Temporal Residual Feature Learning for Efficient 3D Convolutional Neural Network on Action Recognition Task
2020 IEEE Workshop on Signal Processing Systems (SiPS)(2020)
摘要
Deep 3-dimensional (3D) Convolutional Neural Network (ConvNet) has shown promising performance on video classification tasks because of its powerful spatio-temporal feature learning ability. However, the extremely intensive requirements of computing power prohibit 3D ConvNet from being used in resource-constrained scenarios. Therefore, it is vital to design efficient architectures. In this paper, we first evaluate the Fully Separated Block (FSB) in 3D ConvNet, which can significantly compress model size. Nevertheless, it also introduces considerable accuracy loss. In order to further improve the performance of efficient 3D ConvNet on action recognition task, a feature enhancement approach named Temporal Residual Module (TRM) is proposed, which can eliminate the loss brought by FSB and achieve even better performance than original baseline with slight computation overhead. Along with TRM, FSB can be viewed as an integrated module termed Temporal Residual Block (TRB) to replace the basic convolutional module in 3D ConvNet, contributing to less model size and better accuracy. Experiments on the popular action recognition dataset report 3.0% accuracy gain with 7.5 χ compression rate over C3D network and 1.0% accuracy gain with 2.3× compression rate over the state-of-the-art SlowFast ConvNet.
更多查看译文
关键词
Transmission line measurements,Three-dimensional displays,Task analysis,Kernel,Solid modeling,Two dimensional displays,Computational modeling
AI 理解论文
溯源树
样例
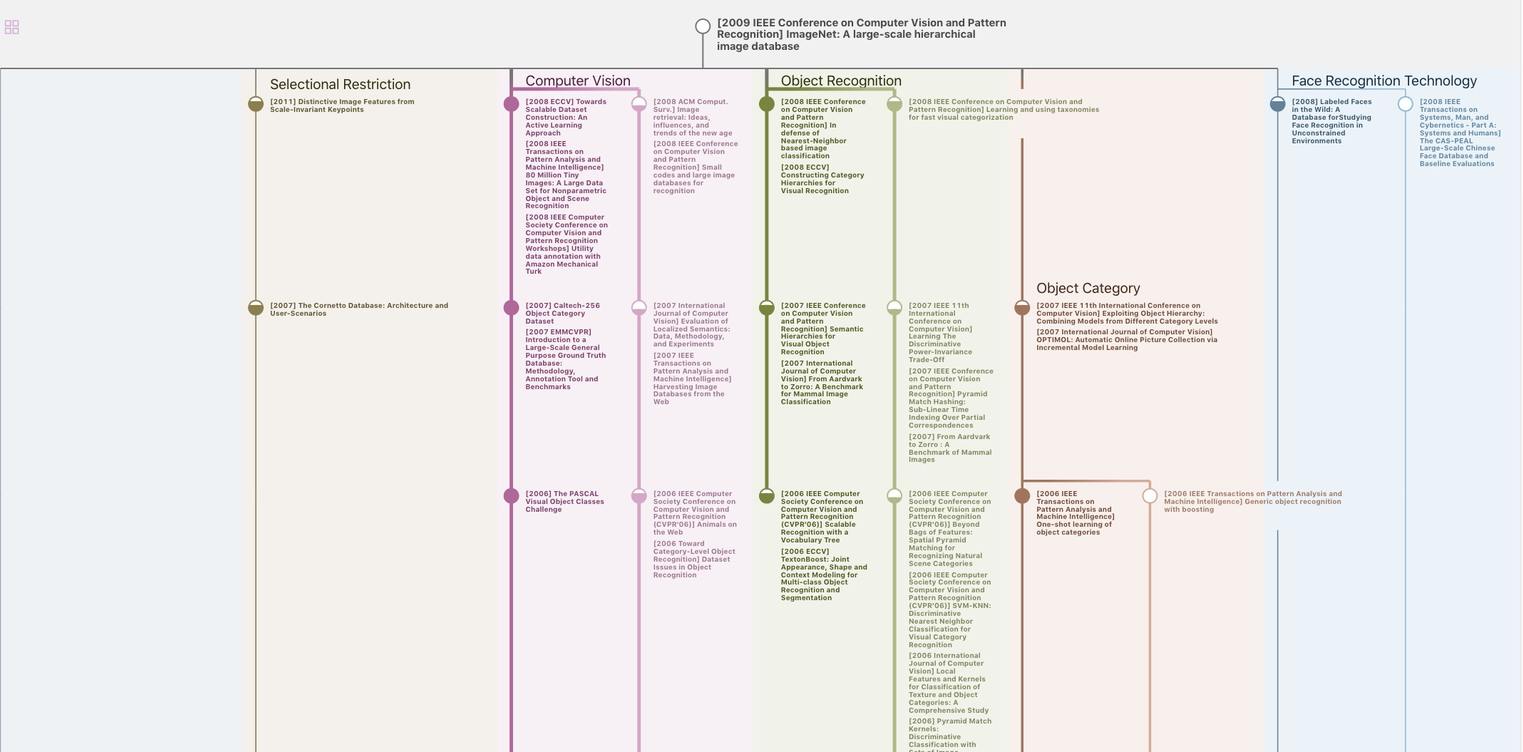
生成溯源树,研究论文发展脉络
Chat Paper
正在生成论文摘要