On Aggregation Of Unsupervised Deep Binary Descriptor With Weak Bits
IEEE TRANSACTIONS ON IMAGE PROCESSING(2020)
摘要
Despite the thrilling success achieved by existing binary descriptors, most of them are still in the mire of three limitations: 1) vulnerable to the geometric transformations; 2) incapable of preserving the manifold structure when learning binary codes; 3) NO guarantee to find the true match if multiple candidates happen to have the same Hamming distance to a given query. All these together make the binary descriptor less effective, given large-scale visual recognition tasks. In this paper, we propose a novel learning-based feature descriptor, namely Unsupervised Deep Binary Descriptor (UDBD), which learns transformation invariant binary descriptors via projecting the original data and their transformed sets into a joint binary space. Moreover, we involve a l(2,1)-norm loss term in the binary embedding process to gain simultaneously the robustness against data noises and less probability of mistakenly flipping bits of the binary descriptor, on top of it, a graph constraint is used to preserve the original manifold structure in the binary space. Furthermore, a weak bit mechanism is adopted to find the real match from candidates sharing the same minimum Hamming distance, thus enhancing matching performance. Extensive experimental results on public datasets show the superiority of UDBD in terms of matching and retrieval accuracy over state-of-the-arts.
更多查看译文
关键词
Image hashing, feature matching, local binary descriptor, similarity retrieval, deep learning
AI 理解论文
溯源树
样例
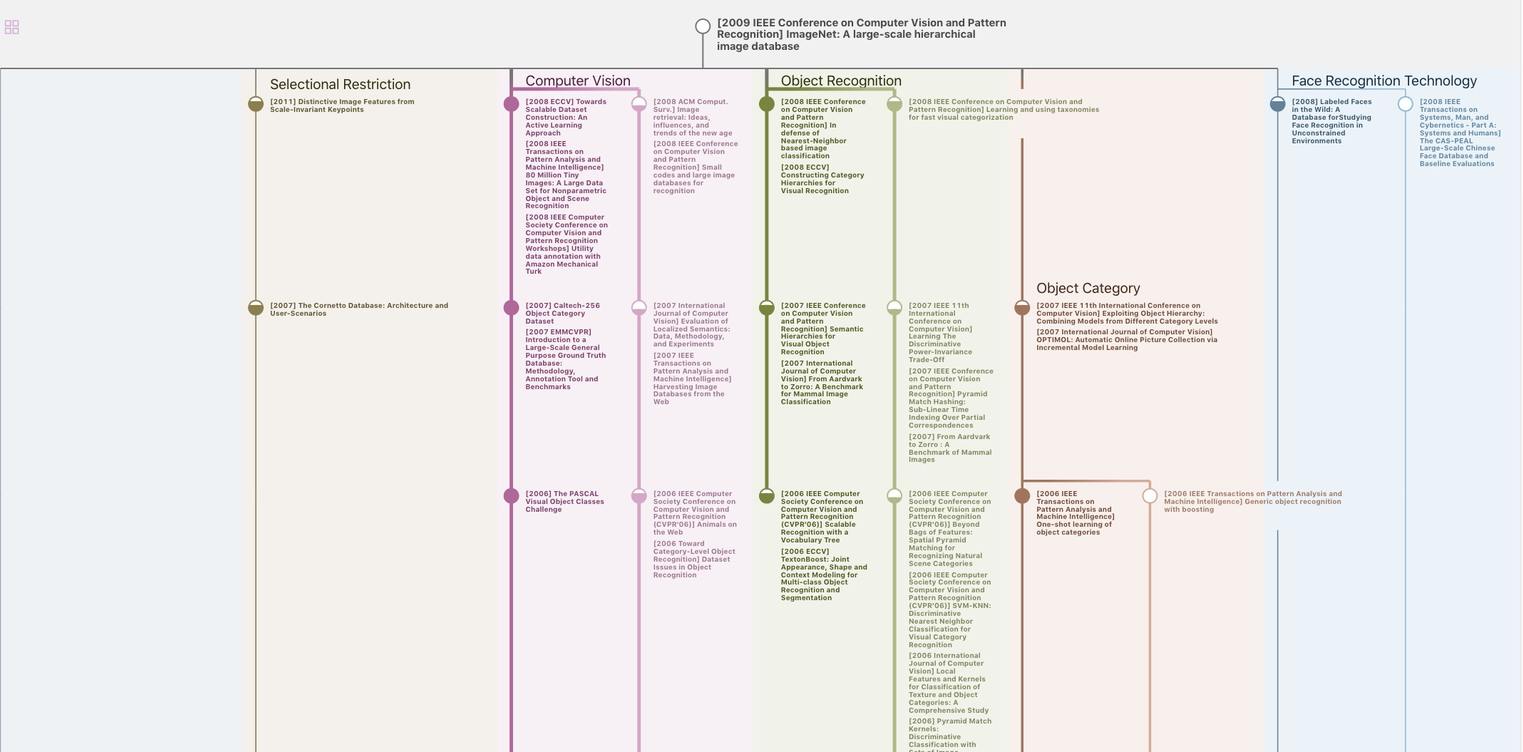
生成溯源树,研究论文发展脉络
Chat Paper
正在生成论文摘要