daVinciNet: Joint Prediction of Motion and Surgical State in Robot-Assisted Surgery
IROS(2020)
摘要
This paper presents a technique to concurrently and jointly predict the future trajectories of surgical instruments and the future state(s) of surgical subtasks in robot-assisted surgeries (RAS) using multiple input sources. Such predictions are a necessary first step towards shared control and supervised autonomy of surgical subtasks. Minute-long surgical subtasks, such as suturing or ultrasound scanning, often have distinguishable tool kinematics and visual features, and can be described as a series of fine-grained states with transition schematics. We propose daVinciNet - an end-to-end dual-task model for robot motion and surgical state predictions. daVinciNet performs concurrent end-effector trajectory and surgical state predictions using features extracted from multiple data streams, including robot kinematics, endoscopic vision, and system events. We evaluate our proposed model on an extended Robotic Intra-Operative Ultrasound (RIOUS+) imaging dataset collected on a da Vinci Xi surgical system and the JHU-ISI Gesture and Skill Assessment Working Set (JIGSAWS). Our model achieves up to 93.85% short-term (0.5s) and 82.11% long-term (2s) state prediction accuracy, as well as 1.07mm short-term and 5.62mm long-term trajectory prediction error.
更多查看译文
关键词
long-term trajectory prediction error,robot-assisted surgery,surgical instruments,multiple input sources,surgical subtasks,fine-grained states,end-to-end dual-task model,robot motion,concurrent end-effector trajectory,robot kinematics,da Vinci Xi surgical system,extended robotic intraoperative ultrasound imaging dataset,daVinciNet,endoscopic vision,JHU-ISI-gesture-and-skill-assessment-working-set,JIGSAWS,supervised autonomy,feature extraction,short-term trajectory prediction error
AI 理解论文
溯源树
样例
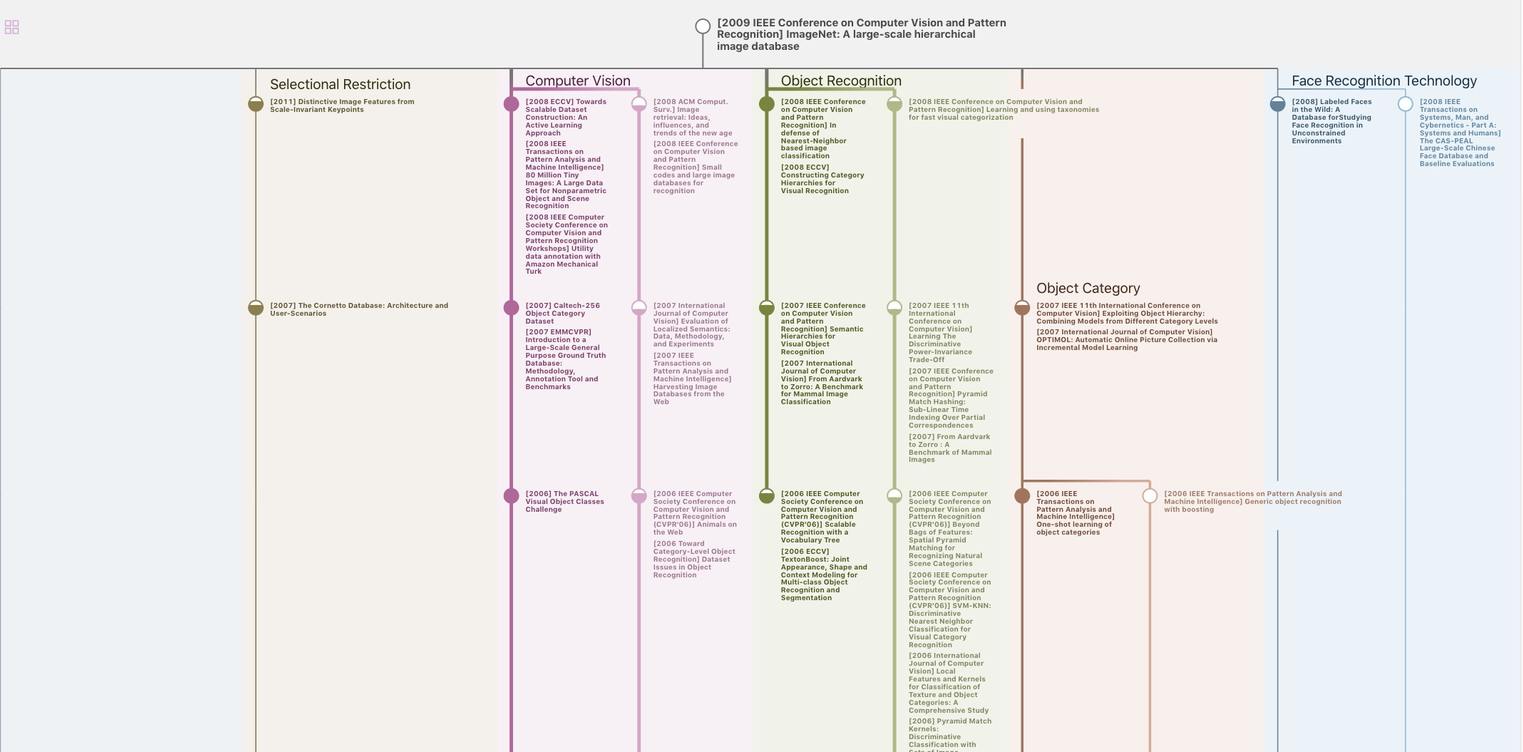
生成溯源树,研究论文发展脉络
Chat Paper
正在生成论文摘要