Crowd Counting via Attention and Multi-Feature Fused Network
HUMAN-CENTRIC COMPUTING AND INFORMATION SCIENCES(2023)
摘要
With the rapid development of Internet of Everything and artificial intelligence techniques and massive amounts of video surveillance data, crowd counting has drawn extensive attention in computer vision. Inspired by deep learning methods, convolutional neural networks (CNN) have been dedicated to improving the effectiveness of crowd counting. As CNN is unable to capture the continuous size changes of heads in images, the large-scale variations impede the development of crowd counting. To solve this problem, this paper presents an attention and multi-feature fused network (AMFNet) containing a multi-level feature extractor and four attentional density estimator (ADE) modules. The multi-level extractor is used to extract the features of different sizes and various kinds of context information based on a deep network backbone. The existing ADE modules are built to merge different level features to generate a high-quality density map. A channel attention unit is adopted in the ADE modules to identify the head accurately. Then, four ADE modules are applied to exploit multi-level features and generate a fine-grained density map for coping with various scales. The experiment results show that the proposed AMFNet performs well in dense crowd scenarios, and that it is comparable to mainstream methods in terms of accuracy and robustness.
更多查看译文
关键词
Crowd Counting,Convolutional Neural Network,Attentional Mechanism,Information Fusion
AI 理解论文
溯源树
样例
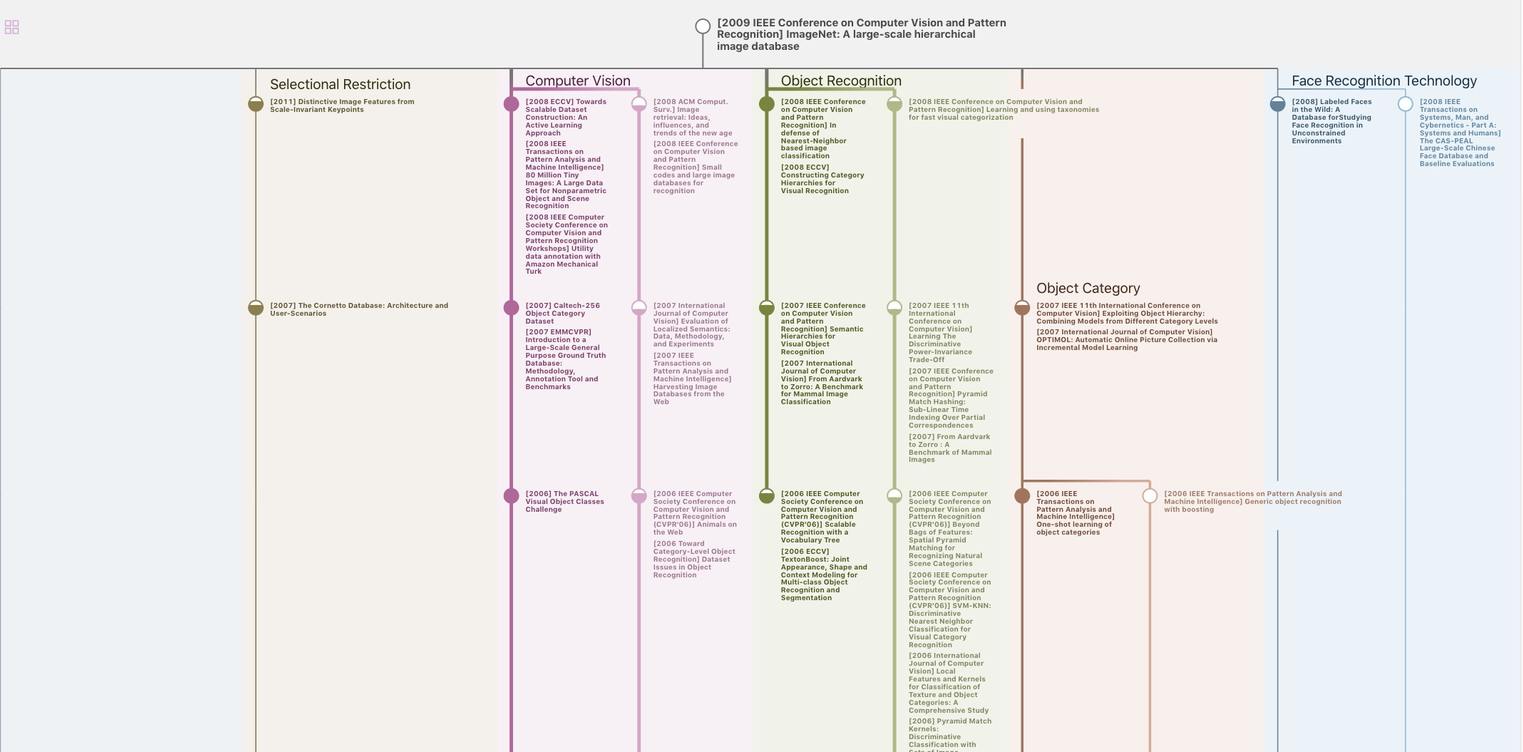
生成溯源树,研究论文发展脉络
Chat Paper
正在生成论文摘要