demonstrate the effectiveness of our algorithms and verify our theoretical guarantees.
更多查看译文
关键词
algorithms,projection-free
AI 理解论文
溯源树
样例
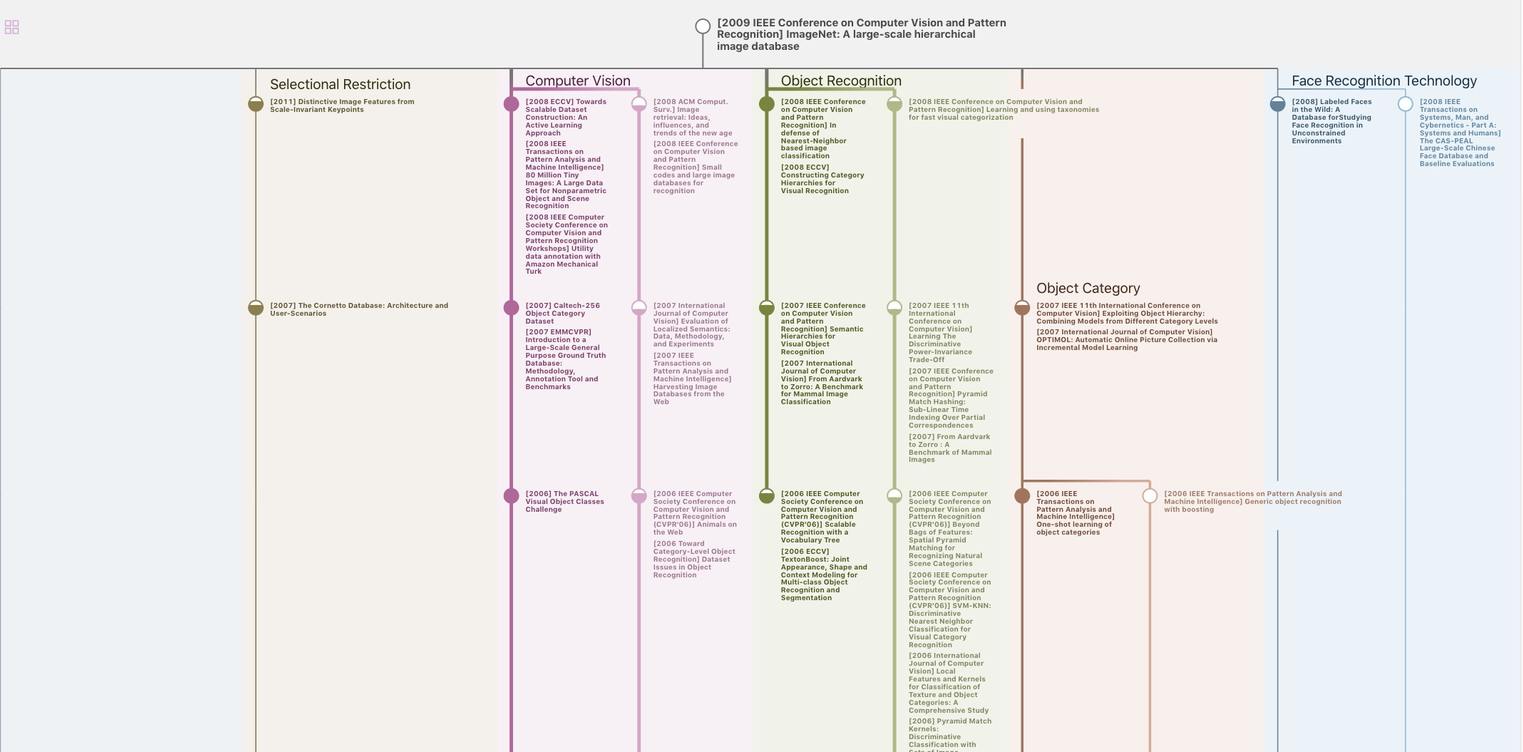
生成溯源树,研究论文发展脉络
Chat Paper
正在生成论文摘要