Inductive Graph Embeddings through Locality Encodings
arxiv(2020)
摘要
Learning embeddings from large-scale networks is an open challenge. Despite the overwhelming number of existing methods, is is unclear how to exploit network structure in a way that generalizes easily to unseen nodes, edges or graphs. In this work, we look at the problem of finding inductive network embeddings in large networks without domain-dependent node/edge attributes. We propose to use a set of basic predefined local encodings as the basis of a learning algorithm. In particular, we consider the degree frequencies at different distances from a node, which can be computed efficiently for relatively short distances and a large number of nodes. Interestingly, the resulting embeddings generalize well across unseen or distant regions in the network, both in unsupervised settings, when combined with language model learning, as well as in supervised tasks, when used as additional features in a neural network. Despite its simplicity, this method achieves state-of-the-art performance in tasks such as role detection, link prediction and node classification, and represents an inductive network embedding method directly applicable to large unattributed networks.
更多查看译文
关键词
locality encodings
AI 理解论文
溯源树
样例
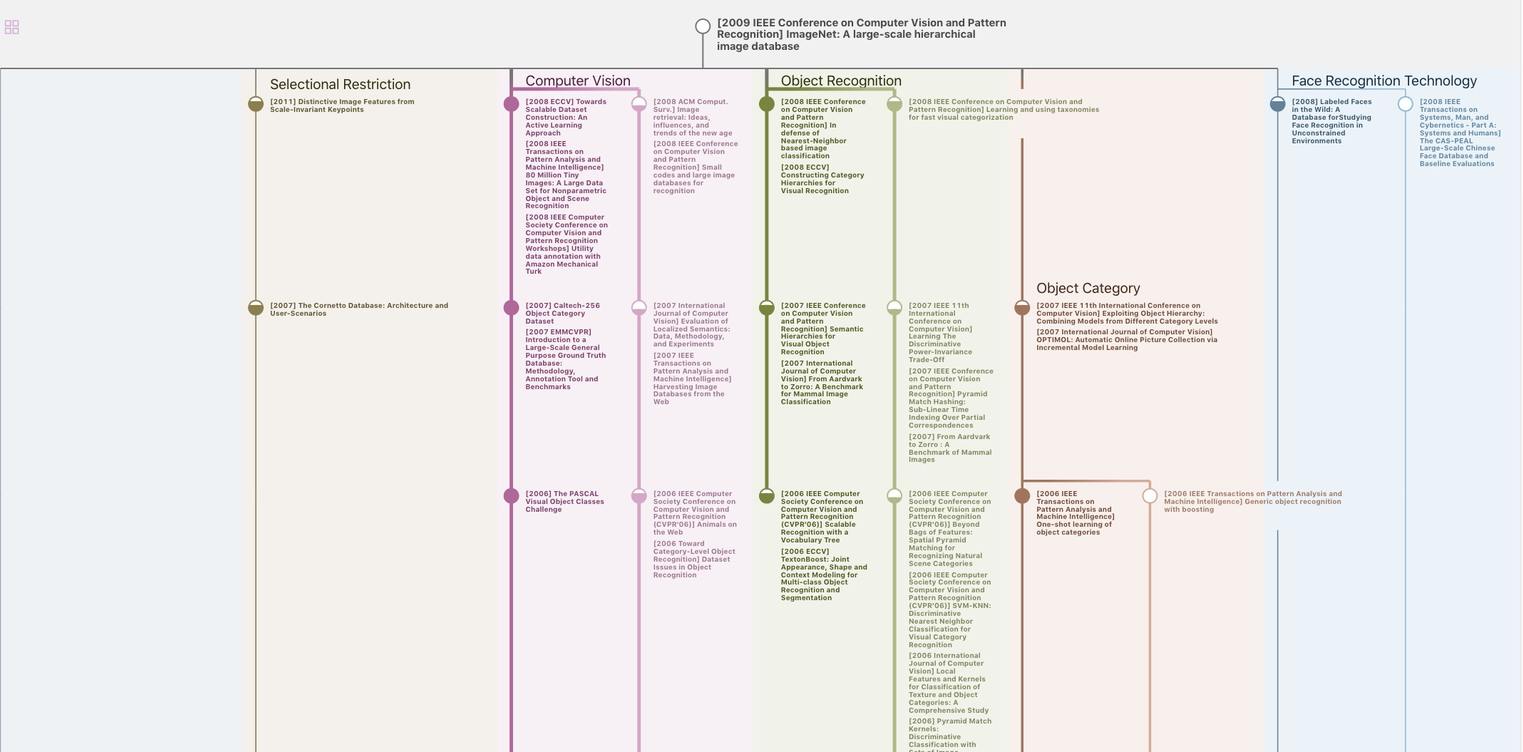
生成溯源树,研究论文发展脉络
Chat Paper
正在生成论文摘要