The act of remembering: a study in partially observable reinforcement learning
arxiv(2020)
摘要
Reinforcement Learning (RL) agents typically learn memoryless policies---policies that only consider the last observation when selecting actions. Learning memoryless policies is efficient and optimal in fully observable environments. However, some form of memory is necessary when RL agents are faced with partial observability. In this paper, we study a lightweight approach to tackle partial observability in RL. We provide the agent with an external memory and additional actions to control what, if anything, is written to the memory. At every step, the current memory state is part of the agent's observation, and the agent selects a tuple of actions: one action that modifies the environment and another that modifies the memory. When the external memory is sufficiently expressive, optimal memoryless policies yield globally optimal solutions. Unfortunately, previous attempts to use external memory in the form of binary memory have produced poor results in practice. Here, we investigate alternative forms of memory in support of learning effective memoryless policies. Our novel forms of memory outperform binary and LSTM-based memory in well-established partially observable domains.
更多查看译文
关键词
observable reinforcement
AI 理解论文
溯源树
样例
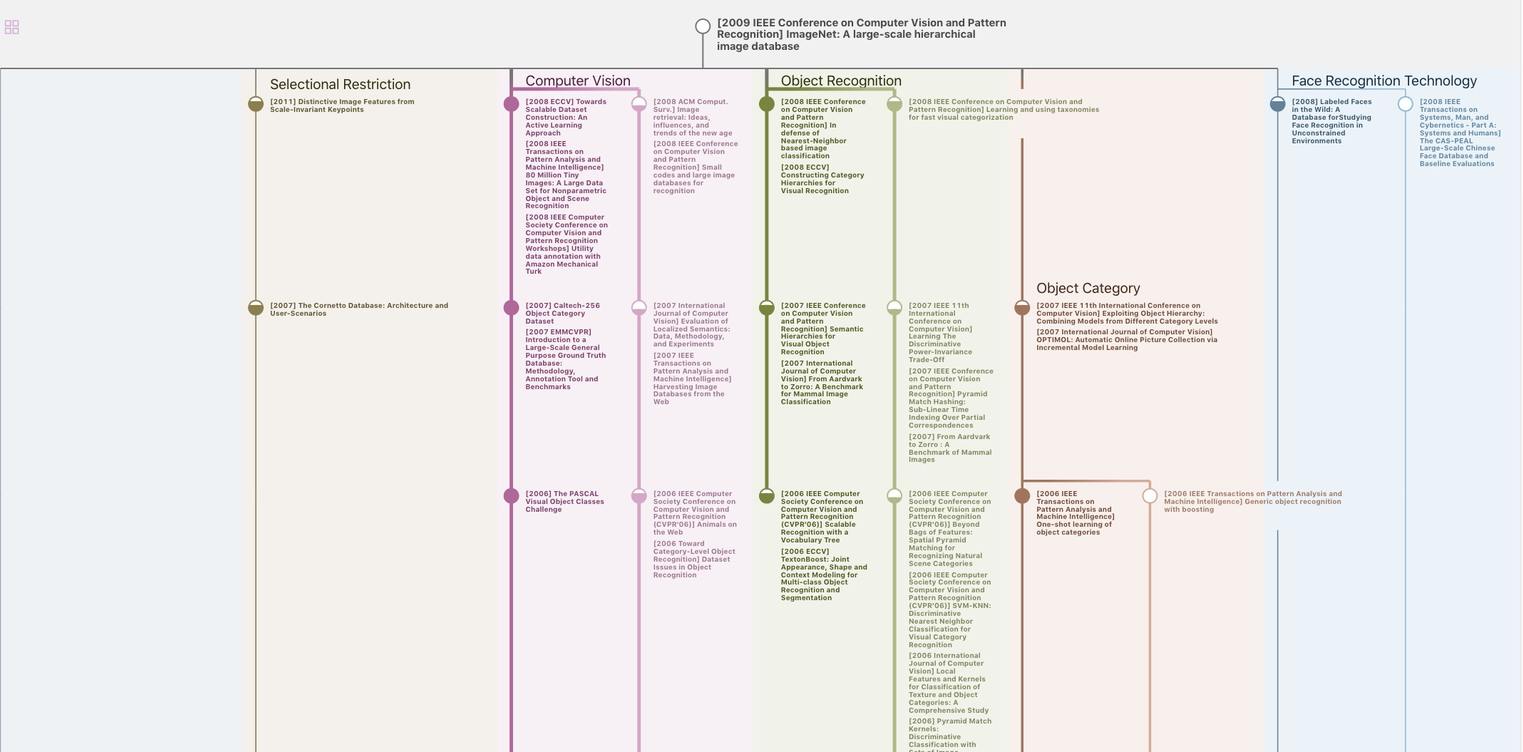
生成溯源树,研究论文发展脉络
Chat Paper
正在生成论文摘要