A machine learning method for automatic detection and classification of patient-ventilator asynchrony
2020 42nd Annual International Conference of the IEEE Engineering in Medicine & Biology Society (EMBC)(2020)
摘要
Patients suffering from respiratory failure are often put on assisted mechanical ventilation. Patient-ventilator asynchrony (PVA) can occur during mechanical ventilation, which cause damage to the lungs and has been linked to increased mortality in the intensive care unit. In current clinical practice PVA is still detected using visual inspection of the air pressure, flow, and volume curves, which is time-consuming and sensitive to subjective interpretation. Correct detection of the patient respiratory efforts is needed to properly asses the type of asynchrony. Therefore, we propose a method for automatic detection of the patient respiratory efforts using a one-dimensional convolution neural network. The proposed method was able to detect patient efforts with a sensitivity and precision of 98.6% and 97.3% for the inspiratory efforts, and 97.7% and 97.2% for the expiratory efforts. Besides allowing detection of PVA, combining the estimated timestamps of patient's inspiratory and expiratory efforts with the timings of the mechanical ventilator further allows for classification of the asynchrony type. In the future, the proposed method could support clinical decision making by informing clinicians on the quality of ventilation and providing actionable feedback for properly adjusting the ventilator settings.
更多查看译文
关键词
Humans,Intensive Care Units,Machine Learning,Respiration,Respiration, Artificial,Ventilators, Mechanical
AI 理解论文
溯源树
样例
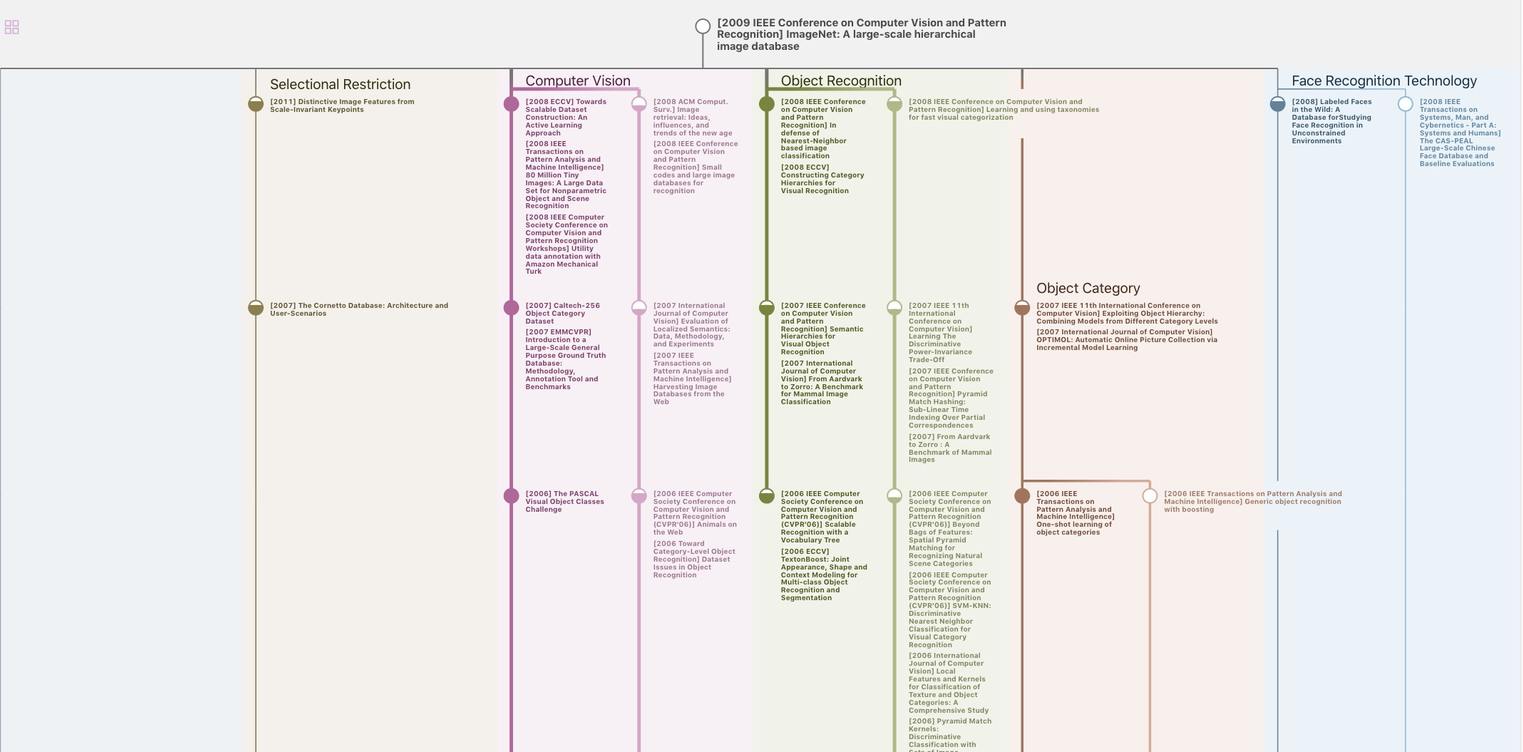
生成溯源树,研究论文发展脉络
Chat Paper
正在生成论文摘要