A Bayesian Hierarchical Framework For Pathway Analysis In Genome-Wide Association Studies
HUMAN HEREDITY(2020)
摘要
Background: Pathway analysis allows joint consideration of multiple SNPs belonging to multiple genes, which in turn belong to a biologically defined pathway. This type of analysis is usually more powerful than single-SNP analyses for detecting joint effects of variants in a pathway. Methods: We develop a Bayesian hierarchical model by fully modeling the 3-level hierarchy, namely, SNP-gene-pathway that is naturally inherent in the structure of the pathways, unlike the currently used ad hoc ways of combining such information. We model the effects at each level conditional on the effects of the levels preceding them within the generalized linear model framework. To deal with the high dimensionality, we regularize the regression coefficients through an appropriate choice of priors. The model is fit using a combination of iteratively weighted least squares and expectation-maximization algorithms to estimate the posterior modes and their standard errors. A normal approximation is used for inference. Results: We conduct simulations to study the proposed method and find that our method has higher power than some standard approaches in several settings for identifying pathways with multiple modest-sized variants. We illustrate the method by analyzing data from two genome-wide association studies on breast and renal cancers. Conclusion: Our method can be helpful in detecting pathway association.
更多查看译文
关键词
Posterior mode, Iteratively weighted least squares, Expectation maximization, Breast cancer, Renal cancer
AI 理解论文
溯源树
样例
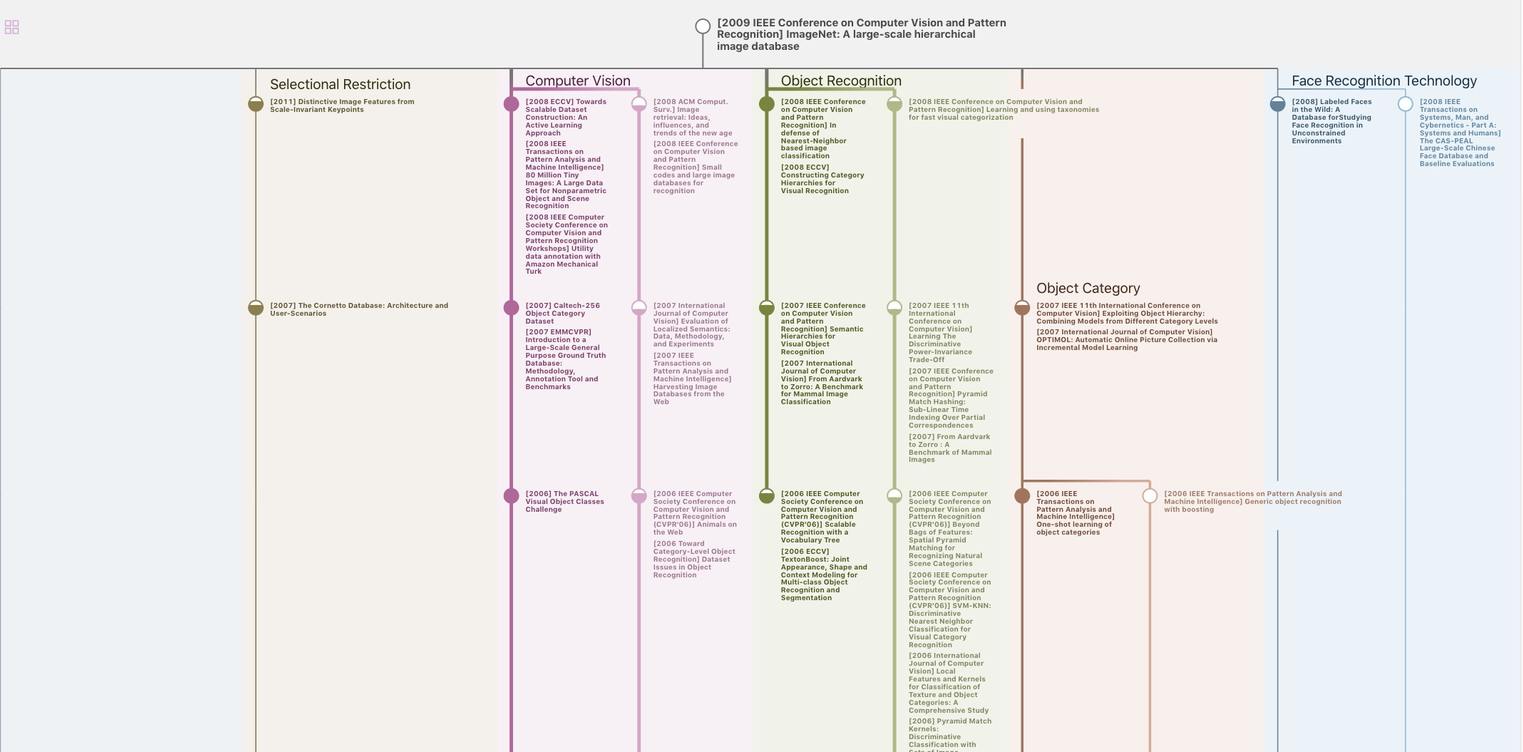
生成溯源树,研究论文发展脉络
Chat Paper
正在生成论文摘要