Soft Rotation Equivariant Convolutional Neural Networks
2020 International Joint Conference on Neural Networks (IJCNN)(2020)
摘要
A key to the generalization ability of Convolutional Neural Networks (CNNs) is the idea that patterns that appear in one region of the image have a high probability of appearing in other regions. This notion is also true for other spatial relationships, such as orientation. Motivated by the fact that in the early layers of CNNs distinct filters often encode for the same feature at different angles, we propose to incorporate the rotation equivariant prior in these models. In this work, different regularization strategies that capture the notion of approximate equivariance were designed and quantitatively evaluated in their ability to generate rotation-equivariant models and their effect on the model’s capacity to generalize to unseen data. Some of these strategies consistently lead to higher test set accuracies when compared to a baseline model, on classification tasks. We conclude that the rotation equivariance prior should be adopted in the general setting when modeling visual data.
更多查看译文
关键词
Feature extraction,Task analysis,Convolution,Visualization,Convolutional neural networks,Data models,Machine learning
AI 理解论文
溯源树
样例
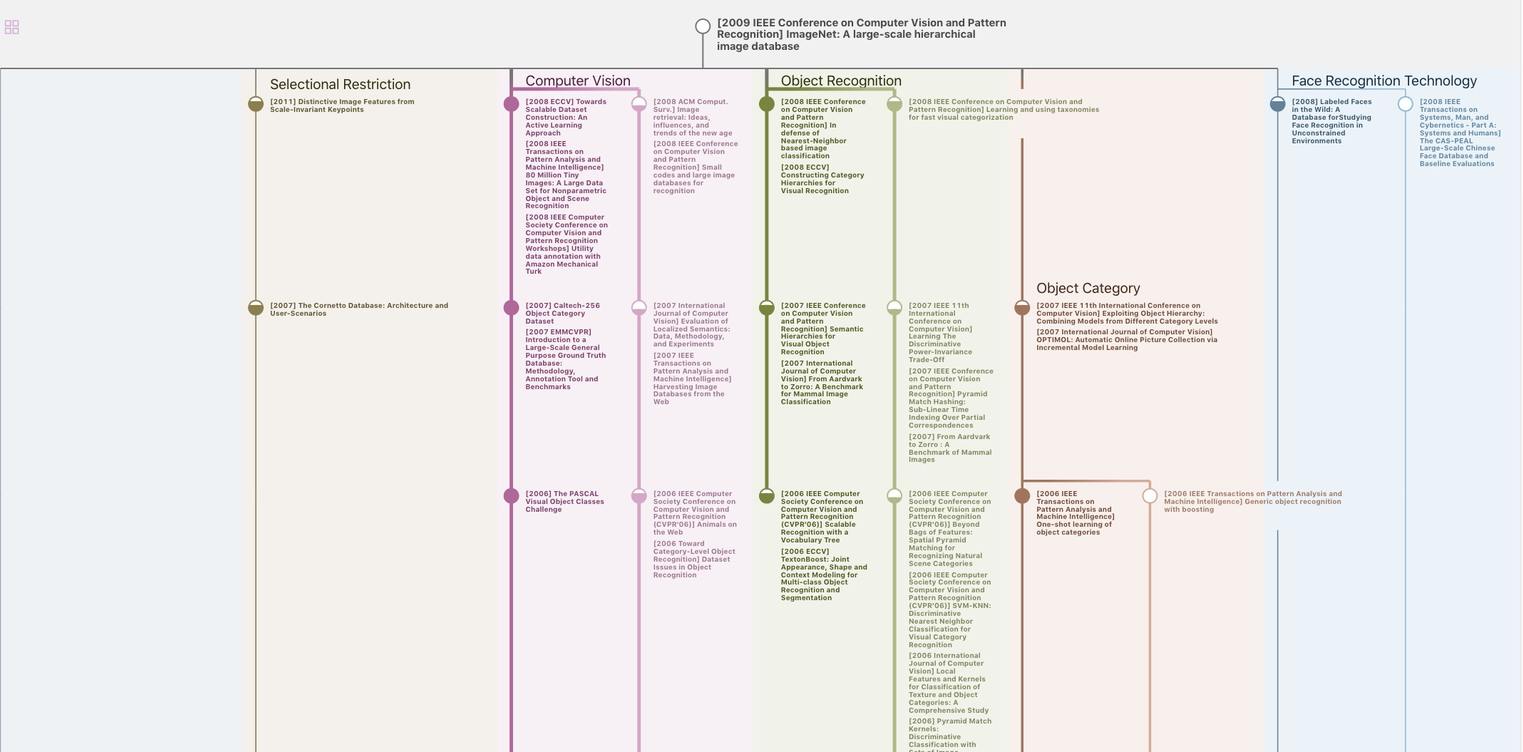
生成溯源树,研究论文发展脉络
Chat Paper
正在生成论文摘要