HIGH-DIMENSIONAL ASYMPTOTICS OF LIKELIHOOD RATIO TESTS IN THE GAUSSIAN SEQUENCE MODEL UNDER CONVEX CONSTRAINTS
arxiv(2022)
摘要
In the Gaussian sequence model Y = mu + xi, we study the likelihood ratio test (LRT) for testing H-0 : mu = mu(0) versus H-1 : mu is an element of K, where mu(0) is an element of K, and K is a closed convex set in R-n. In particular, we show that under the null hypothesis, normal approximation holds for the log-likelihood ratio statistic for a general pair (mu(0), K), in the high-dimensional regime where the estimation error of the associated least squares estimator diverges in an appropriate sense. The normal approximation further leads to a precise characterization of the power behavior of the LRT in the high-dimensional regime. These characterizations show that the power behavior of the LRT is in general nonuniform with respect to the Euclidean metric, and illustrate the conservative nature of existing minimax optimality and suboptimality results for the LRT. A variety of examples, including testing in the orthant/circular cone, isotonic regression, Lasso and testing parametric assumptions versus shape-constrained alternatives, are worked out to demonstrate the versatility of the developed theory.
更多查看译文
关键词
Central limit theorem, isotonic regression, Lasso, normal approximation, power analysis, projection onto a closed convex set, second-order Poincare inequalities, shape constraint
AI 理解论文
溯源树
样例
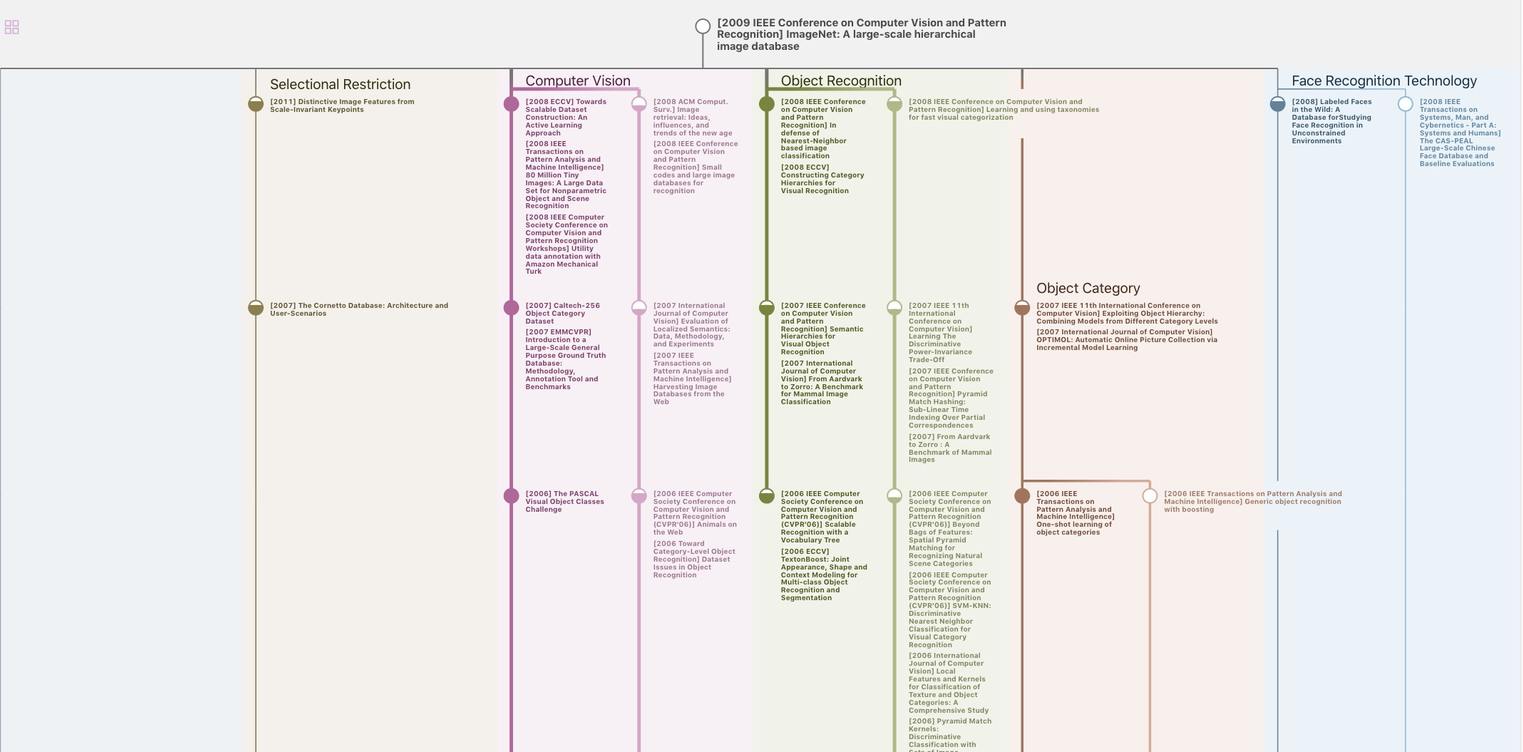
生成溯源树,研究论文发展脉络
Chat Paper
正在生成论文摘要