Group structure detection for a high-dimensional panel data model
CANADIAN JOURNAL OF STATISTICS-REVUE CANADIENNE DE STATISTIQUE(2022)
摘要
This article investigates latent group structures in a high-dimensional panel data model. It is assumed that subjects are classified into unobserved groups, where the fixed effects for each subject are the same within a group but heterogeneous across different groups. A penalized regression approach is proposed to identify latent group structures and significant covariates simultaneously. A new algorithm is proposed to optimize the objective function. When the sample size goes to infinity, it is shown that the proposed estimator recovers latent group structures and important covariates with probability approaching one. The finite sample performance of the proposed estimator is evaluated through Monte Carlo studies and illustrated using a real dataset.
更多查看译文
关键词
Panel data, penalized estimator, subgroup analysis, ultra-high-dimensional data
AI 理解论文
溯源树
样例
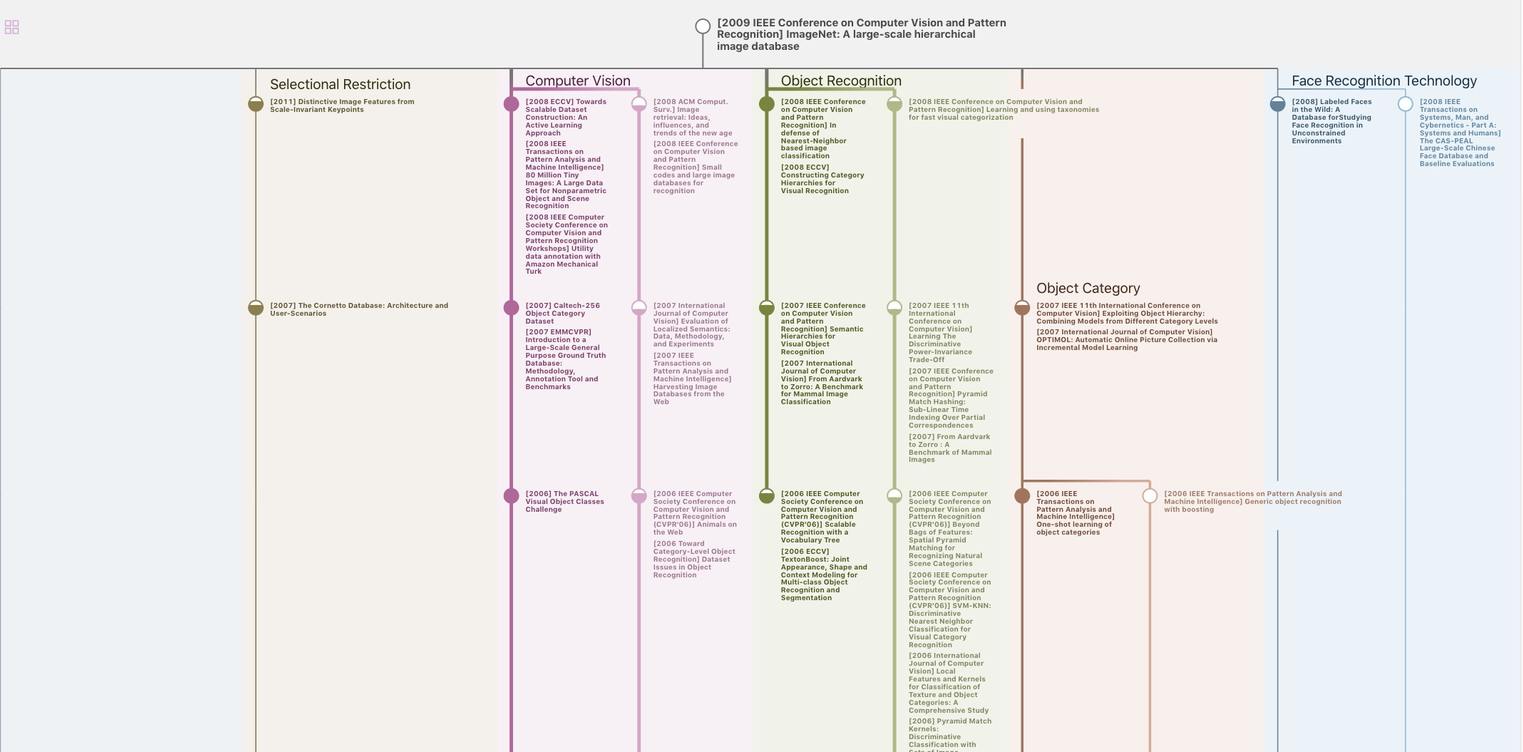
生成溯源树,研究论文发展脉络
Chat Paper
正在生成论文摘要