RDCNet: Instance segmentation with a minimalist recurrent residual network
MLMI@MICCAI(2020)
摘要
Instance segmentation is a key step for quantitative microscopy. While several machine learning based methods have been proposed for this problem, most of them rely on computationally complex models that are trained on surrogate tasks. Building on recent developments towards end-to-end trainable instance segmentation, we propose a minimalist recurrent network called recurrent dilated convolutional network (RDCNet), consisting of a shared stacked dilated convolution (sSDC) layer that iteratively refines its output and thereby generates interpretable intermediate predictions. It is light-weight and has few critical hyperparameters, which can be related to physical aspects such as object size or density.We perform a sensitivity analysis of its main parameters and we demonstrate its versatility on 3 tasks with different imaging modalities: nuclear segmentation of H&E slides, of 3D anisotropic stacks from light-sheet fluorescence microscopy and leaf segmentation of top-view images of plants. It achieves state-of-the-art on 2 of the 3 datasets.
更多查看译文
关键词
instance segmentation
AI 理解论文
溯源树
样例
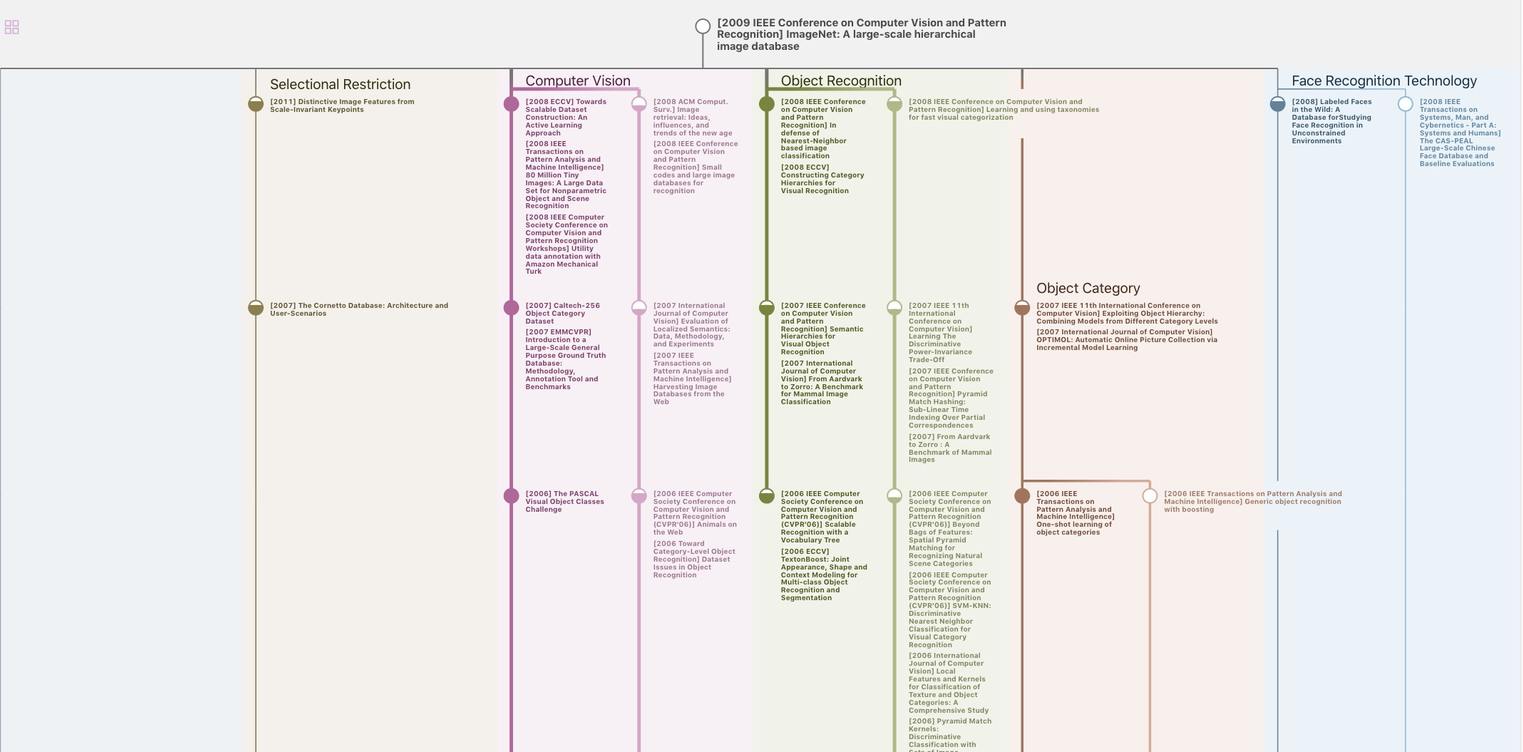
生成溯源树,研究论文发展脉络
Chat Paper
正在生成论文摘要