Fault Detection Based on Modified t-SNE
2019 CAA Symposium on Fault Detection, Supervision and Safety for Technical Processes (SAFEPROCESS)(2019)
摘要
Dimension reduction is a general step to process high dimensional data for fault detection. Principal component analysis (PCA) divides data space into principal component space and residual space. But it is a global method without considering local geometric properties between data points. Concentrating on local structure of data, manifold learning can be introduced in dynamic and continuous process for fault detection. It can extract latent features of data, and also be viewed as nonlinear dimension reduction. In this paper, we propose a modified t-SNE algorithm for fault detection, simultaneously considering local structure and different scales of variables. Modified t-SNE converts Mahalanobis distance to the conditional probability for representing pairwise similarities instead of Euclidean distance, which satisfies the characteristics of industrial process data. A subspace can be obtained from high-dimension to low-dimension by applying modified t-SNE, which effectively preserves local structure. Simulation on Tennessee Eastman process (TEP) demonstrates the effectiveness of our proposed method.
更多查看译文
关键词
dimension reduction,fault detection,local structure,modified t-SNE,mahalanobis distance
AI 理解论文
溯源树
样例
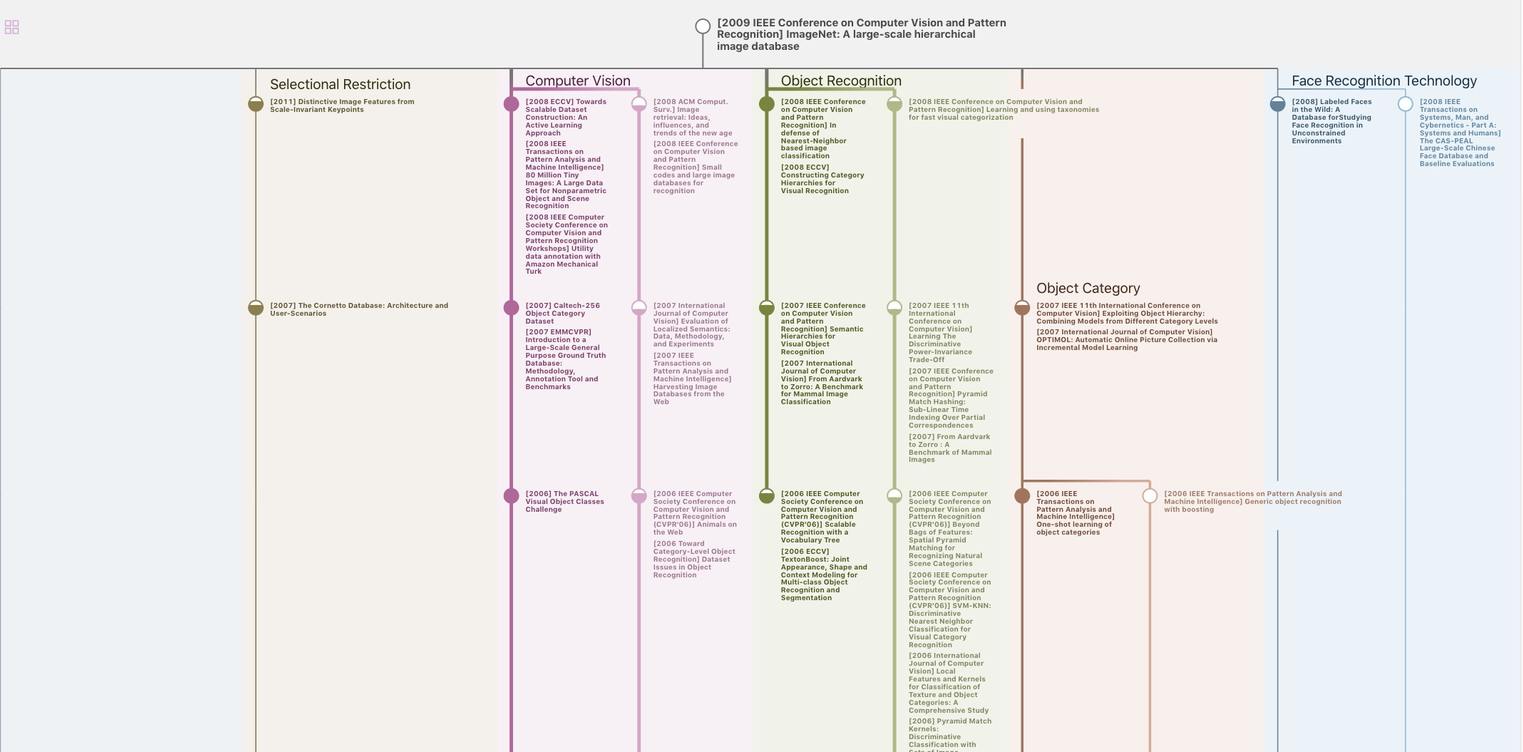
生成溯源树,研究论文发展脉络
Chat Paper
正在生成论文摘要