Mitigating Gender Bias for Neural Dialogue Generation with Adversarial Learning
Conference on Empirical Methods in Natural Language Processing(2020)
摘要
Dialogue systems play an increasingly important role in various aspects of our daily life. It is evident from recent research that dialogue systems trained on human conversation data are biased. In particular, they can produce responses that reflect people’s gender prejudice. Many debiasing methods have been developed for various NLP tasks, such as word embedding. However, they are not directly applicable to dialogue systems because they are likely to force dialogue models to generate similar responses for different genders. This greatly degrades the diversity of the generated responses and immensely hurts the performance of the dialogue models. In this paper, we propose a novel adversarial learning framework Debiased-Chat to train dialogue models free from gender bias while keeping their performance. Extensive experiments on two real-world conversation datasets show that our framework significantly reduces gender bias in dialogue models while maintaining the response quality.
更多查看译文
关键词
neural dialogue generation,adversarial
AI 理解论文
溯源树
样例
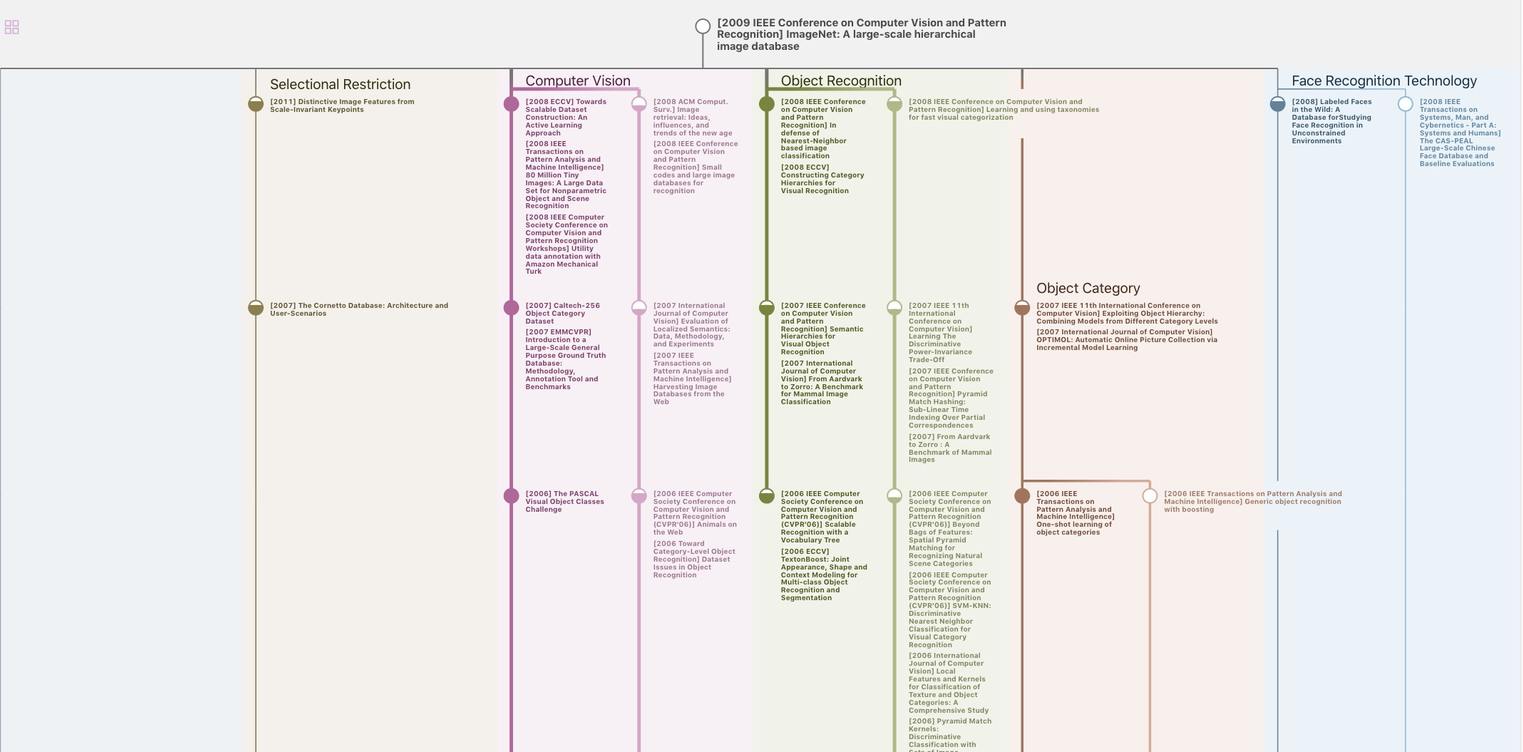
生成溯源树,研究论文发展脉络
Chat Paper
正在生成论文摘要