Learning Clique Subgraphs in Structural Brain Network Classification with Application to Crystallized Cognition
NeuroImage(2021)
摘要
Structural brain networks constructed from diffusion MRI are important biomarkers for understanding human brain structure and its relation to cognitive functioning. There is increasing interest in learning differences in structural brain networks between groups of subjects in neuroimaging studies, leading to a variable selection problem in network classification. Traditional methods often use independent edgewise tests or unstructured generalized linear model (GLM) with regularization on vectorized networks to select edges distinguishing the groups, which ignore the network structure and make the results hard to interpret. In this paper, we develop a symmetric bilinear logistic regression (SBLR) with elastic-net penalty to identify a set of small clique subgraphs in network classification. Clique subgraphs, consisting of all the interconnections among a subset of brain regions, have appealing neurological interpretations as they may correspond to some anatomical circuits in the brain related to the outcome. We apply this method to study differences in the structural connectome between adolescents with high and low crystallized cognitive ability, using the crystallized cognition composite score, picture vocabulary and oral reading recognition tests from NIH Toolbox. A few clique subgraphs containing several small sets of brain regions are identified between different levels of functioning, indicating their importance in crystallized cognition.
更多查看译文
关键词
Network classification,Signal subgraph learning,Clique subgraphs,Structural brain networks,Symmetric bilinear logistic regression
AI 理解论文
溯源树
样例
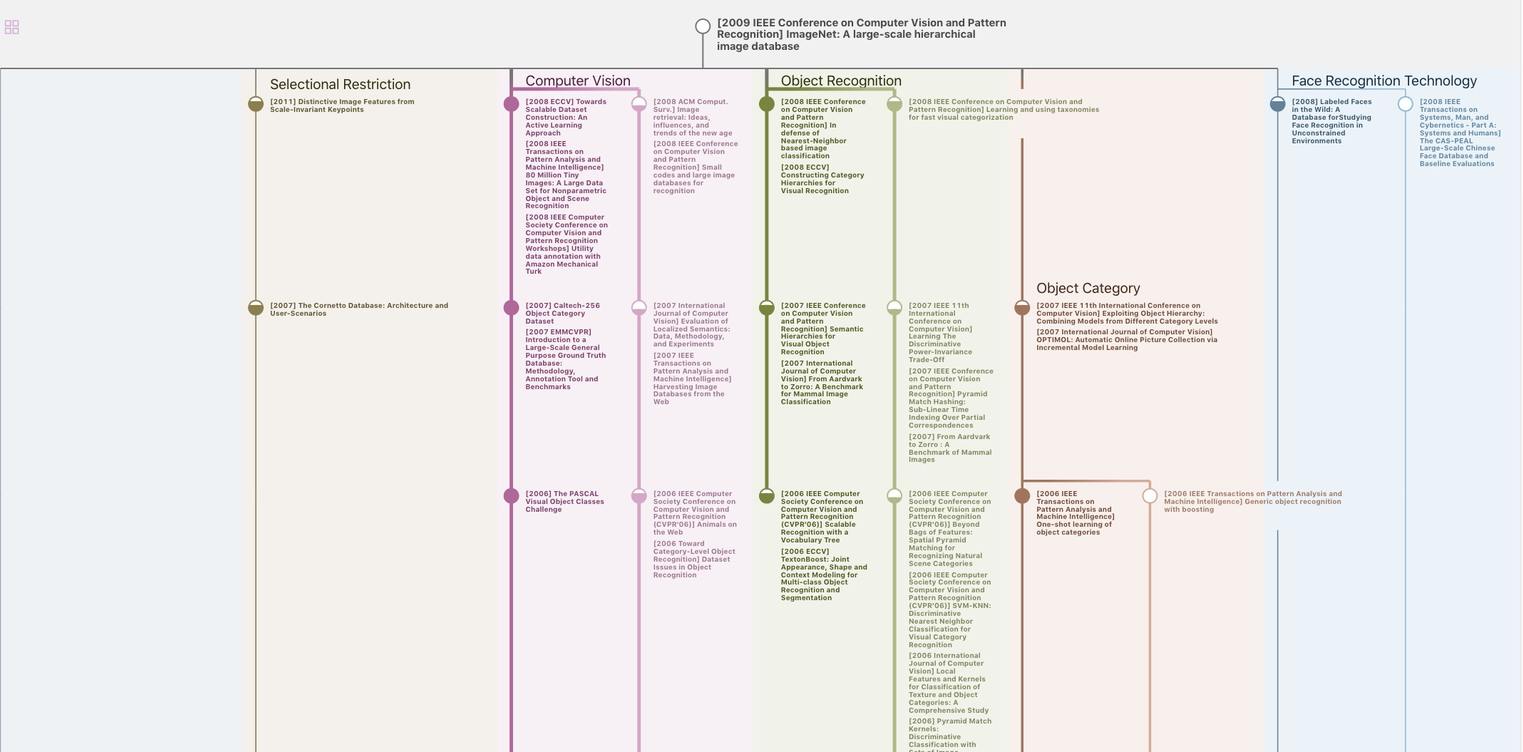
生成溯源树,研究论文发展脉络
Chat Paper
正在生成论文摘要