Participatory Research for Low-resourced Machine Translation: A Case Study in African Languages
EMNLP(2020)
摘要
Research in NLP lacks geographic diversity, and the question of how NLP can be scaled to low-resourced languages has not yet been adequately solved. "Low-resourced"-ness is a complex problem going beyond data availability and reflects systemic problems in society. In this paper, we focus on the task of Machine Translation (MT), that plays a crucial role for information accessibility and communication worldwide. Despite immense improvements in MT over the past decade, MT is centered around a few high-resourced languages. As MT researchers cannot solve the problem of low-resourcedness alone, we propose participatory research as a means to involve all necessary agents required in the MT development process. We demonstrate the feasibility and scalability of participatory research with a case study on MT for African languages. Its implementation leads to a collection of novel translation datasets, MT benchmarks for over 30 languages, with human evaluations for a third of them, and enables participants without formal training to make a unique scientific contribution. Benchmarks, models, data, code, and evaluation results are released under https://github.com/masakhane-io/masakhane-mt.
更多查看译文
关键词
machine translation,participatory research,african languages,low-resourced
AI 理解论文
溯源树
样例
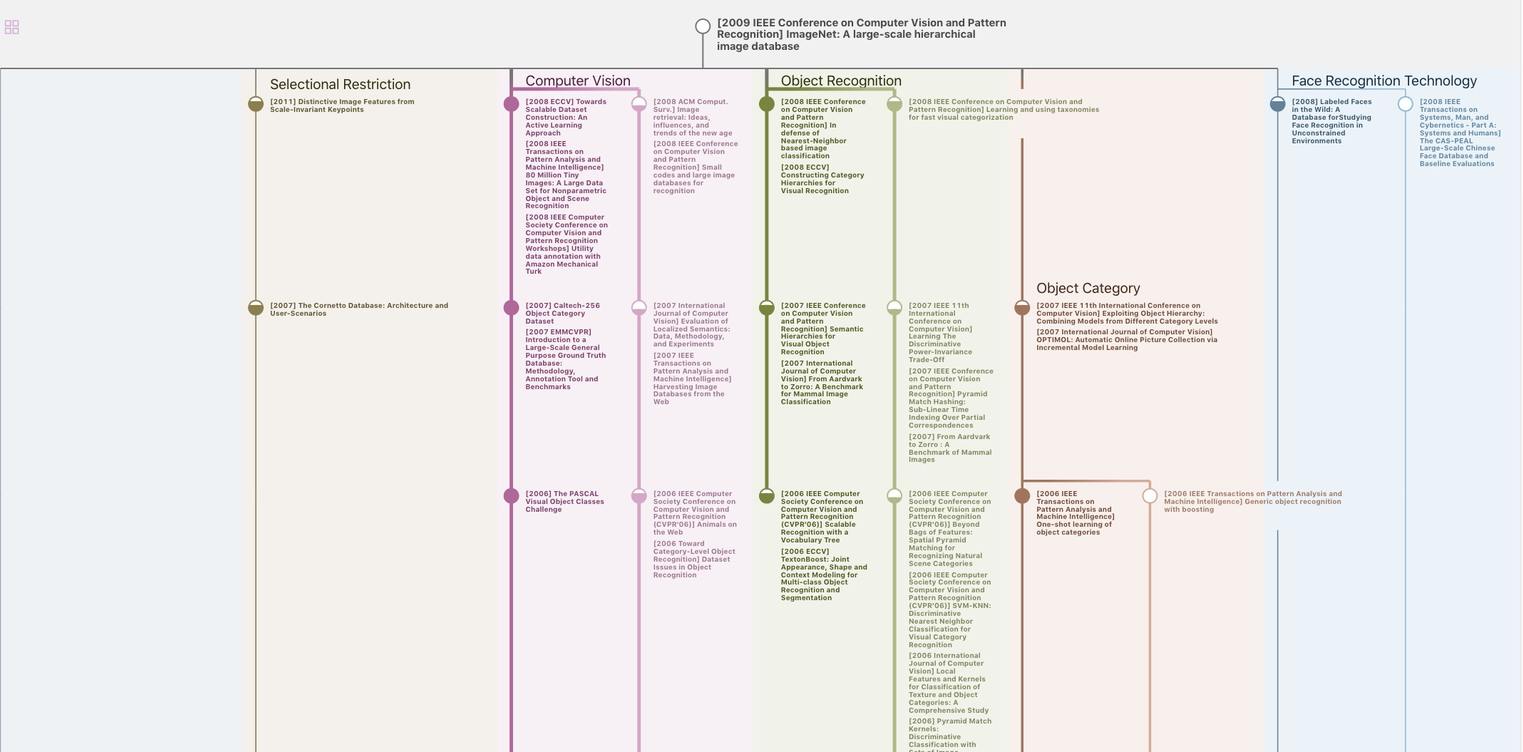
生成溯源树,研究论文发展脉络
Chat Paper
正在生成论文摘要