Processing Optimization and Property Predictions of Hot-Extruded Bi-Te-Se Thermoelectric Materials via Machine Learning
ADVANCED THEORY AND SIMULATIONS(2020)
摘要
Traditional experiment-based materials research is becoming increasingly insufficient to thoroughly understand materials' characteristics and thus it is becoming difficult to develop novel materials with better performance. Machine learning is applied to hot-extruded CuxBi2Te2.85+ySe0.15 thermoelectric materials, and the relationships between the processing, microstructure, and properties are further investigated via a data-driven approach. A properties-to-microstructure-to-processing inverse analysis is proposed and performed by a genetic algorithm. The analysis results indicate that hot-extruded materials have a potential best figure of merit (ZT) value of 1.15, which is 1.32 times larger than their best experimental value (0.87). To obtain this optimal property, processing variables such as higher extrusion temperature and larger Cu content and microstructure with a larger average grain size and higher density are required. The proposed data-driven approach is expected to provide a new avenue for designing high-performance Bi-Te-Se thermoelectric materials and thus to accelerate discoveries of novel materials.
更多查看译文
关键词
data-driven materials design,inverse analysis,machine learning,property prediction,thermoelectric materials
AI 理解论文
溯源树
样例
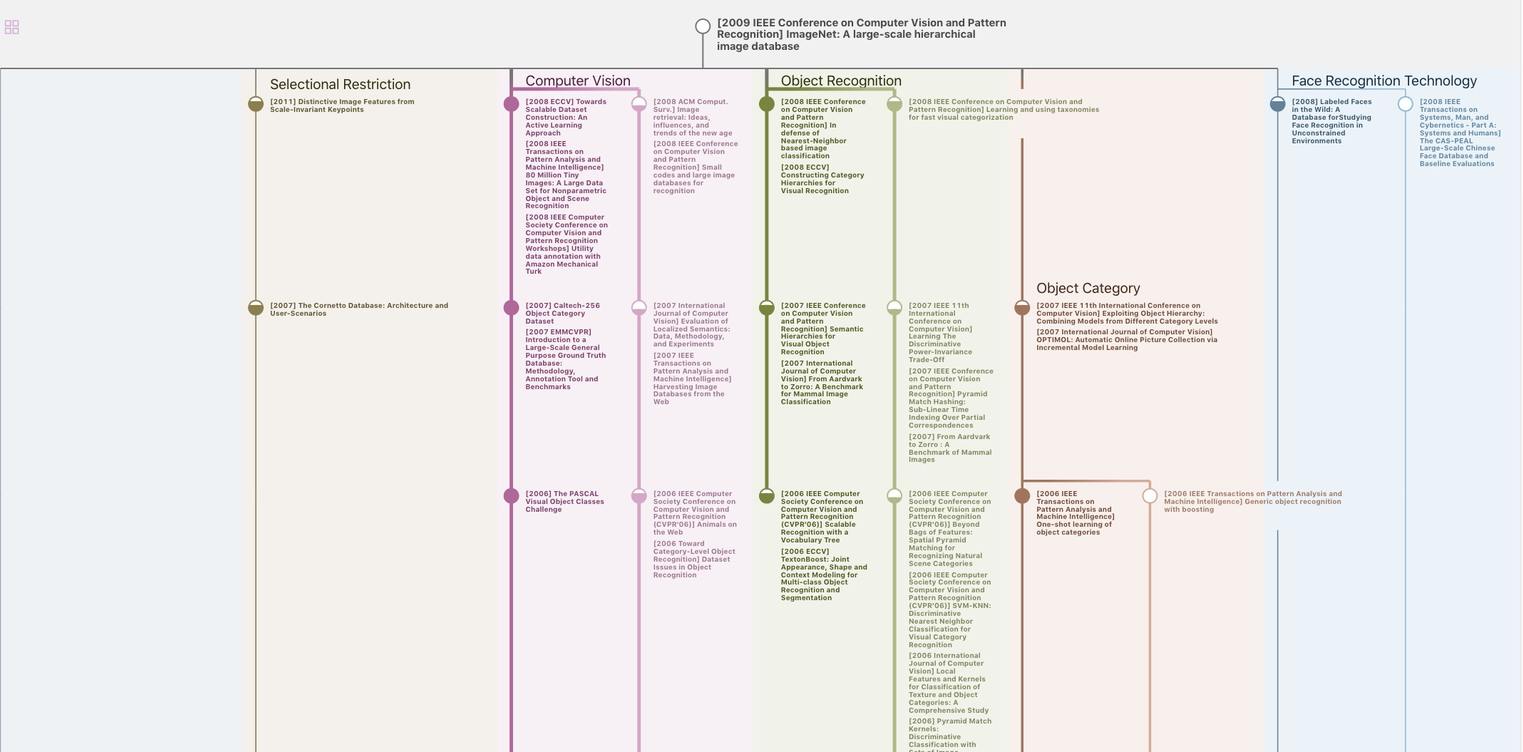
生成溯源树,研究论文发展脉络
Chat Paper
正在生成论文摘要