GAN-based Defect Synthesis for Anomaly Detection in Fabrics
2020 25th IEEE International Conference on Emerging Technologies and Factory Automation (ETFA)(2020)
摘要
Image-based quality control aims at detecting anomalies in products and is a crucial part of the production process. Challenges arise from the complexity and variety of products, defects, and the rarity of defect occurrence. Quality control thus still relies heavily on manual inspection. Supervised, data driven approaches have greatly improved performance, but suffer from a major drawback: They require large amounts of annotated training data, limiting their economic viability.In this work, we overcome this drawback by leveraging the consistency of defect appearance across fabrics to transfer knowledge about anomalies from one fabric to another. We realize this by adapting the image-to-image translation framework, introducing guidance by means of a segmentation map. We evaluate both image quality as well as usability of generated defects. For the image quality, both classical (L1, L2 and Structured Similarity Measurement (SSIM)) and perceptual (Learned Perceptual Image Patch Similarity (LPIPS)) metrics indicate efficacy of the presented approach, i.e. defect synthesis only occurs in the targeted regions, and the background fabric pattern remains largely unchanged. To evaluate usability of generated defects, we train pseudo-supervised models on synthesized defects of fabrics unseen during training. A comparison with semi-supervised, autoencoder based approaches demonstrates the suitability of our approach, yielding average Area Under the Receiver Operating Characteristic Curve (AUROC) values of 0.81 for pseudo-supervised vs. 0.69 for semi-supervised settings.
更多查看译文
AI 理解论文
溯源树
样例
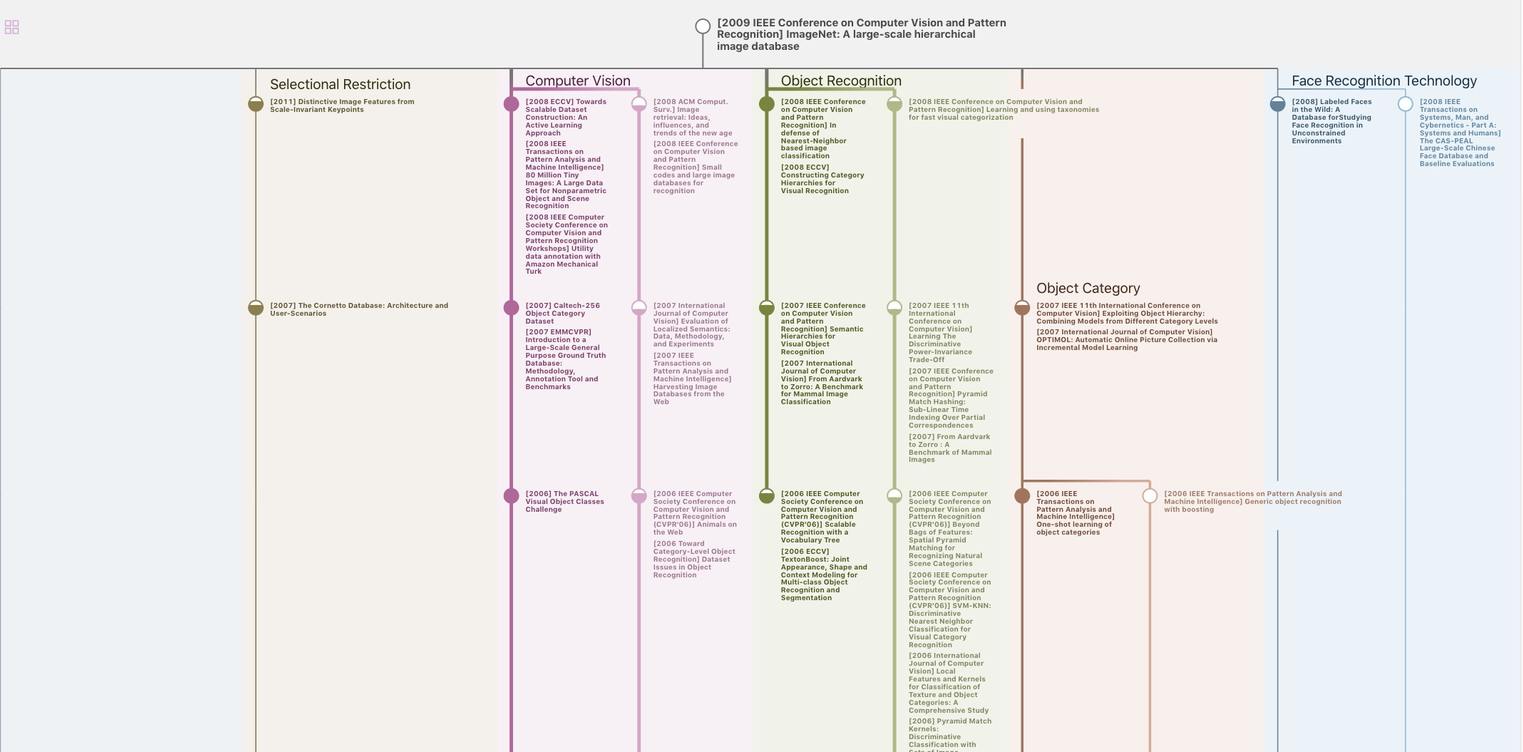
生成溯源树,研究论文发展脉络
Chat Paper
正在生成论文摘要