Anatomy-Regularized Representation Learning for Cross-Modality Medical Image Segmentation
IEEE Transactions on Medical Imaging(2021)
摘要
An increasing number of studies are leveraging unsupervised cross-modality synthesis to mitigate the limited label problem in training medical image segmentation models. They typically transfer ground truth annotations from a label-rich imaging modality to a label-lacking imaging modality, under an assumption that different modalities share the same anatomical structure information. However, since these methods commonly use voxel/pixel-wise cycle-consistency to regularize the mappings between modalities, high-level semantic information is not necessarily preserved. In this paper, we propose a novel anatomy-regularized representation learning approach for segmentation-oriented cross-modality image synthesis. It learns a common feature encoding across different modalities to form a shared latent space, where 1) the input and its synthesis present consistent anatomical structure information, and 2) the transformation between two images in one domain is preserved by their syntheses in another domain. We applied our method to the tasks of cross-modality skull segmentation and cardiac substructure segmentation. Experimental results demonstrate the superiority of our method in comparison with state-of-the-art cross-modality medical image segmentation methods.
更多查看译文
关键词
Heart,Image Processing, Computer-Assisted,Skull
AI 理解论文
溯源树
样例
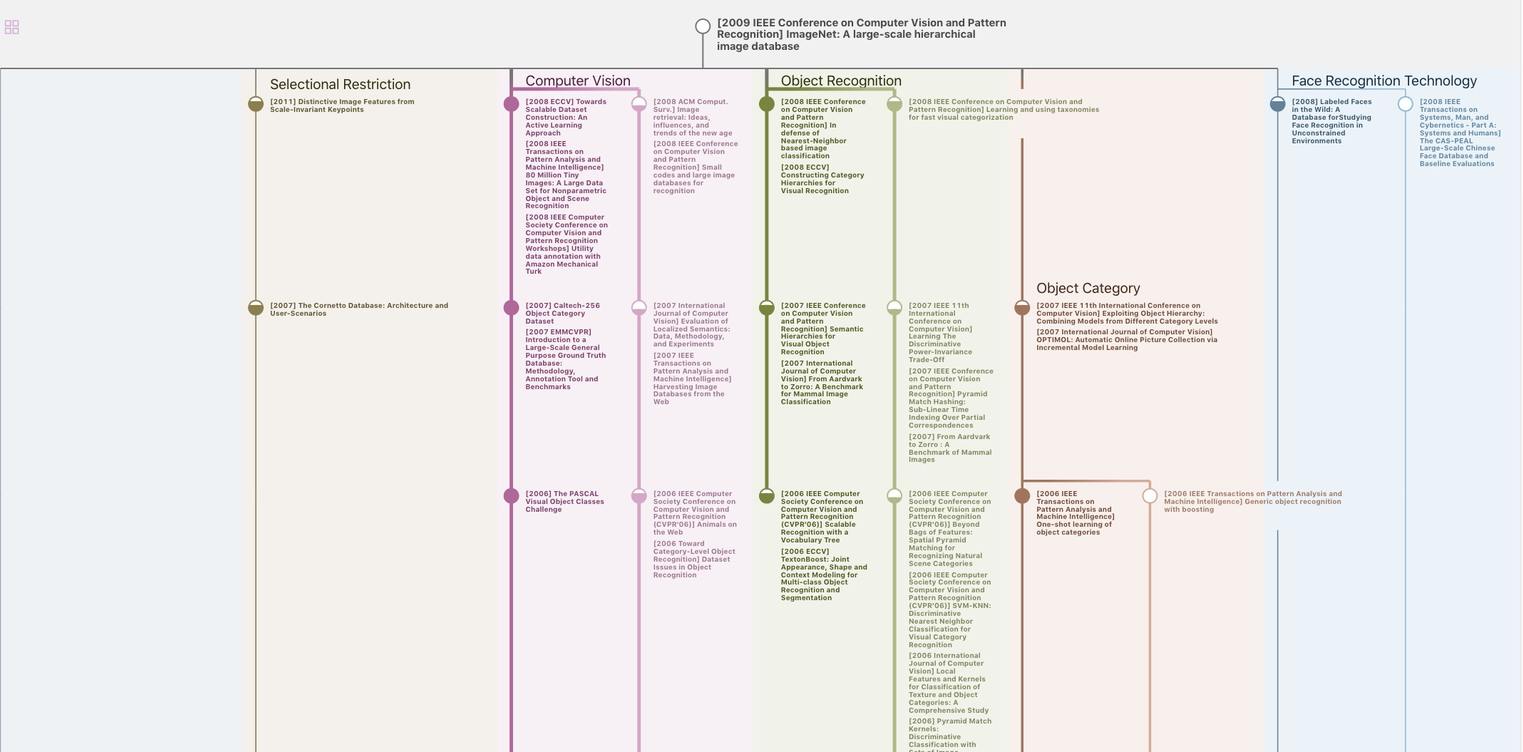
生成溯源树,研究论文发展脉络
Chat Paper
正在生成论文摘要