Pruning Redundant Mappings in Transformer Models via Spectral-Normalized Identity Prior
EMNLP(2020)
摘要
Traditional (unstructured) pruning methods for a Transformer model focus on regularizing the individual weights by penalizing them toward zero. In this work, we explore spectral-normalized identity priors (SNIP), a structured pruning approach that penalizes an entire residual module in a Transformer model toward an identity mapping. Our method identifies and discards unimportant non-linear mappings in the residual connections by applying a thresholding operator on the function norm. It is applicable to any structured module, including a single attention head, an entire attention block, or a feed-forward subnetwork. Furthermore, we introduce spectral normalization to stabilize the distribution of the post-activation values of the Transformer layers, further improving the pruning effectiveness of the proposed methodology. We conduct experiments with BERT on 5 GLUE benchmark tasks to demonstrate that SNIP achieves effective pruning results while maintaining comparable performance. Specifically, we improve the performance over the state-of-the-art by 0.5 to 1.0% on average at 50% compression ratio.
更多查看译文
关键词
transformer models,mappings,spectral-normalized
AI 理解论文
溯源树
样例
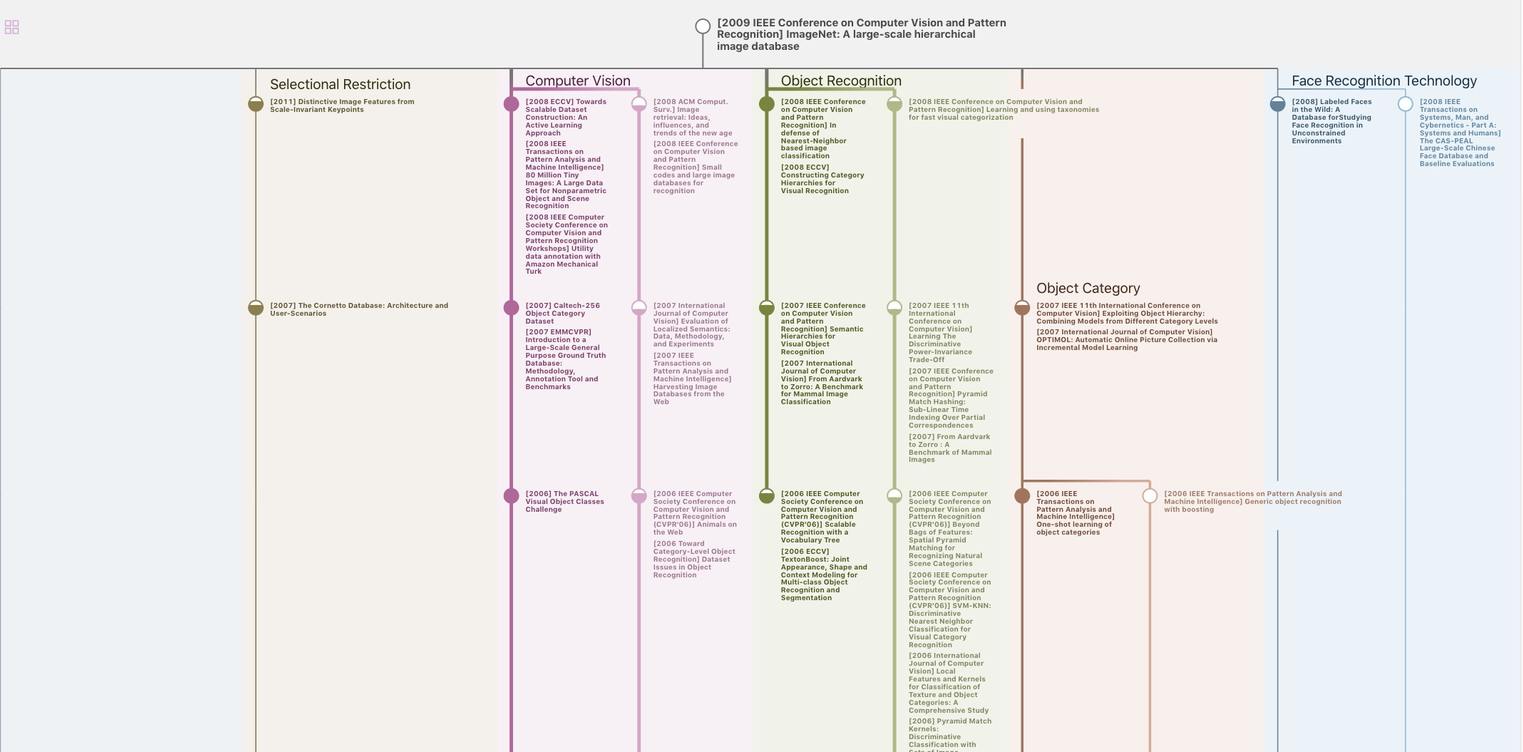
生成溯源树,研究论文发展脉络
Chat Paper
正在生成论文摘要