Data-Driven Fast Clustering of Second-Life Lithium-Ion Battery: Mechanism and Algorithm
ADVANCED THEORY AND SIMULATIONS(2020)
摘要
While electrical vehicles (EVs) are expanding rapidly and getting more and more popular in the market, researchers have started to leverage the remaining capacity of used or to-be-retired batteries for their second-life applications. It is crucial to develop a fast and efficient technology to first sort them and then extend their life while delivering energy, waste reduction, and economic benefits. In this work, a pulse clustering model embedded with improved bisecting K-means algorithm is developed to effectively sort retired batteries with life cycles ranging from new to an end-of-life state. The relevance of selected variables is rigorously validated, reaching the accuracy as high as 88% compared with the traditional full charge-discharge test. To note, the test time has largely reduced from hours to minutes. This data-driven clustering modeling with fast pulse test is a promising approach for clustering lithium-ion batteries, which is demonstrated with a home-built and high throughput intelligent clustering machine. In general, the technology opens a new generation of battery clustering, improving the efficiency and accuracy over the past semiempirical approaches.
更多查看译文
关键词
bisecting K-means algorithms,fast clustering,lithium-ion batteries,pulse tests,retired batteries
AI 理解论文
溯源树
样例
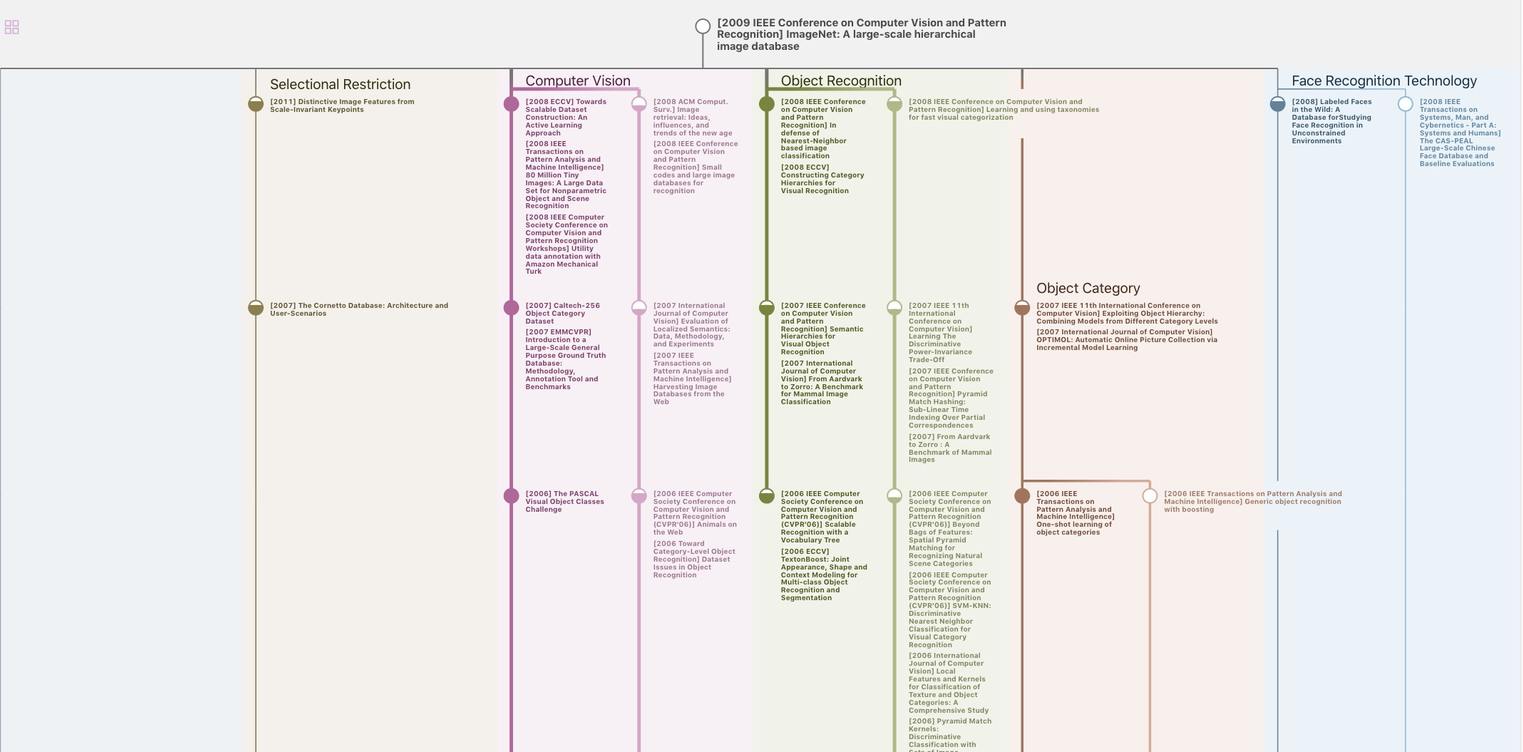
生成溯源树,研究论文发展脉络
Chat Paper
正在生成论文摘要