Deep Reinforcement Learning for On-line Dialogue State Tracking
arxiv(2020)
摘要
Dialogue state tracking (DST) is a crucial module in dialogue management. It is usually cast as a supervised training problem, which is not convenient for on-line optimization. In this paper, a novel companion teaching based deep reinforcement learning (DRL) framework for on-line DST optimization is proposed. To the best of our knowledge, this is the first effort to optimize the DST module within DRL framework for on-line task-oriented spoken dialogue systems. In addition, dialogue policy can be further jointly updated. Experiments show that on-line DST optimization can effectively improve the dialogue manager performance while keeping the flexibility of using predefined policy. Joint training of both DST and policy can further improve the performance.
更多查看译文
关键词
deep reinforcement learning,dialogue,tracking,on-line
AI 理解论文
溯源树
样例
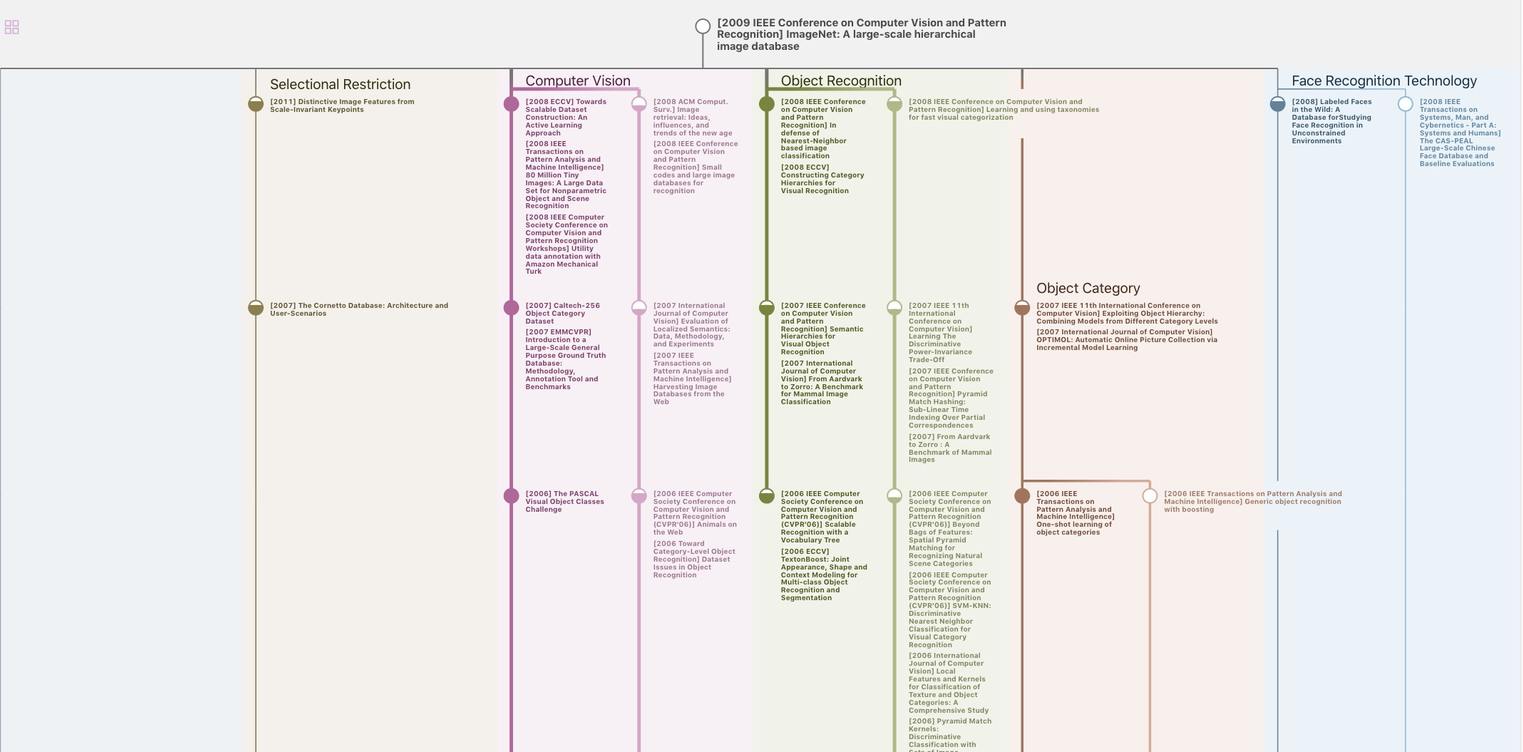
生成溯源树,研究论文发展脉络
Chat Paper
正在生成论文摘要