Continual Learning for Natural Language Generation in Task-oriented Dialog Systems
EMNLP(2020)
摘要
Natural language generation (NLG) is an essential component of task-oriented dialog systems. Despite the recent success of neural approaches for NLG, they are typically developed in an offline manner for particular domains. To better fit real-life applications where new data come in a stream, we study NLG in a "continual learning" setting to expand its knowledge to new domains or functionalities incrementally. The major challenge towards this goal is catastrophic forgetting, meaning that a continually trained model tends to forget the knowledge it has learned before. To this end, we propose a method called ARPER (Adaptively Regularized Prioritized Exemplar Replay) by replaying prioritized historical exemplars, together with an adaptive regularization technique based on ElasticWeight Consolidation. Extensive experiments to continually learn new domains and intents are conducted on MultiWoZ-2.0 to benchmark ARPER with a wide range of techniques. Empirical results demonstrate that ARPER significantly outperforms other methods by effectively mitigating the detrimental catastrophic forgetting issue.
更多查看译文
关键词
dialog systems,natural language generation,learning,task-oriented
AI 理解论文
溯源树
样例
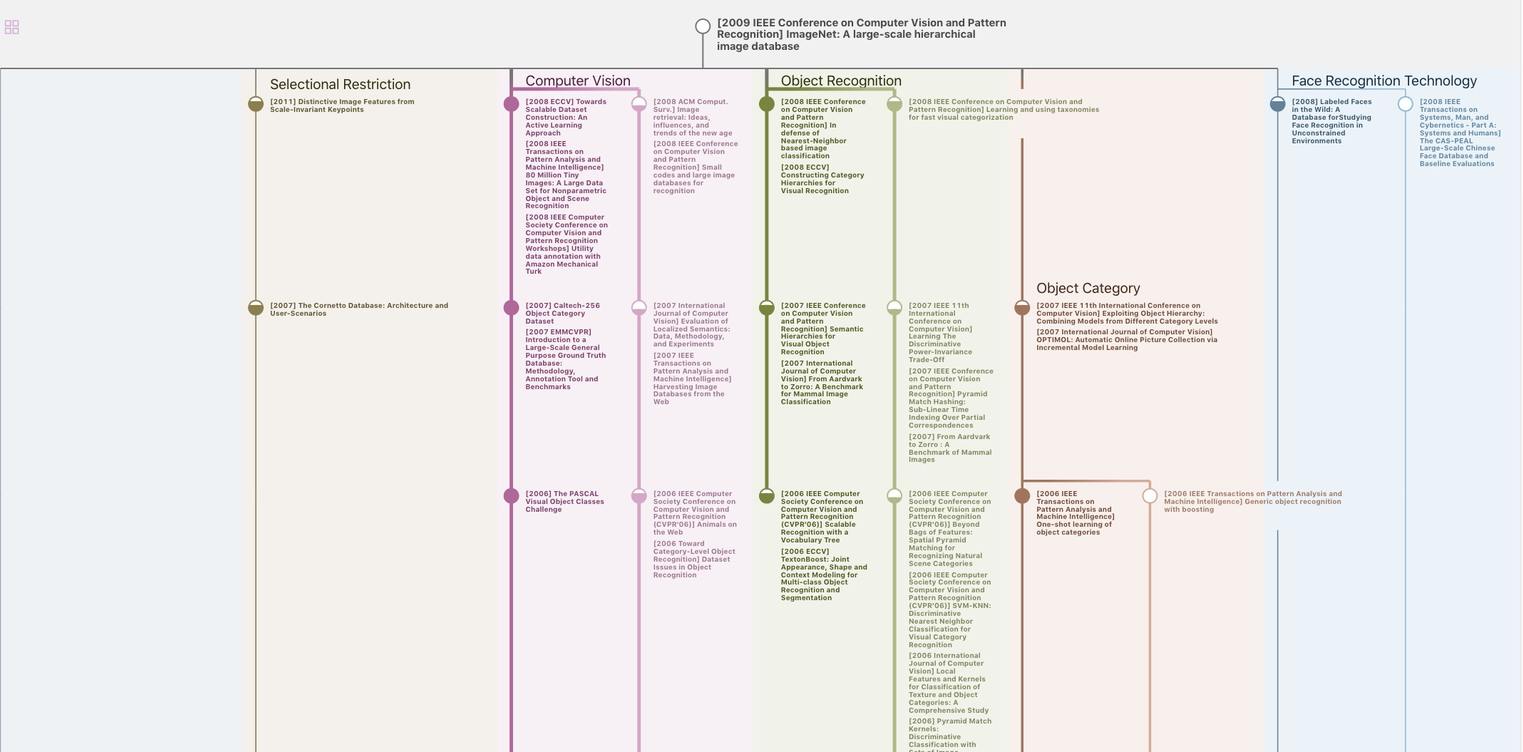
生成溯源树,研究论文发展脉络
Chat Paper
正在生成论文摘要