Proportional stochastic generalized Lotka-Volterra model with an application to learning microbial community structures.
arxiv(2023)
摘要
Inferring microbial community structure based on temporal metagenomics data is an im-portant goal in microbiome studies. The deterministic generalized Lotka-Volterra (GLV) dif-ferential equations have been commonly used to model the dynamics of microbial taxa. However, these approaches fail to take random environmental fluctuations into account and usually ignore the compositional nature of relative abundance data, which may deteri-orate the estimates. In this article, we consider the microbial dynamics in terms of relative abundances by introducing a reference taxon, and propose a new proportional stochas-tic GLV (pSGLV) differential equation model, where the random perturbations of Brownian motion in this model can naturally account for the external environmental effects on the microbial community. We establish conditions and show some mathematical properties of the solutions including general existence and uniqueness, stochastic ultimate bounded-ness, stochastic permanence, the existence of stationary distribution, and ergodicity prop-erty. We further develop approximate maximum likelihood estimators (AMLEs) based on discrete observations and systematically investigate the consistency and asymptotic nor-mality of the proposed estimators. At last, numerical simulations support our theoretical findings and our method is demonstrated through an application to the well-known "mov-ing picture" temporal microbial dataset.(c) 2023 Elsevier Inc. All rights reserved.
更多查看译文
关键词
Interaction network,Maximum likelihood,Relative abundance,Stochastic differential equation
AI 理解论文
溯源树
样例
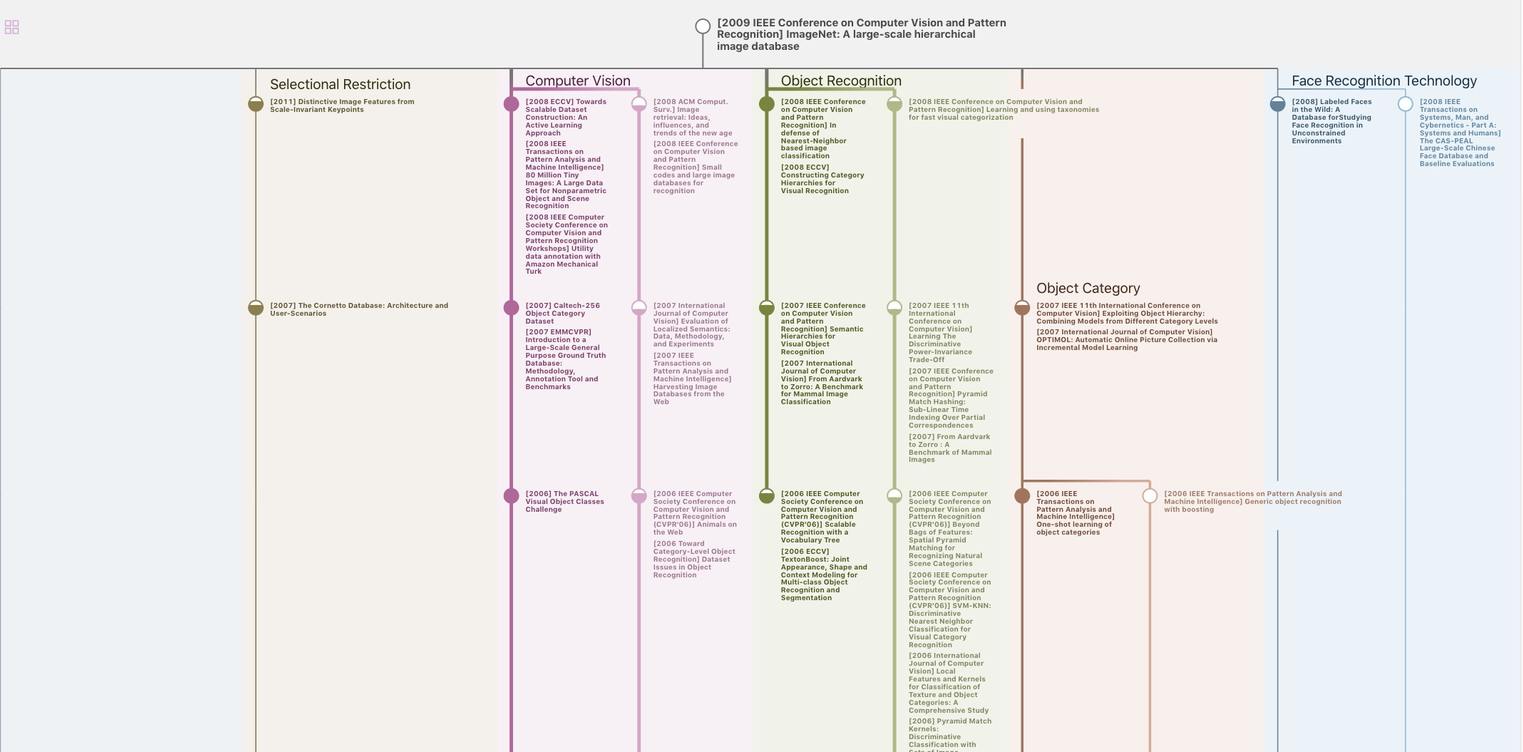
生成溯源树,研究论文发展脉络
Chat Paper
正在生成论文摘要