Super-Resolution And Denoising Of 4d-Flow Mri Using Physics-Informed Deep Neural Nets
COMPUTER METHODS AND PROGRAMS IN BIOMEDICINE(2020)
摘要
Background and Objective: Time resolved three-dimensional phase contrast magnetic resonance imaging (4D-Flow MRI) has been used to non-invasively measure blood velocities in the human vascular system. However, issues such as low spatio-temporal resolution, acquisition noise, velocity aliasing, and phaseoffset artifacts have hampered its clinical application. In this research, we developed a purely data-driven method for super-resolution and denoising of 4D-Flow MRI.Methods: The flow velocities, pressure, and the MRI image magnitude are modeled as a patient-specific deep neural net (DNN). For training, 4D-Flow MRI images in the complex Cartesian space are used to impose data-fidelity. Physics of fluid flow is imposed through regularization. Creative loss function terms have been introduced to handle noise and super-resolution. The trained patient-specific DNN can be sampled to generate noise-free high-resolution flow images. The proposed method has been implemented using the TensorFlow DNN library and tested on numerical phantoms and validated in-vitro using high resolution particle image velocitmetry (PIV) and 4D-Flow MRI experiments on transparent models subjected to pulsatile flow conditions.Results: In case of numerical phantoms, we were able to increase spatial resolution by a factor of 100 and temporal resolution by a factor of 5 compared to the simulated 4D-Flow MRI. There is an order of magnitude reduction of velocity normalized root mean square error (vNRMSE). In case of the in-vitro validation tests with PIV as reference, there is similar improvement in spatio-temporal resolution. Although the vNRMSE is reduced by 50%, the method is unable to negate a systematic bias with respect to the reference PIV that is introduced by the 4D-Flow MRI measurement.Conclusions: This work has demonstrated the feasibility of using the readily available machinery of deep learning to enhance 4D-Flow MRI using a purely data-driven method. Unlike current state-of-the-art methods, the proposed method is agnostic to geometry and boundary conditions and therefore eliminates the need for tedious tasks such as accurate image segmentation for geometry, image registration, and estimation of boundary flow conditions. Arbitrary regions of interest can be selected for processing. This work will lead to user-friendly analysis tools that will enable quantitative hemodynamic analysis of vascular diseases in a clinical setting. (C) 2020 Published by Elsevier B.V.
更多查看译文
关键词
PI-DNN, 4D-Flow MRI, Data assimilation, Super-resolution, Denoising, Validation
AI 理解论文
溯源树
样例
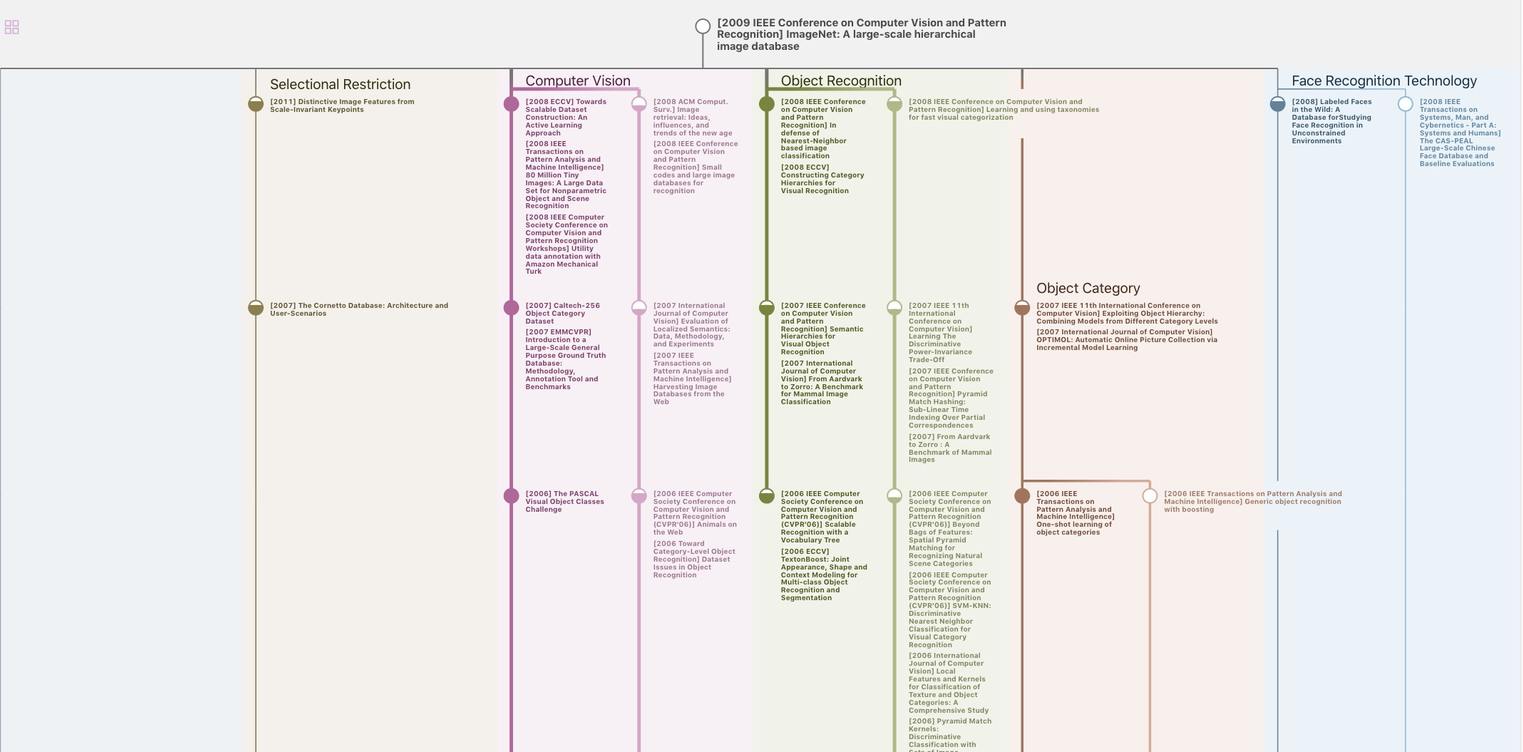
生成溯源树,研究论文发展脉络
Chat Paper
正在生成论文摘要