Reinforcement Learning Of Sequential Price Mechanisms
arxiv(2021)
摘要
We introduce the use of reinforcement learning for indirect mechanisms, working with the existing class of sequential price mechanisms, which generalizes both serial dictatorship and posted price mechanisms and essentially characterizes all strongly obviously strategyproof mechanisms. Learning an optimal mechanism within this class forms a partially-observable Markov decision process. We provide rigorous conditions for when this class of mechanisms is more powerful than simpler static mechanisms, for sufficiency or insufficiency of observation statistics for learning, and for the necessity of complex (deep) policies. We show that our approach can learn optimal or near-optimal mechanisms in several experimental settings.
更多查看译文
关键词
reinforcement learning,price,mechanisms
AI 理解论文
溯源树
样例
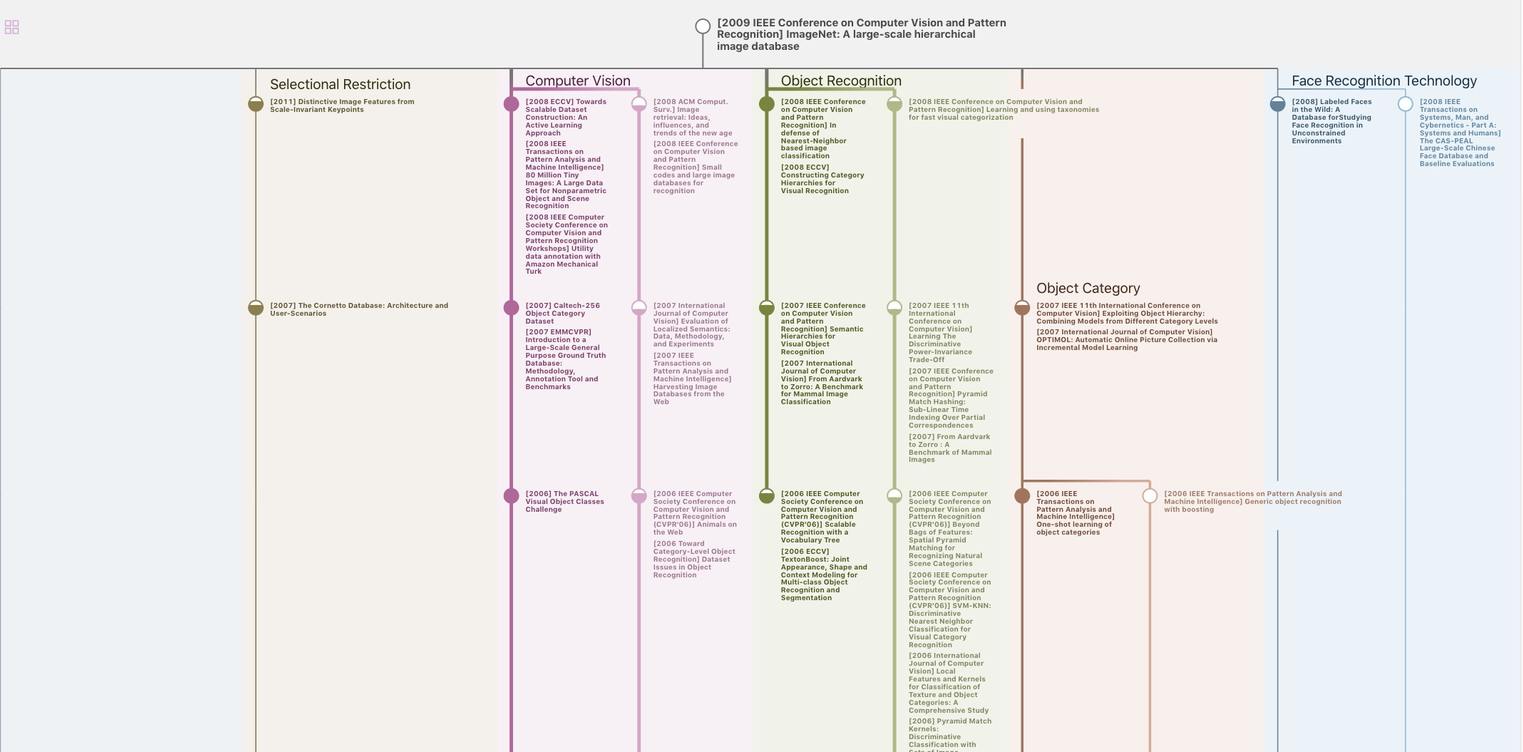
生成溯源树,研究论文发展脉络
Chat Paper
正在生成论文摘要