Learning Monocular 3D Vehicle Detection without 3D Bounding Box Labels
GCPR(2020)
摘要
The training of deep-learning-based 3D object detectors requires large datasets with 3D bounding box labels for supervision that have to be generated by hand-labeling. We propose a network architecture and training procedure for learning monocular 3D object detection without 3D bounding box labels. By representing the objects as triangular meshes and employing differentiable shape rendering, we define loss functions based on depth maps, segmentation masks, and ego- and object-motion, which are generated by pre-trained, off-the-shelf networks. We evaluate the proposed algorithm on the real-world KITTI dataset and achieve promising performance in comparison to state-of-the-art methods requiring 3D bounding box labels for training and superior performance to conventional baseline methods.
更多查看译文
关键词
3D object detection, Differentiable rendering, Autonomous driving
AI 理解论文
溯源树
样例
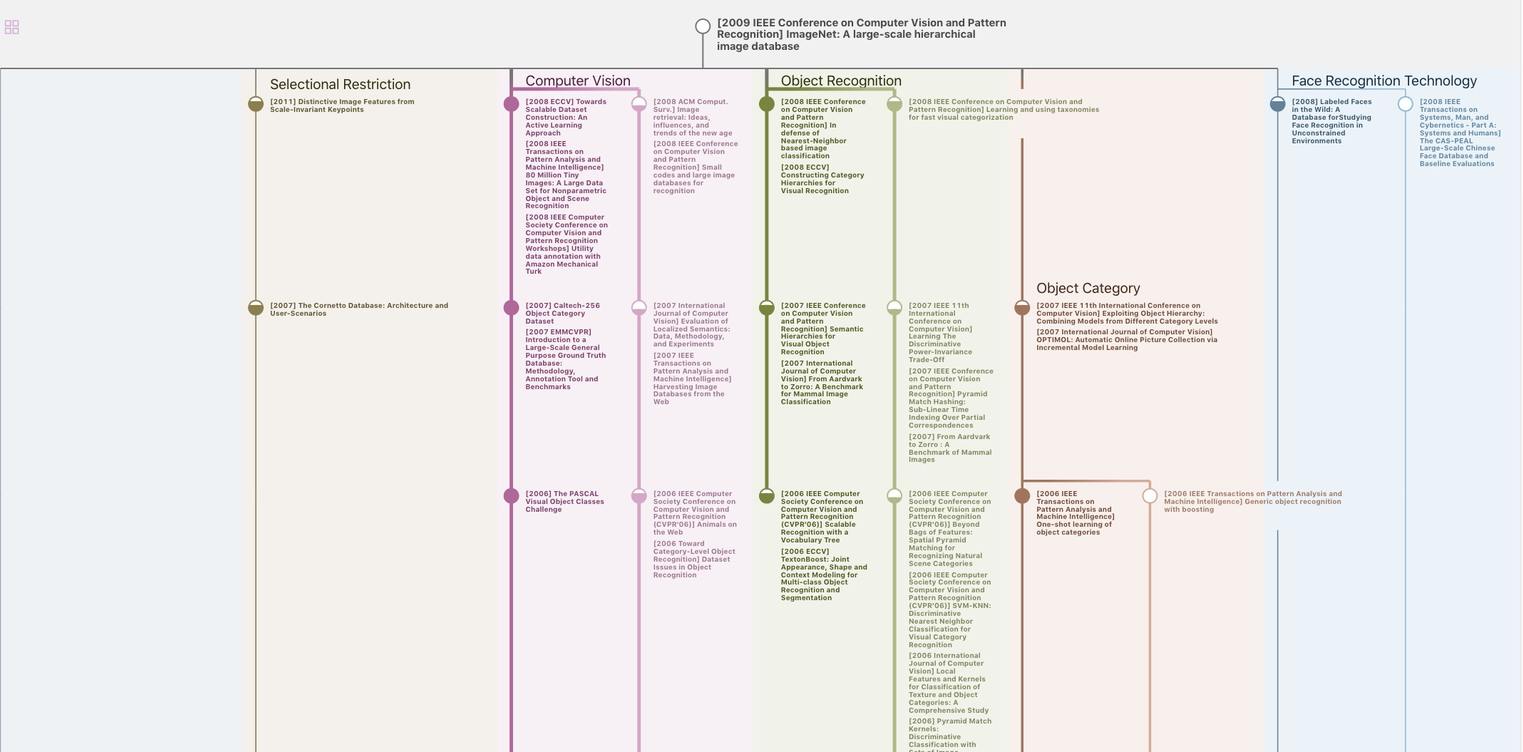
生成溯源树,研究论文发展脉络
Chat Paper
正在生成论文摘要