A Fully Hyperbolic Neural Model for Hierarchical Multi-Class Classification
EMNLP(2020)
摘要
Label inventories for fine-grained entity typing have grown in size and complexity. Nonetheless, they exhibit a hierarchical structure. Hyperbolic spaces offer a mathematically appealing approach for learning hierarchical representations of symbolic data. However, it is not clear how to integrate hyperbolic components into downstream tasks. This is the first work that proposes a fully hyperbolic model for multi-class multi-label classification, which performs all operations in hyperbolic space. We evaluate the proposed model on two challenging datasets and compare to different baselines that operate under Euclidean assumptions. Our hyperbolic model infers the latent hierarchy from the class distribution, captures implicit hyponymic relations in the inventory, and shows performance on par with state-of-the-art methods on fine-grained classification with remarkable reduction of the parameter size. A thorough analysis sheds light on the impact of each component in the final prediction and showcases its ease of integration with Euclidean layers.
更多查看译文
关键词
hyperbolic neural model,classification,multi-class
AI 理解论文
溯源树
样例
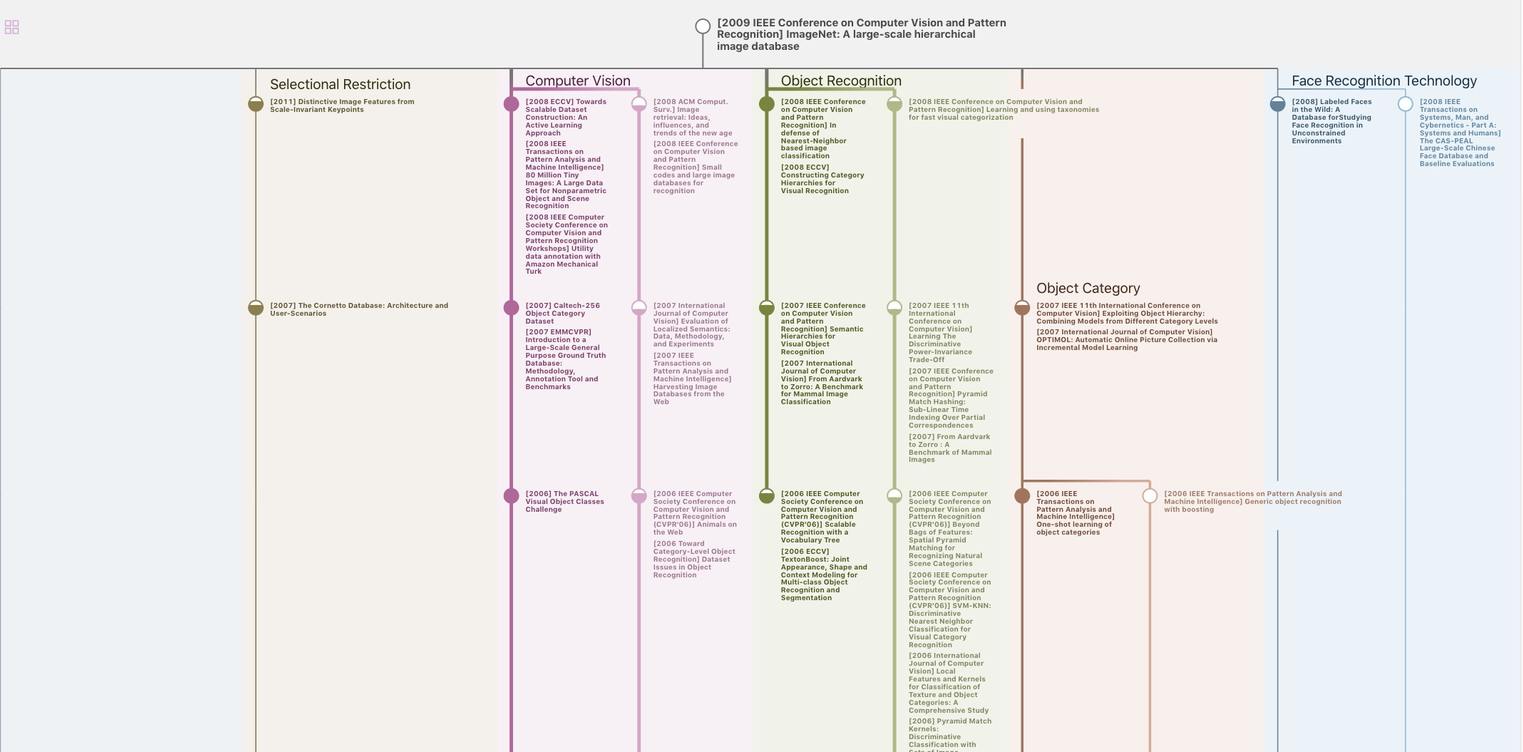
生成溯源树,研究论文发展脉络
Chat Paper
正在生成论文摘要