Exploration in Approximate Hyper-State Space for Meta Reinforcement Learning
ICML(2021)
摘要
Meta-learning is a powerful tool for learning policies that can adapt efficiently when deployed in new tasks. If however the meta-training tasks have sparse rewards, the need for exploration during meta-training is exacerbated given that the agent has to explore and learn across many tasks. We show that current meta-learning methods can fail catastrophically in such environments. To address this problem, we propose HyperX, a novel method for meta-learning in sparse reward tasks. Using novel reward bonuses for meta-training, we incentivise the agent to explore in approximate hyper-state space, i.e., the joint state and approximate belief space, where the beliefs are over tasks. We show empirically that these bonuses allow an agent to successfully learn to solve sparse reward tasks where existing meta-learning methods fail.
更多查看译文
AI 理解论文
溯源树
样例
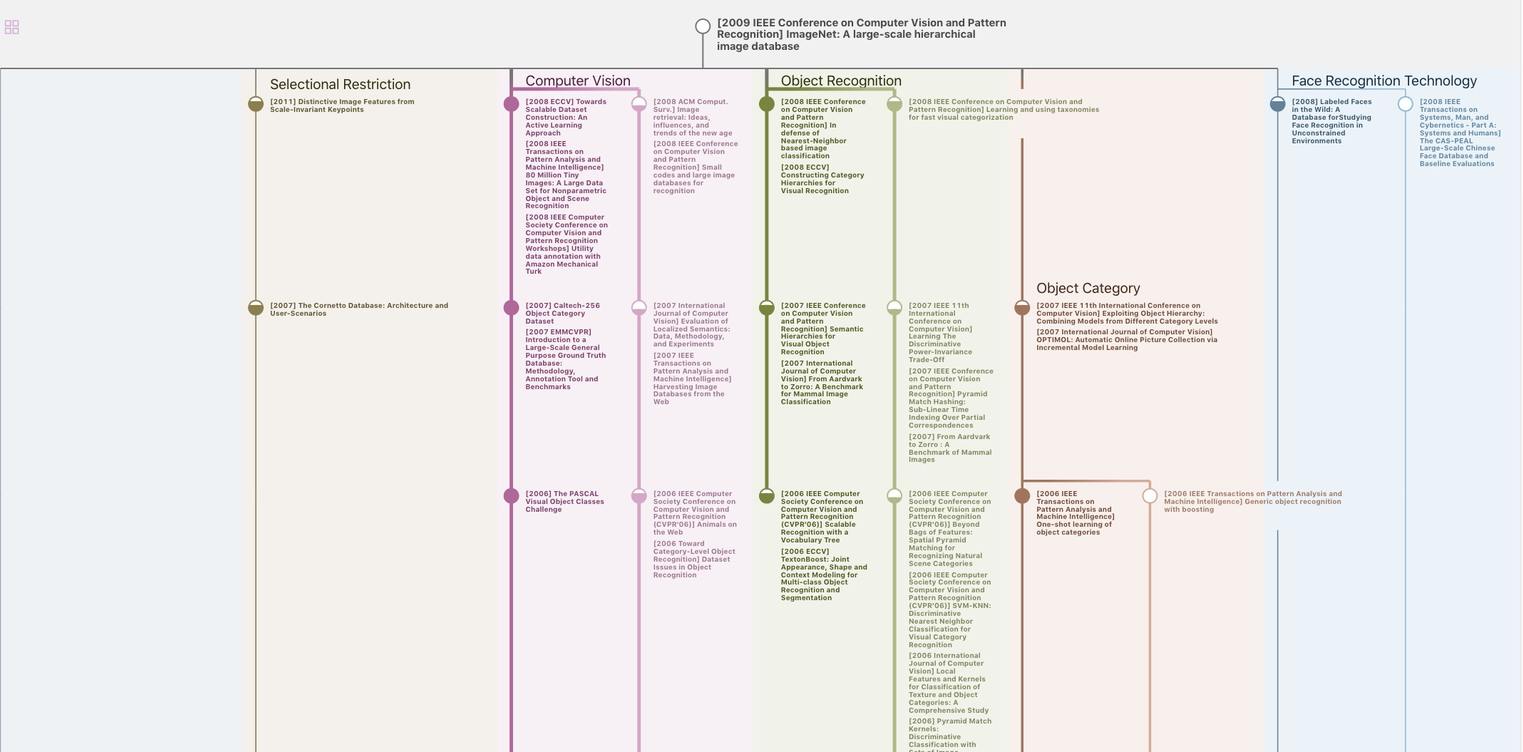
生成溯源树,研究论文发展脉络
Chat Paper
正在生成论文摘要